斯坦福大学机器学习第七讲Lecture7.docx
《斯坦福大学机器学习第七讲Lecture7.docx》由会员分享,可在线阅读,更多相关《斯坦福大学机器学习第七讲Lecture7.docx(11页珍藏版)》请在冰豆网上搜索。
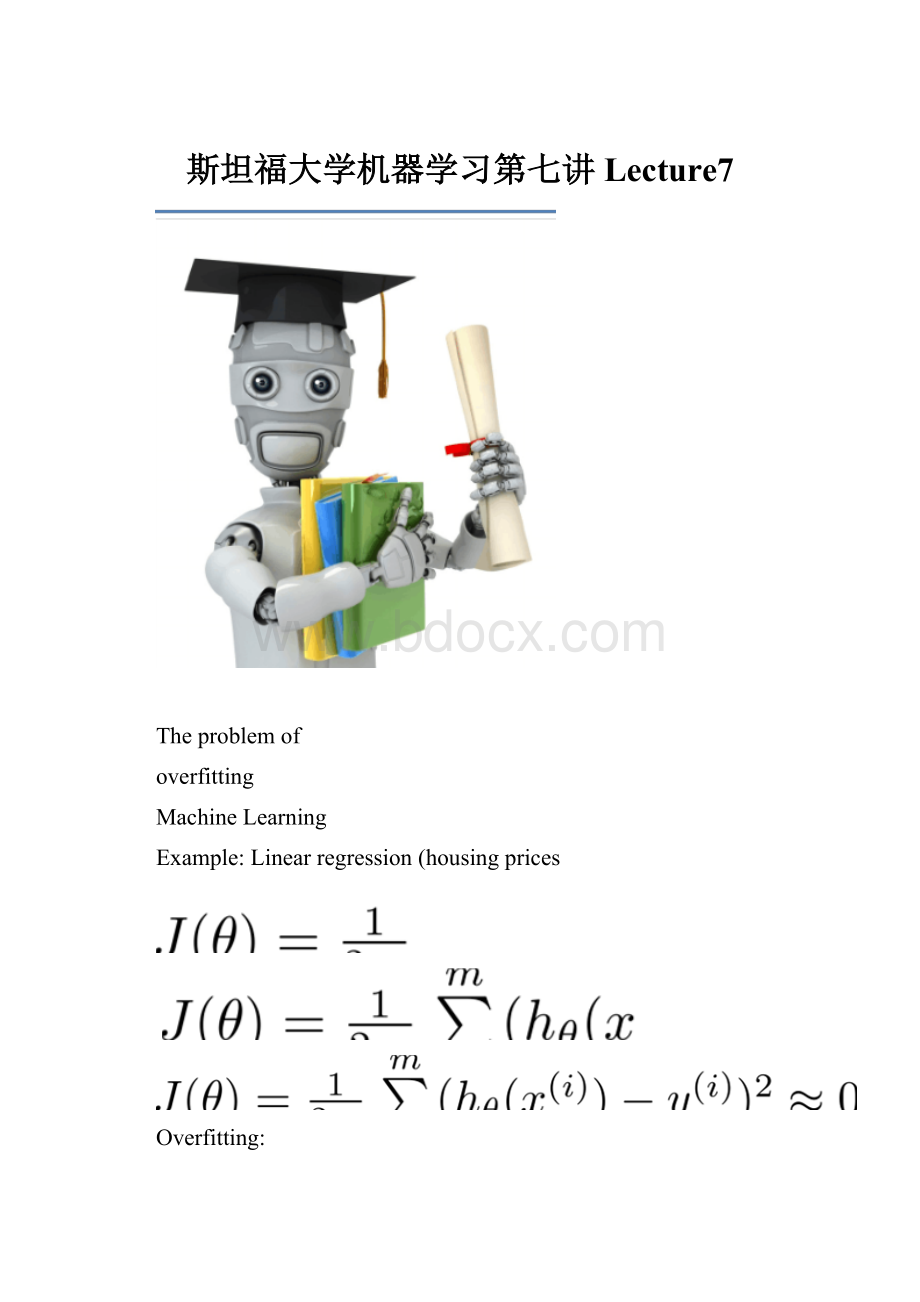
斯坦福大学机器学习第七讲Lecture7
Theproblemof
overfitting
MachineLearning
Example:
Linearregression(housingprices
Overfitting:
Ifwehavetoomanyfeatures,thelearnedhypothesistogeneralizetonewexamples(predictpricesonnewexamples.
Price
SizePrice
SizePrice
Size
Example:
Logisticregression
(=sigmoidfunction
x
1
x
2
x
1
x
2
x
1
x
2
P
r
i
c
e
Sizesizeofhouse
no.ofbedrooms
no.offloors
ageofhouse
averageincomeinneighborhood
kitchensize
Options:
1.Reducenumberoffeatures.
―Manuallyselectwhichfeaturestokeep.
―Modelselectionalgorithm(laterincourse.
2.Regularization.
―Keepallthefeatures,butreducemagnitude/valuesofparameters.
―Workswellwhenwehavealotoffeatures,eachofwhichcontributesabittopredicting.
Costfunction
MachineLearning
Intuition
Supposewepenalizeandmake,reallysmall.
PriceSizeofhousePrice
Sizeofhouse
Smallvaluesforparameters
―“Simpler”hypothesis
―LesspronetooverfittingHousing:
―Features:
―Parameters:
P
r
ic
eSizeo
fhouse
Whatifissettoanextremelylargevalue(perhapsfortoolargeforourproblem,say?
-Algorithmworksfine;settingtobeverylargecan’thurtit
-Algortihmfailstoeliminateoverfitting.
-Algorithmresultsinunderfitting.(Failstofiteventrainingdatawell.
-Gradientdescentwillfailtoconverge.
Whatifissettoanextremelylargevalue(perhapsfortoolargeforourproblem,say?
Price
Sizeofhouse
Regularizedlinear
regression
MachineLearning
Regularizedlinearregression
Gradientdescent
Repeat
Normalequation
Suppose,
Non-invertibility
(optional/advanced.(#examples(#featuresIf,
Regularized
logisticregression
MachineLearning
Regularizedlogisticregression.x2x1Costfunction:
AndrewNg
GradientdescentRepeatAndrewNg
Advancedoptimizationfunction[jVal,gradient]=costFunction(thetajVal=[codetocompute];];gradient(1=[codetocomputegradient(2=[codetocompute];gradient(3=[codetocompute];gradient(n+1=[codetocompute];AndrewNg