4 Lowlevel and highlevel prior learning for visual saliency estimation 2.docx
《4 Lowlevel and highlevel prior learning for visual saliency estimation 2.docx》由会员分享,可在线阅读,更多相关《4 Lowlevel and highlevel prior learning for visual saliency estimation 2.docx(37页珍藏版)》请在冰豆网上搜索。
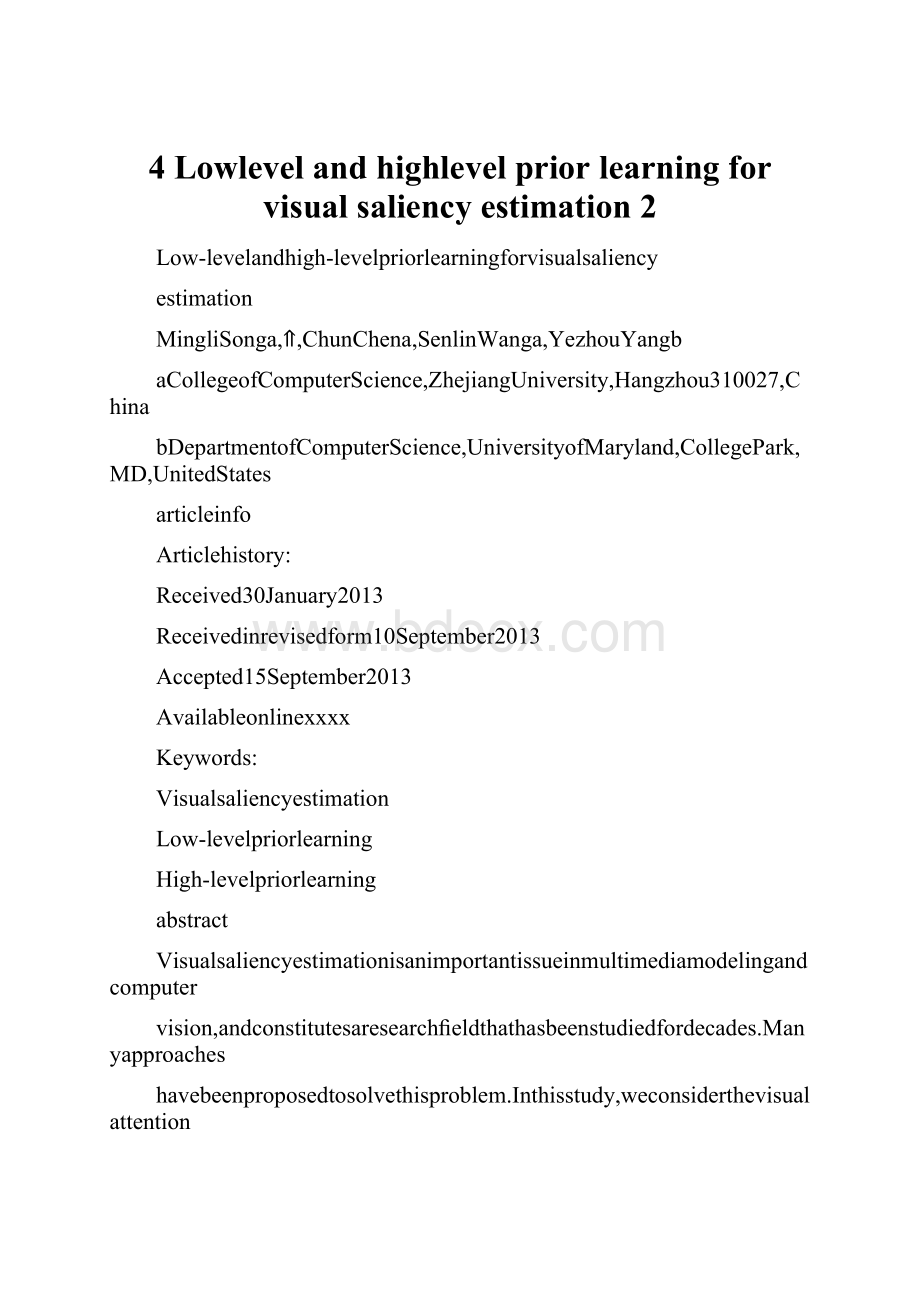
4Lowlevelandhighlevelpriorlearningforvisualsaliencyestimation2
Low-levelandhigh-levelpriorlearningforvisualsaliency
estimation
MingliSonga,⇑,ChunChena,SenlinWanga,YezhouYangb
aCollegeofComputerScience,ZhejiangUniversity,Hangzhou310027,China
bDepartmentofComputerScience,UniversityofMaryland,CollegePark,MD,UnitedStates
articleinfo
Articlehistory:
Received30January2013
Receivedinrevisedform10September2013
Accepted15September2013
Availableonlinexxxx
Keywords:
Visualsaliencyestimation
Low-levelpriorlearning
High-levelpriorlearning
abstract
Visualsaliencyestimationisanimportantissueinmultimediamodelingandcomputer
vision,andconstitutesaresearchfieldthathasbeenstudiedfordecades.Manyapproaches
havebeenproposedtosolvethisproblem.Inthisstudy,weconsiderthevisualattention
problembluewithrespecttotwoaspects:
low-levelpriorlearningandhigh-levelprior
learning.Ontheonehand,inspiredbytheconceptofchanceofhappening,thelow-level
priors,i.e.,ColorStatistics-basedPriors(CSP)andSpatialCorrelation-basedPriors(SCP),
arelearnedtodescribethecolordistributionandcontrastdistributioninnaturalimages.
Ontheotherhand,thehigh-levelpriors,i.e.,therelativerelationshipsbetweenobjects,
arelearnedtodescribetheconditionalprioritybetweendifferentobjectsintheimages.
Inparticular,wefirstlearnthelow-levelpriorsthatarestatisticallybasedonalargeset
ofnaturalimages.Then,thehigh-levelpriorsarelearnedtoconstructaconditionalprob-
abilitymatrixbluethatreflectstherelativerelationshipbetweendifferentobjects.Subse-
quently,asaliencymodelispresentedbyintegratingthelow-levelpriors,thehigh-level
priorsandtheCenterBiasPrior(CBP),inwhichtheweightsthatcorrespondtothelow-
levelpriorsandthehigh-levelpriorsarelearnedbasedontheeyetrackingdataset.The
experimentalresultsdemonstratethatourapproachoutperformstheexistingtechniques.
Ó2013ElsevierInc.Allrightsreserved.
1.Introduction
Thesurroundingenvironmentcontainsatremendousamountofvisualinformation,whichthehumanvisualsystem
(HVS)cannotfullyprocess[24].Therefore,theHVStendstopayattentiontoonlyafewpartswhileneglectingotherparts
ofascene.Thisphenomenonisusuallyreferredtobypsychologistsasvisualattention.Topredictautomaticallywherepeo-
plelookinanimage,visualattentionanalysishasbeeninvestigatedfordozensofyearsinthecomputervisionfield.How-
ever,untilnowithasbeenanopenproblemthathasyettobeaddressed.Recently,understandingcomputervisionproblems
fromtheviewpointofapsychologistisbecominganimportantresearchtrack.Becausevisualattentionisalsoanimportant
issueandhasbeenstudiedformorethanacenturyinthepsychologyfield,itisreasonabletoadoptsomeusefulconcepts
frompsychologytosolvethevisualattentionanalysisprobleminmultimediamodeling[10,17,29],imageretrieval
[21,23,30]andcomputervision[9,22].
Existingvisualattentionmethodscanbebrieflydividedintothreegroups,whicharebasedonthedifferentdrivingcon-
ditions,namely,theinformation-drivenmethod,thelow-levelfeature-drivenmethodandthehybridfeature-drivenmethod.
0020-0255/$-seefrontmatterÓ2013ElsevierInc.Allrightsreserved.
http:
//dx.doi.org/10.1016/j.ins.2013.09.036
⇑Correspondingauthor.
E-mailaddress:
brooksong@ieee.org(M.Song).
InformationSciencesxxx(2013)xxx–xxx
ContentslistsavailableatScienceDirect
InformationSciences
journalhomepage:
Pleasecitethisarticleinpressas:
M.Songetal.,Low-levelandhigh-levelpriorlearningforvisualsaliencyestimation,Inform.Sci.(2013),
http:
//dx.doi.org/10.1016/j.ins.2013.09.036
Theinformation-drivenmethods[2]makecontributionstothevisualattentionissuefromasignalprocessingperspec-
tive.HouandZhang[11]analyzethelogspectrumofeachimageandobtainthespectralresidual.Thespectralresidualis
transformedtothespatialdomaintoobtainasaliencymap.BruceandTsotsos[1,2]believethatthesaliencyregionprovides
moreinformationthanotherregions,andamethodcalled‘‘AttentionbasedonInformationMaximization(AIM)’’isproposed
tomaximizetheself-informationintheimage.Thisapproachperformsmarginallybetterthanthepreviousmodels.Zhang
etal.[36]furtherusethespatiotemporalvisualfeaturestogeneralizethestaticimagesaliencymodeltodynamicscenes,in
whichself-informationisemployedtorepresenttheinformativelevel.
Thelow-levelfeature-drivenmethodcomputesthesaliencymapfromthecontrastsandisbasedonasetoflow-level
features,suchasthecolor,intensity,andorientation.Theselow-levelfeaturesareextractedfromtheoriginalimageatdif-
ferentscalesandorientations.Thelow-levelfeature-drivenmethodperformswellforsomenaturescenesorsyntheticdata.
Ittietal.[14]computethesaliencyvalueusingacenter-surroundfiltertocapturethespatialdiscontinuity.Meuretal.pres-
entamethodtocomputethesaliencymapbasedonthefusionofseverallow-levelfeatures(intensity,color,orientation).
OlivaandTorralba[20]findthattheshapeofthesceneisalsoanimportantfactorforhumanperception.Theyprovidea
definitionofspatialenveloptodescribetheshapeofthesceneinvisualattentionanalysis.However,forthenaturalscenes
thathavecomplexscenarios,thelow-levelfeature-drivenmethodcannotpredictwherehumanlookcorrectly.Fig.1(b)isthe
saliencymapthatisgeneratedbyIttietal.[14],whichisobtainedfromcolor,intensityandorientationfeatures.Fig.1(c)is
thesaliencymapthatisobtainedbyOlivaandTorralba[20]andisbasedonthespatialenvelop.Therealeye-trackingdatais
giveninFig.1(e).Itisnoticeablethatthereisalargedistancebetweenthesaliencymapsandtherealeye-trackingdata.
Thehybridfeature-drivenmethodaccountsfornotonlythelow-levelfeaturesbutalsosomehigh-levelfeatures,suchas
face,humanandotherobjects[4,7,15],toobtainbetterresults.Thismethodisalsotreatedasaconcept-drivenmethod.Cerf
etal.[4]addfacedetectionintothelow-levelfeature-drivenmodel[14]andimprovethesaliencymap’saccuracysignifi-
cantly.Juddetal.[15]expandthehybridmodelfurther,whichincludesnotonlyhigh-levelfeaturesbutalsomid-levelfea-
tures(horizonline).Then,theytrainanSVMclassifierfromtheeye-trackingdatasettolearndifferentfeatures’parameters
forsaliencymapconstruction.Fig.1(d)showsthatitachievesbetterresultsthantheinformation-drivenmethod[14]and
thelow-levelfeature-drivenmethod[20].However,becausethismethodignorestheinter-relationshipsamongdifferent
high-levelfeatures(objects),thesalientareasofthemapdonotmatchtheeye-trackingdataverywell.
Apartfromtheabovethreegroupsofmethods,othermodels,suchasBayesianmodel[12,32],efficientcoding[25],and
multiviewlearning[31,34,33,28]providesomedifferentviewsforthetopicaswell.
Ourproposedtechniqueisatypeofhybridfeature-drivenmethod.Incontrasttotheprevioushybridfeaturedrivenmod-
el,ourapproachperformsbothlow-levelpriorlearningandhigh-levelfeaturelearningforvisualsaliencyestimation.Inthe
low-levelpriorlearningpart,theconceptof‘‘ChanceofHappening(CoH)’’isintroducedwhendeducingthelow-levelsal-
iencyvalue.Additionallytwolow-levelpriors,i.e.,ColorStatistics-basedPriors(CSP)andSpatialCorrelation-basedPriors
(SCP),arelearnedtodescribethecolordistributionandcontrastdistributioninnaturalimages,whichareusedtocompute
theCoHvalueaswellasthelow-levelsaliencyvalue.Inthehigh-levelpriorlearningpart,therelativerelationshipislearned
todescribetheconditionalprioritybetweendifferentobjectsinimages,whichisusedtocomputethehigh-levelsaliency
value.Afterward,anewsaliencymodelispresentedbyintegratingthelow-levelsaliency,thehigh-levelsaliencyandthe
CenterBiasPrior(CBP),inwhichtheweightsthatcorrespondtothelow-levelandthehigh-levelarelearnedbasedon
theeye-trackingdataset.
Fig.1.Comparisonofsomeexistingsaliencymodelsandeye-trackingdata.(a)Originalcolorimages,(b)Ittietal.saliencymaps[14],(c)OlivaandTorralba
saliencymaps[20](d)Juddetal.saliencymaps[15]and(e)eye-trackingdata.
2M.Songetal./InformationSciencesxxx(2013)xxx–xxx
Pleasecitethisarticleinpressas:
M.Songetal.,Low-levelandhigh-levelpriorlearningforvisualsaliencyestimation,Inform.Sci.(2013),
http:
//dx.doi.org/10.1016/j.ins.2013.09.036
Themajorcontributionsofthispaperinclude:
(1)anovelhybridfeature-drivenmodelispresentedtoperformbothlow-
levelpriorlearningandhigh-levelfeaturelearningforvisualsaliencyestimation;
(2)aconceptof‘‘ChanceofHappening’’for
low-levelpriorlearningisintroduced;and(3)relativerelationshipsaredefinedtodescribetheconditionalprioritybetween
differentobjectsinimages.
Therestofthispaperisorganizedasfollows.WediscussthemotivationoftheproposedapproachinSection2.Section3
describesourproposedvisualsaliencyestimation,whichaccountsforthelow-levelsaliency,thehigh-levelsaliencyandthe
centerbiasprior.ExperimentalresultsandanalysisaregiveninSection4.WefinallyconcludeinSection5.
2.Motivationoftheproposedmethod
ItisknownthatvisualstimuliarethemainreasonthattheHVSstayactiveandreadyforstimulitodrivethemovements
ofeye,whichleadstothevisualattentionmechanism.Accordingtotheresearchofpsychologists[13],visualstimulicanbe
dividedintotwodifferenttypesbasedonthereactiontimeofthevisualneurons.Onetypeisindependentofaspecifictask
andcanbeoperatedveryrapidlyin25–50msperitem.Theimage’scolor,intensity,andcontrastbelongtothisstimulus;itis
thesefeaturesthatthelow-levelfeature-drivenmethodisconcernedwith.Theothertypeisrelatedtosomecognitivefac-
tors,suchasknowledge,expectationsorcurrentgoals,e.g.,textorfaceinformation.Thistaskusuallytakes200msormore
forneuronstoreact.Fig.2showsbrieflythelow-levelandthehigh-levelvisualinformationthatareprocessedbythevisual
neuronsofHVS[13].First,thevisualinformation(atypicalimageofascene)iscapturedbythehumaneyesandentersthe
visualcortex.Then,thelow-levelinformationandthehigh-levelinformationareprocessedbytheinferotemporalcortexand
theposteriorparietalcortex,respectively.Afterward,someothervisualneurons(notshown)modulatetheseaspectsto-
gethertodrivethefinaleyemovement.
Forexample,theimageontherightofFig.2isanordinarystreetsceneinourdailylife.Fromtheviewpointoflow-level
saliency,thewhitebannerinthemiddlewillattractahuman’sattentionbecauseitsintensityisdifferentfromthesurround-
ings.Forthesamereason,twotelephoneboothsnearthedoorcanalsobenoticed.Thesedeductionsareinaccordancewith
theexperimentalresultsfromItti’ssaliencymodel[14].However,fromtheviewpointofahigh-levelfeature-drivenmet