深度学习的亚健康识别算法的研究.docx
《深度学习的亚健康识别算法的研究.docx》由会员分享,可在线阅读,更多相关《深度学习的亚健康识别算法的研究.docx(76页珍藏版)》请在冰豆网上搜索。
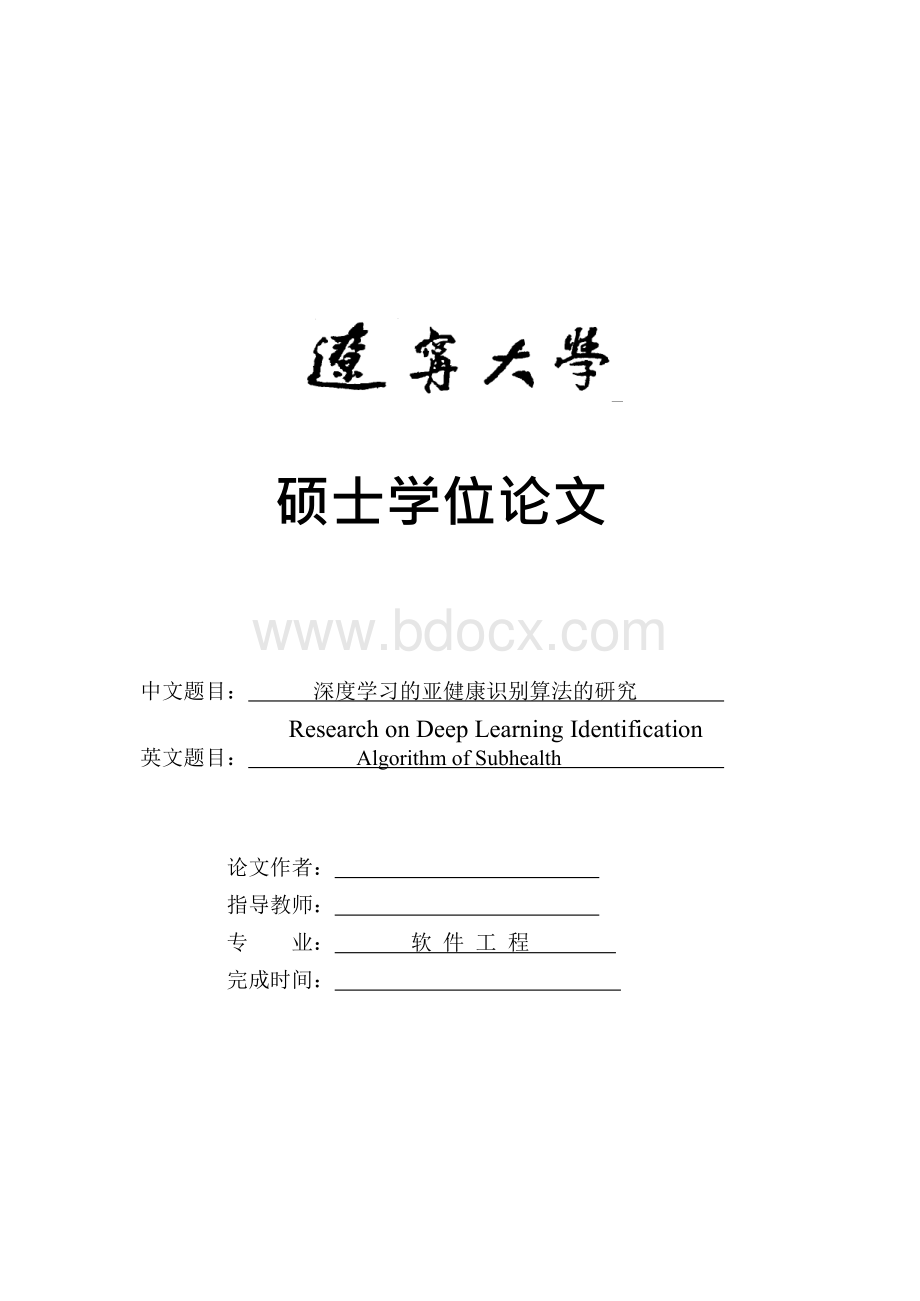
硕士学位论文
中文题目:
深度学习的亚健康识别算法的研究
ResearchonDeepLearningIdentification
英文题目:
AlgorithmofSubhealth
论文作者:
指导教师:
专 业:
软件工程 完成时间:
申请辽宁大学硕士学位论文
深度学习的亚健康识别算法的研究
ResearchonDeepLearningIdentificationAlgorithmofSubhealth
作 者:
指导教师:
专 业:
软件工程
答辩日期:
摘要
摘 要
在工业生产过程中,由于复杂的工作环境和生产流程,故障时有发生,而故障状态的发生并不是瞬时完成的,从正常状态到故障状态的转化是一个积累的过程,而如果能提前预测故障的发生并进行及时的调控,对于工业生产的顺利进行和经济效益的最大化尤为重要,因此研究故障的发生和预测成为故障诊断领域的重点,并引起了很多专家学者的关注。
在阅读分析了大量的深度学习和故障诊断方法后,针对传统的故障诊断特征提取困难,诊断效果不理想,本文提出了一种基于改进深度学习的故障诊断方法。
本文采用层叠降噪自动编码机作为深度学习的网络结构,通过降噪编码机可以对机械振动信号中大量的噪声进行过滤,有助于噪声特征的提取。
而深度学习中的非监督预训练方法可以自动的提取出数据中的深层特征,简化传统人工提取的过程。
针对深度学习过程中由于层数加深导致梯度消失的问题,本文对层叠降噪自动编码机的结构进行改进,将浅层节点的表达和深层节点的表达进行融合,避免了深层网络表达能力不如浅层网络的缺点,最后使用支持向量机对提取出的深度特征进行分类处理。
针对机械设备从“正常”状态到“故障”状态分类的局限性,本文通过引入可靠性理论中的威布尔分布对滚动轴承的“亚健康”状态进行划分。
由于同一种轴承在同样工况下寿命分布不均匀,所以不仅需要对轴承进行整体预测,也需要对轴承在线状态进行检测,本文利用深度学习的方法提出了一种轴承不同时期状态划分的方法,对时间序列进行处理,将其应用于滚动轴承寿命预测。
针对不同的滚动轴承运行状态本文提出了一种基于统计概率时间序列处理方法,通过对滚动承状态时间序列的预测可以有效的对当前轴承进行使用寿命的预测。
通过实验台数据对本文的方法进行验证,从实验对比结果表明,改进的层叠降噪自动编码器对滚动轴承故障诊断具有良好的表现,对不同滚动轴承不同工况下的“亚健康”状态诊断也取得了很好的效果。
关键词:
深度学习,层叠降噪自动编码,健康度,“亚健康
I
Abstract
Abstract
Intheindustrialproductionprocess,afaultwilloccurrduetothecomplexityoftheworkenvironmentandproductionprocess,butthefaultnotoccurinstantaneously,fromanormaltoafaultconditionisacumulativeprocess,itisimportanttotheindustrialproductionandthesmoothprogressofeconomicandbenefitmaximizationifwecanpredictthefaultinadvanceandintimelyregulation.Therefore,theresearchoffaultoccurrenceandpredictionbecomesafocusinthefieldoffaultdiagnosis,andcausedtheattentionofmanyexpertsandscholars.
Afterreadingalargenumberofdeeplearningandmethodsoffaultdiagnosis,thetraditionalfaultdiagnosisfeatureextractiondifficultdiagnosisresultisnotsatisfactory,soweproposeamethodoffaultdiagnosisbasedonimproveddeeplearning.Inthispaper,thestackeddenoisingautoencoderwhichcanbefilteredthenoiseofalargenumberofmechanicalvibrationsignalsisusedfordeeplearningstructuretoextractthecharacteristicsofthenoise.Thedepthofthedatacanbeextractedautomaticallybytheunsupervisedpre-trainmethod,whichgreatlysimplifiestheprocessoftraditionalmanualextraction.Inthispaper,amethodisproposedtoimprovethestackeddenoisingautoencodertogetrideofthethegradientgoawaywithdeeperlayers.Toavoidthedeepnetworkexpressionabilitythanshallownetworkfaults,combinetheshallowanddeepnodesisproposedandfinallytheuseofsupportvectormachinetoextractthedepthfeatureforclassification.
Inthispaper,formachineryandequipmentfromthe"normal"statetothe"failure"stateclassificationlimitations,introducethereliabilitytheoryofWeibullDistributiontodivisionofthesubhealthstateofrollingbearing.Duetothesamebearingunderthesameoperatinglifetimedistributionisnotuniform,soit’snotonlyneedtotheoverallpredictionofbearing,butalsoneedtotesttheonlinestatusofbearing,thedeeplearningofthemethodisproposedtodivisonabearingdifferentperiodstatedivisionmethod,aftersmoothingoftimeseries,itisusedinrollingbearinglifeprediction.Inviewofthedifferentrunningstateofrollingbearings,this
II
paperputforwardabasedonstatisticalprobabilityonlinepredictionmethod,therollingbearingconditiontimeseriespredictioncanbeeffectivetothebearingoftheservicelifeprediction.
Throughtheexperimentaldataofthismethodverifyfromthecomparisonoftheexperimentalresultsshowthat,improvedstackeddenoisingautoencoderforrollingbearingfaultdiagnosishasgoodperformanceanddifferentrollingbearingsunderdifferentworkingconditionsofthesubhealthstatediagnosisalsoachievedgoodresults.
Keywords:
DeepLearning,ImprovedStackDenoisingAutoencoder,HealthDegree,Subhealth
VI
目录
目 录
第1章绪论 1
1.1研究背景和研究意义 1
1.2国内外研究现状 3
1.2.1滚动轴承故障诊断的研究现状 3
1.2.2“亚健康”理论的研究现状及发展 5
1.3论文的主要研究工作 6
1.4论文的结构安排 7
第2章机器学习基础理论 8
2.1BP神经网络 8
2.1.1BP神经网络结构 8
2.1.2BP神经网络的学习步骤 9
2.1.3BP神经网络的优缺点 11
2.2深度学习 11
2.2.1深度学习的基本思想 12
2.2.2深度学习常用方法 14
2.2.3深度学习优点 15
2.3支持向量机 16
2.3.1最优分类面 17
2.3.2广义最优分类面 18
2.3.3支持向量回归机 19
2.3.4核函数 21
2.3.5支持向量机优点 22
2.4本章小结 22
第3章基于深度学习的故障诊断的研究 23
3.1自动编码器原理 23
3.1.1目标函数及推导 24
3.1.2运行步骤 26
3.2层叠降噪自动编码 26
3.3改进层叠降噪自动编码器 28
3.3.1对隐藏层的改进 28
3.3.2对结构的改进 29
3.3.3改进SDA-SVM训练流程 29
3.4实验结果及分析 32
3.4.1轴承数据预处理 33
3.4.2参数确定 35
3.4.3隐层特征的提取 36
3.4.4实验结果分析 40
3.5本章小结 40
第4章滚动轴承的“亚健康”识别 42
4.1“亚健康”状态 42
4.1.1健康度的定义 42
4.1.2亚健康状态的划分 43
4.2“亚健康”识别方法的提出 46
4.2.1已有剩余寿命预测方法 46
4.2.2提出“亚健康”识别方法 48
4.2.3预测剩