人脸识别的简单算法.docx
《人脸识别的简单算法.docx》由会员分享,可在线阅读,更多相关《人脸识别的简单算法.docx(14页珍藏版)》请在冰豆网上搜索。
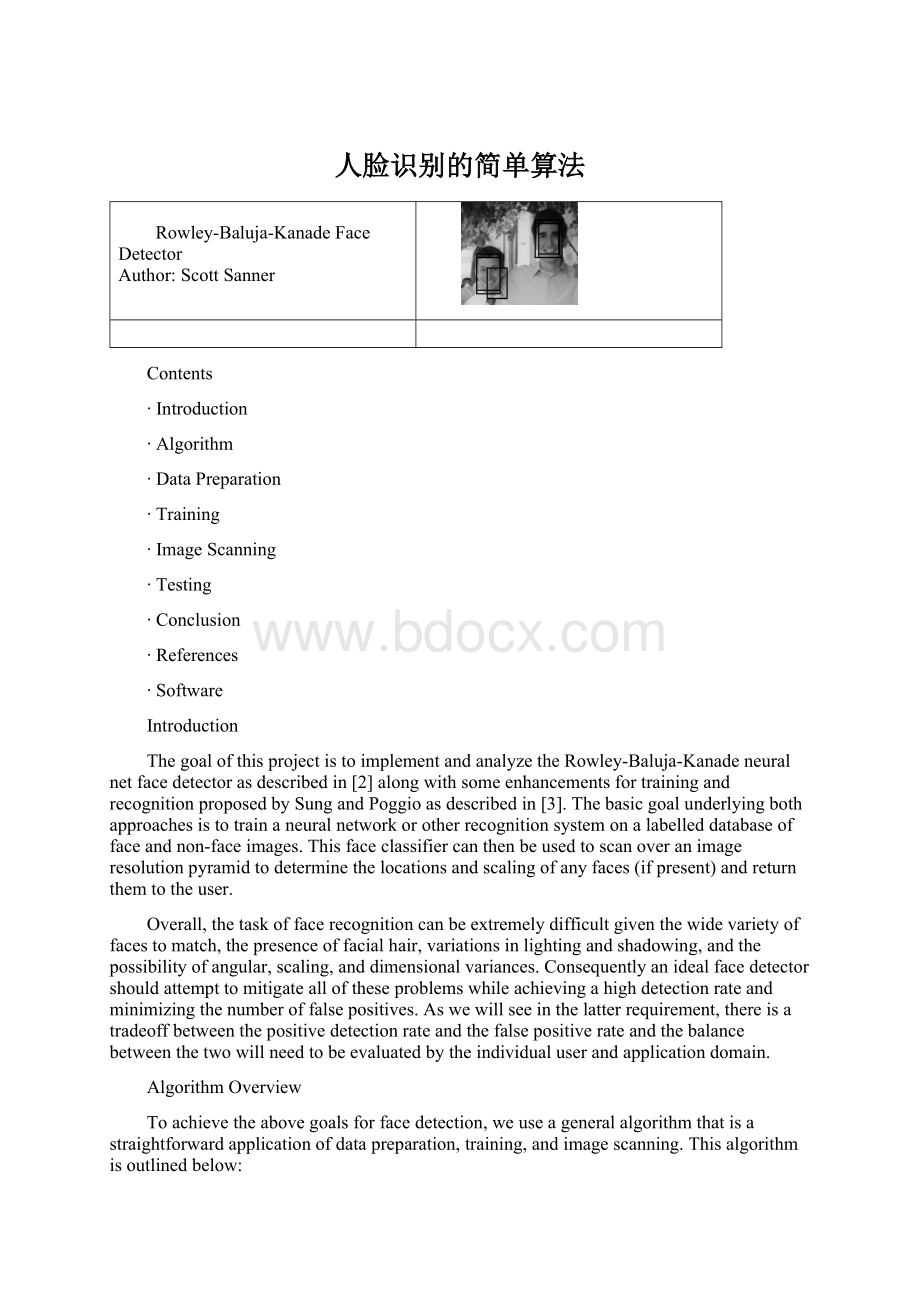
人脸识别的简单算法
Rowley-Baluja-KanadeFaceDetector
Author:
ScottSanner
Contents
∙Introduction
∙Algorithm
∙DataPreparation
∙Training
∙ImageScanning
∙Testing
∙Conclusion
∙References
∙Software
Introduction
ThegoalofthisprojectistoimplementandanalyzetheRowley-Baluja-Kanadeneuralnetfacedetectorasdescribedin[2]alongwithsomeenhancementsfortrainingandrecognitionproposedbySungandPoggioasdescribedin[3].Thebasicgoalunderlyingbothapproachesistotrainaneuralnetworkorotherrecognitionsystemonalabelleddatabaseoffaceandnon-faceimages.Thisfaceclassifiercanthenbeusedtoscanoveranimageresolutionpyramidtodeterminethelocationsandscalingofanyfaces(ifpresent)andreturnthemtotheuser.
Overall,thetaskoffacerecognitioncanbeextremelydifficultgiventhewidevarietyoffacestomatch,thepresenceoffacialhair,variationsinlightingandshadowing,andthepossibilityofangular,scaling,anddimensionalvariances.Consequentlyanidealfacedetectorshouldattempttomitigatealloftheseproblemswhileachievingahighdetectionrateandminimizingthenumberoffalsepositives.Aswewillseeinthelatterrequirement,thereisatradeoffbetweenthepositivedetectionrateandthefalsepositiverateandthebalancebetweenthetwowillneedtobeevaluatedbytheindividualuserandapplicationdomain.
AlgorithmOverview
Toachievetheabovegoalsforfacedetection,weuseageneralalgorithmthatisastraightforwardapplicationofdatapreparation,training,andimagescanning.Thisalgorithmisoutlinedbelow:
NormalizeTrainingData:
-Foreachfaceandnon-faceimage:
-Subtractoutanapproximationoftheshadingplane
tocorrectforsinglelightsourceeffects
-Rescalehistogramsothateveryimagehasthesame
samegraylevelrange
-Aggregatedataintolabeleddatasets
TrainNeuralNet:
-UntiltheNeuralNetreachesconvergence(oradecrease
inperformanceonthevalidationset):
-Performgradientdescenterrorbackpropagationon
ontheneuralnetforthebatchofalltrainingdata
ApplyFaceDetectortoImage:
-Buildaresolutionpyramidoftheimagebysuccessively
successivelydecreasingtheimageresolutionateach
levelofthepyramid,stoppingatsomedefaultminimum
resolution
-Foreachlevelofthepyramid
-Scanovertheimage,applyingthetrainedneuralnet
facedetectortoeachrectanglewithintheimage
-Ifapositivefaceclassificationisfoundfora
rectangle,scalethisrectangletothesize
appropriatefortheoriginalimageandadditto
thefacebounding-boxset
-Returntherectanglesinthefacebounding-boxset
DataPreparation
Inperformingfacedetectionwithaneuralnet,afewface-specificandnon-face-specificissuesarise.
Intherealmoffacespecificissues,wedonotwantthebackgroundtobecomeinvolvedinfacematching.Consequently,ifpersonAisintwodifferentsettingswewanttoensurethatweperformaswellaspossibleindetectingpersonA'sfacedespitethebackgroundvariation.Ifwewereonlytolookatpotentialcandidaterectanglesforafacethenwewouldreceiveinterferencefromthecornerswhicharemorelikelytoconsistofbackgroundthanfacepixels.Neuralnetsareespeciallysusceptibletosucherrorssinceanyconsistenciesbetweendatainthetrainingset(nomatterhowplausibleapredictorofface-hoodinreallife)willlikelybedetectedandexploited.Thus,as[3]suggests,itisagoodideatomaskanovalwithinthefacerectangletoprunethepixelsusedintraininginneuralnet.Fortruefaceimages,thisusuallyguaranteesthatonlypixelsfromthefaceareusedasinputtotheneuralnet.Forourimplementation,weusetheovalmaskwhichcanbeseeninfigure3.Theboundingrectangleforthismaskis18x27pixels.
Anotherfacespecificissueisthatofposeorglasses.Wewanttorecognizeafaceinvariantofwhetherapersonissmiling,sad,wearingglasses,ornotwearingglasses.Consequentlyitisimportanttoconstructasetoftrainingdatawhichcoversabroadrangeofhumanemotions,poses,andglasses/non-glasseswearingfaces.Thisensuresthegreatestgeneralizationwhenapplyingthefacedetectortofaceswhichhavenotbeenseenbefore.Forourdataset,weuse30facesandtheirleft-rightflippedversionswithavarietyofemotionsandposesascontainedintheYaleFaceDatabase[1].Itwouldbeadvantageoustohavemorefacesandposesthanthisbutthetimelimitsofthisprojectconstrainedtheamountoftimethatcouldbedevotedtophotoediting(sincetheYaleFaceDatabaseisnotinadirectlyusableformat).
Onenon-facespecificissueisthatoflightingdirection.Neuralnetsareespeciallysusceptibletopixelmagnitudevaluesandthedifferencesbetweenimagesilluminatedfromtheleftorrightmaybeenoughtomakethemappearastwodifferentclassificationsfromtheperspectiveoftheneuralnet.Consequently,therehastobesomemethodforcorrectingforunidirectionallightingeffects(evenifonlyapproximate).Additionally,notallimageswillhavethesamegrayleveldistributionorrangeanditisimportanttomitigatethisasmuchaspossibletoavoidbiaseffectsduetograyleveldistribution.
Forourdataset,weattempttocorrectforunidirectionallightingeffectsassuggestedby[2]byfittingasinglelinearplanetotheimage.Thisplanecanbecomputedefficientlythroughsimplelinearprojectionsolvingtheequation[XY1]*C=Z(whereX,Y,andZarethevectorscorrespondingtotheirrespectivecoordinatevalues,1isavectorof1'stocomputetheconstantoffset,andCisavectorofthreenumbersdefiningthelinearslopesintheXandYdirectionsandtheconstantoffset).TocomputeC,wesimplyneedtocompute([XYO]'*[XYO])^-1*[XYO]'*Z.TheseplanecoefficientsinCapproximatetheaveragegraylevelacrosstheimageunderalinearconstraintandthuscanbeusedtoconstructashadingplanethatcanbesubtractedoutoftheoriginalimage.Oncethelightingdirectioniscorrectedfor,thegrayscalehistogramcanthenberescaledtospantheminandmaximumgrayscalelevelsallowedbytherepresentation.
Thiswasdoneforourface(andnon-face)trainingdataandanoriginalsubsetofimagesareshowninfigure1below:
Figure1:
InitialImages.
Fromfigure1,wethenapproximatetheshadingplaneasshownbelow.Notethatthesecondandthirdimagesinfigure1showheavydirectionallightingeffectsandthattheshadingplaneinfigure2accuratelyrepresentstheseeffects.
Figure2:
ShadingApproximations.
Now,giventheimagesinfigures1and2,wecansubtractfigure2fromfigure1andrescalethegraylevelstotheminimumandmaximumrangeforourrepresentation.Wecanthenapplyamasktothisimagetoremovebackgroundinterference.Thisresultisshownbelowinfigure3.
Noteinthefollowingfigurethattheunidirectionallightingeffectspresentintheoriginalsecondandthirdimages(figure1)havenowbeenremovedandthatunlikefigure1,allimagesinfigure3haveapproximatelythesamegrayleveldistribution.Thisnormalizationisextremelyimportanttoproperfunctioningoftheneuralnetwork.
Figure3:
NormalizedandMaskedImages.
Inadditiontothefaceimages,wealsoperformthesamenormalizationonasetofnon-facesceneryimages.Sincewenormalizeallimagesduringthefacedetectionscanningprocess,itisimportanttotrainonnormalizedsceneryimagessincetheunnormalizedsetwouldbeunrepresentativeofthoseseenduringtraining.Asetoffiveofthe160sceneryimagesisshownbelowinfigure4.(Actuallyonly40sceneryimageswereused,buttheirleft-rightandupside-downversionswerealsoaddedtothedataset.)
Figure4:
Non-faceImageExamples.
Onceallofthetrainingdataimageshavebeennormalizedtheyareaggregatedintolabelleddatasetsandpassedontothetrainingphase.Additionally,thenormalizationprocessoccursoncemoreduringtheactualfacedetectionprocess,i.e.allimagesrectanglesarenormalizedbeforeclassifyingthemwiththeneuralnet.
Training
Givenourmasksize,weuseaneuralnet(createdandtrainedusingMatlab'sneuralnettoolbox)withapproximately400inputunitsconnecteddirectlytoacorrespondingpixelwithintheimagemask,20hiddenunits,and1outputunitusedforprediction(yieldingidealtrainingvaluesof-0.9forsceneryand0.9foraface).
Theneuralnetistrainedfor500epochs(oruntilerrorincreasesonanindependentvalidationchosenseparatelyfromthetrainingset).Thesumofsquareserrorrateonthetrainingset(blue)andthevalidationset(red)areplottedbelowinFigure5.Notethataroundepoch50,thevalidationseterrorsurpassesthetrainingseterror(aswouldbeexpected).Howeverthevalidationseterrorneverincreasesfromaprevioustimestepandthereforethenetworkprocedestoapproximateconvergence.Thisindicatesthatinsomesensethetrainingsetisadequateenoughtogeneralizetounseeninstances.
Figure5:
TrainingErrorvs.Epochs.
Thefinalperformanceofthenetworkonallofthefaceandnon-facedataisshownbelowintable1.Thenetworkapparentlyperformsmuchbetteratdetectingnon-faceswhichisprobablyduetothebiastowardnon-facetrainingimagesinthedataset.However,thishastheadvantageofyieldingalowerfalsepositiveratethanifthebiashadbeeninfavorofthefaceimagesinstead.
FaceDetectionRate
Non-faceDetectionRate
OverallClassifcationRate
PercentageCorrect
86.7%
98.1%
97.7%
TrainingSetSize
60
160
220
Table1:
TrainingResults.
Nowthattheneuralnethasbeensuccessfullytrained,itcannowbeusedforclassifyingcandidatefacerectanglespasse