HammersleyClifford定理.docx
《HammersleyClifford定理.docx》由会员分享,可在线阅读,更多相关《HammersleyClifford定理.docx(10页珍藏版)》请在冰豆网上搜索。
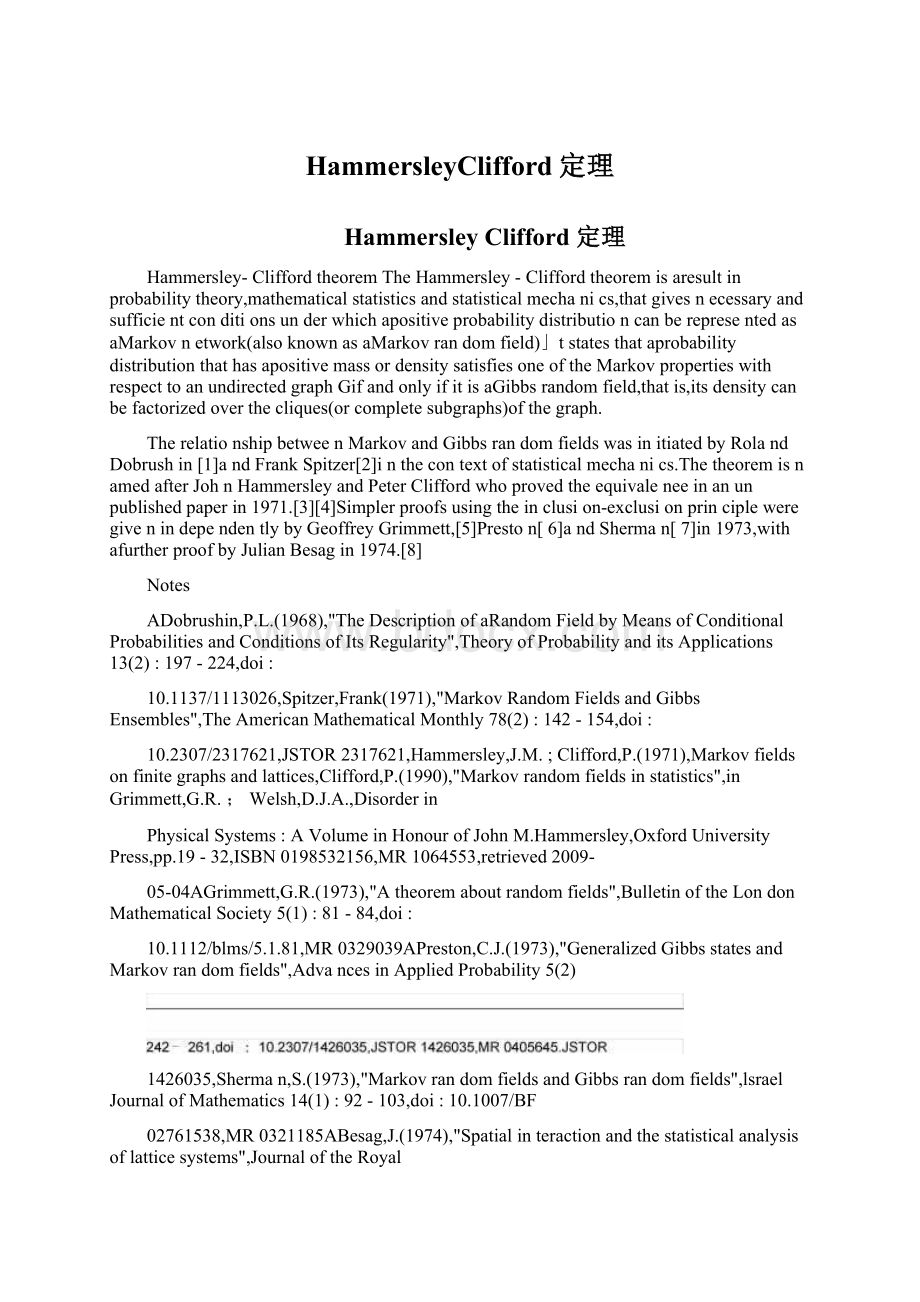
HammersleyClifford定理
HammersleyClifford定理
Hammersley-CliffordtheoremTheHammersley-Cliffordtheoremisaresultinprobabilitytheory,mathematicalstatisticsandstatisticalmechanics,thatgivesnecessaryandsufficientconditionsunderwhichapositiveprobabilitydistributioncanberepresentedasaMarkovnetwork(alsoknownasaMarkovrandomfield)」tstatesthataprobabilitydistributionthathasapositivemassordensitysatisfiesoneoftheMarkovpropertieswithrespecttoanundirectedgraphGifandonlyifitisaGibbsrandomfield,thatis,itsdensitycanbefactorizedoverthecliques(orcompletesubgraphs)ofthegraph.
TherelationshipbetweenMarkovandGibbsrandomfieldswasinitiatedbyRolandDobrushin[1]andFrankSpitzer[2]inthecontextofstatisticalmechanics.ThetheoremisnamedafterJohnHammersleyandPeterCliffordwhoprovedtheequivaleneeinanunpublishedpaperin1971.[3][4]Simplerproofsusingtheinclusion-exclusionprincipleweregivenindependentlybyGeoffreyGrimmett,[5]Preston[6]andSherman[7]in1973,withafurtherproofbyJulianBesagin1974.[8]
Notes
ADobrushin,P.L.(1968),"TheDescriptionofaRandomFieldbyMeansofConditionalProbabilitiesandConditionsofItsRegularity",TheoryofProbabilityanditsApplications13
(2):
197-224,doi:
10.1137/1113026,Spitzer,Frank(1971),"MarkovRandomFieldsandGibbsEnsembles",TheAmericanMathematicalMonthly78
(2):
142-154,doi:
10.2307/2317621,JSTOR2317621,Hammersley,J.M.;Clifford,P.(1971),Markovfieldsonfinitegraphsandlattices,Clifford,P.(1990),"Markovrandomfieldsinstatistics",inGrimmett,G.R.;Welsh,D.J.A.,Disorderin
PhysicalSystems:
AVolumeinHonourofJohnM.Hammersley,OxfordUniversityPress,pp.19-32,ISBN0198532156,MR1064553,retrieved2009-
05-04AGrimmett,G.R.(1973),"Atheoremaboutrandomfields",BulletinoftheLondonMathematicalSociety5
(1):
81-84,doi:
10.1112/blms/5.1.81,MR0329039APreston,C.J.(1973),"GeneralizedGibbsstatesandMarkovrandomfields",AdvancesinAppliedProbability5
(2)
1426035,Sherman,S.(1973),"MarkovrandomfieldsandGibbsrandomfields",lsraelJournalofMathematics14
(1):
92-103,doi:
10.1007/BF
02761538,MR0321185ABesag,J.(1974),"Spatialinteractionandthestatisticalanalysisoflatticesystems",JournaloftheRoyal
StatisticalSociety.SeriesB(Methodological)36
(2):
192-236,MR
0373208.JSTOR2984812FurtherreadingBilmes,Jeff(Spring2006),Handout2:
Hammersley-Clifford,coursenotesfromUniversityofWashingtoncourse.Grimmett,Geoffrey,ProbabilityonGraphs,Chapter7,Helge,TheHammersley-CliffordTheoremanditsImpactonModernStatistics,probability-relatedarticleisastub.YoucanhelpWikipediabyexpandingit.
Retrievedfrom"-Clifford_theorem"FromWikipedia,thefreeencyclopediaThefirstafternoonofthememorialsessionforJulianBesaginBristolwasanintenseandattimesemotionalmoment,wherefriendsandcolleaguesofJuliansharedmemoriesandstories.Thiscollectionoftributesshowedhowmuchofalarger-than-lifecharacterhewas,fromhisIong-termedandwide-rangedimpactonstatisticstohisveryhighexpectations,bothforhimselfandforothers,leadingtoatotalanduncompromisingresearchethics,tohispassionfor[extreme]sportsandoutdoors.(Thestoriesduringandafterdinerwereofamorepersonalnature,butatleastasmuchenjoyable!
)Thetalksonthesecondday
showedhowmuchandhowdeeplyJulianhadcontributedtospatialstatisticsandagriculturalexperiments,topseudo-likelihood,toMarkovrandomfieldsandimageanalysis,andtoMCMCmethodologyandpractice.IhopeIdidnotbotchtoomuchmypresentationonthehistoryofMCMC,whileIfoundreadingthroughthe1974,1986and1993ReadPapersandtheirdiscussionsanimmenselyrewardingexperiment(lwishIhaddonepriortocompletingourStatisticalSciencepaper,butitwasboundtobeincompletebynature!
).Someinterestinglinksmadebytheaudieneewere
thepriorpublicationofproofsoftheHammersley-Cliffordtheoremin1973(byGrimmet,Preston,andSteward,respectively),aswellastheproposalofaGibbssamplerbyBrianRipleyasearlyas1977(eventhoughHastingsdiduseGibbsstepsinoneofhisexamples).ChristopheAndrieualsopointedouttomeaveryearlyMonteCarloreviewbyJohnHaltoninthe1970SIAMRewiew,reviewthatIwillread(andcommment)assoonaspossible.Overall,IamquitegladIcouldtakepartinthismemorialandIamgratefultobothPetersfororganisingitasafittingtributeto
Julian.MarkovChainMonteCarlo(MCMC)methodsarecurrentlyaveryactivefieldofresearch.MCMCmethodsaresamplingmethods,basedonMarkov
Chainswhichareergodicwithrespecttothetargetprobabilitymeasure.Theprincipleofadaptivemethodsistooptimizeontheflysomedesignparametersofthealgorithmwithrespecttoagivencriterionreflectingthesampler'sperformance(optimizetheacceptaneerate,optimizeanimportaneesamplingfunction,etc…).Apostdoctoral
positionisopenedtoworkonthenumericalanalysisofadaptiveMCMCmethods:
convergence,numericalefficiency,developmentandanalysisofnewalgorithms.Aparticularemphasiswillbegiventoapplicationsinstatisticsandmoleculardynamics.(Detaileddescription)PositionfundedbytheFrenchNationalResearchAgency(ANR)throughthe2009-2012projectANR-08-BLAN-0218.Thepositionwillbenefitfromaninterdisciplinaryenvironmentinvolvingnumericalanalysts,statisticiansandprobabilists,andofstronginteractionsbetweenthepartnersoftheprojectANR-08-BLAN-021InthemostrecentissueofStatisticalScience,thespecialtopicis"CelebratingtheEMAlgorithm'sQuandunciacentennial".ItcontainsanhistoricalsurveybyMartinTannerandWingWongontheemergeneeofMCMCBayesiancomputationinthe1980's,Thissurveyismorefocusedandmoreinformativethanourglobalhistory(alsotoappearinStatisticalScience).Inparticular,itprovidestheauthors'analysisastowhyMCMCwasdelayedbytenyearsorso(orevenmorewhenconsideringthataGibbssamplerasasimulationtoolappearsinbothHastings'(1970)andBesag's(1974)papers).Theydismiss[our]concernsaboutcomputingpower(IwasrunningMonteCarlosimulationsonmyApplelieby1986andasinglemeansquareerrorcurveevaluationforaJames-Steintypeestimatorwouldthentakeclosetoaweekend!
)andMarkovinnumeracy,ratherattributingthereluctaneetoalackofconfideneeintothemethod.Thisperspectiveremainsdebatableas,apartfromTonyO'HaganwhowasthenfightingagainMonteCarlomethodsasbeingun-Bayesian(1987,JRSSD),ldonotrememberanynegativeattitudeatthetimeaboutsimulationandtheimmediatespreadoftheMCMCmethodsfromAlanGelfand'sandAdrianSmith'spresentationsoftheir1990papershowsontheoppositethattheBayesiancommunitywasreadyforthemove.
AnotherinterestingpointmadeinthishistoricalsurveyisthatMetropolis'andotherMarkovchainmethodswerefirstpresentedoutsidesimulationsectionsofbookslikeHammersleyandHandscomb(1964),Rubinstein(1981)andRipley(1987),perpetuatingtheimpressionthatsuchmethodsweremostlyoptimisationornichespecificmethods.ThisisalsowhyBesag'searlierworks(notmentionedinthissurvey)didnotgetwiderrecognition,untillater.SomethingIwasnot
sampling(i.e.populationMonteCarlo)ntheBayesianliteratureofthe1980's,withproposalsfromHermanvanDijk,AdrianSmith,andothers.The
即pendixaboutSmithetal.(1985),the1987specialissueofJRSSD,andthecomputationcontentsofValencia3(thatIsadlymissedforbeingintheArmy!
)isalsoquiteinformativeabouttheperceptionofcomputationalBayesianstatisticsatthistime.
AmissingconnectioninthissurveyisGillesCeleuxandJeanDiebolt'sstochasticEM(orSEM).Asearlyas1981,withMichelBroniatowski,theyproposedasimulatedversionofEMformixtureswherethelatentvariablezwassimulatedfromitsconditionaldistributionratherthanreplacedwithitsexpectation.SothiswasthefirsthalfoftheGibbssamplerformixtureswecompletedwithJeanDieboltabouttenyearslater.(AlsofoundinGelmanandKing,1990.)Theseauthorsdidnotgetmuchrecognitionfromthecommunity,though,astheyfocusedalmostexclusivelyonmixtures,usedsimulationtoproducearandomnessthatwouldescapethelocalmodeattraction,ratherthantargetingtheposteriordistribution,anddidnotanalysetheMarkoviannatureoftheiralgorithmuntillaterwiththesimulatedannealingEMalgorithm.
Share:
Share
概率图模型分为有向和无向的模型。
有向的概率图模型主要包括贝叶斯网络和隐马尔可夫模型,无向的概率图模型则主要包括马尔可夫随机场模型和条件随机场模型。
2001年,卡耐基.梅隆大学的Lafferty教授(JohnLafferty,AndrewMcCallum,FernandoPereira)等针对序列数据处理提出了CRF模型(ConditionalRandomFieldsProbabilisticModelsforSegmentingandLabelingSequeneeData)。
这种模型直接对后验概率建模,很好地解决了MRF莫型利用多特征时需要复杂的似
然分布建模以及不能利用观察图像中上下文信息的问题。
Kumar博士在2003年将
CRF模型扩展到2-维格型结构,开始将其引入到图像分析领域,吸引了学术界的高度关注。
对给定观察图像,估计对应的标记图像y观察图像,x未知的标记图像
1.如果直接对后验概率建模(即考虑公式中的第一项),可以得到判别的(Discriminative)概率框架。
特别地,如果后验概率直接通过Gibbs分布建模,
(x,y)称为一个CRF得到的模型称为判别的CRF模型。
2.通过对(x,y)的联合建模(即考虑公式中的第二项),可以得到联合的概率框架?
。
特别地,如果考虑双随机场(x,y)的马尔可夫性,即公式的第二项为Gibbs分布,那么(x,y)被称为一个双
MRF(PairwiseMRF,PMRF)[9]。
3.后验概率通过公式所示的p(x)和p(y|x)建模,其中p(y|x)为生成观察图像的模型,因此这种框架称为生成的(Generative)概率框
架。
特别地,如果先验p(x)服从Gibbs分布,x称为一个MRF[12],得到的模型称为生成的MRF莫型。
--【面向图像标记的随机场模型研究】运用Hammersley-Clifford定理,标记场的后验概率服从Gibbs分布
其中,z(y,9)为归一化函数,©c为定义在基团c上的带有参数0的势函数。
CRF模型中一个关键的问题是定义合适的势函数。
因此发展不同形式的扩展CRF模型是当前CRF模型的一个主要研究方向。
具体的技术途径包括:
一是扩展势函数。
通过引进更复杂的势函数,更多地利用多特征和上下文信息;二是扩展模型结构。
通过引入更复杂的模型结构,可以利用更高层次、更多形式的上下文信息。
扩展势函数
(1)对数回归(LogisticRegression,LR)
⑵支持向量机(SupportVectorMachine,SVM)
⑶核函数
(4)Boost
(5)Probit
扩展模型结构
(1)动态CRF模型
动态CRF(Dynamic