论文.docx
《论文.docx》由会员分享,可在线阅读,更多相关《论文.docx(45页珍藏版)》请在冰豆网上搜索。
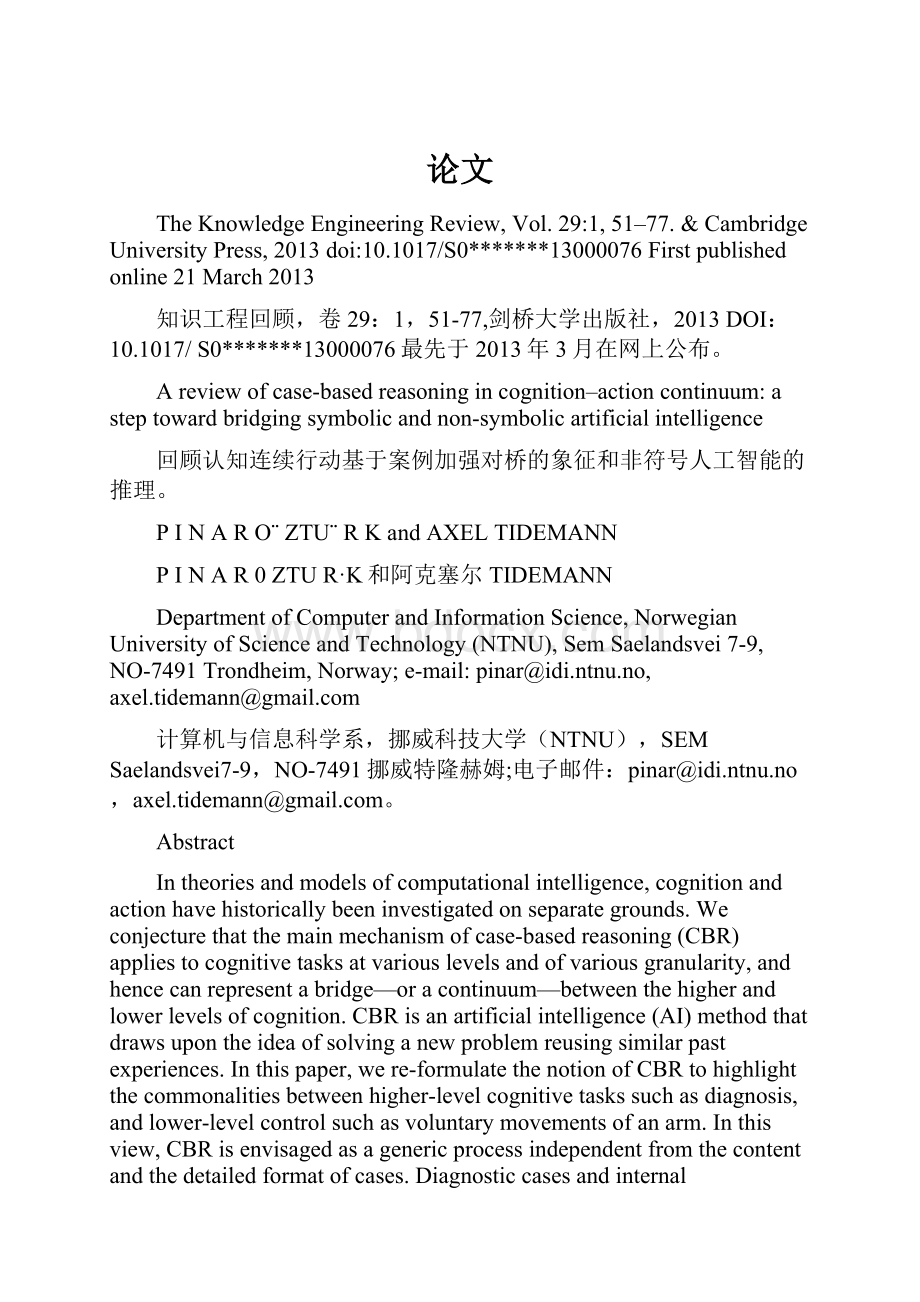
论文
TheKnowledgeEngineeringReview,Vol.29:
1,51–77.&CambridgeUniversityPress,2013doi:
10.1017/S0*******13000076Firstpublishedonline21March2013
知识工程回顾,卷29:
1,51-77,剑桥大学出版社,2013DOI:
10.1017/S0*******13000076最先于2013年3月在网上公布。
Areviewofcase-basedreasoningincognition–actioncontinuum:
asteptowardbridgingsymbolicandnon-symbolicartificialintelligence
回顾认知连续行动基于案例加强对桥的象征和非符号人工智能的推理。
PINARO¨ZTU¨RKandAXELTIDEMANN
PINAR0ZTUR·K和阿克塞尔TIDEMANN
DepartmentofComputerandInformationScience,NorwegianUniversityofScienceandTechnology(NTNU),SemSaelandsvei7-9,NO-7491Trondheim,Norway;e-mail:
pinar@idi.ntnu.no,axel.tidemann@
计算机与信息科学系,挪威科技大学(NTNU),SEMSaelandsvei7-9,NO-7491挪威特隆赫姆;电子邮件:
pinar@idi.ntnu.no,axel.tidemann@。
Abstract
Intheoriesandmodelsofcomputationalintelligence,cognitionandactionhavehistoricallybeeninvestigatedonseparategrounds.Weconjecturethatthemainmechanismofcase-basedreasoning(CBR)appliestocognitivetasksatvariouslevelsandofvariousgranularity,andhencecanrepresentabridge—oracontinuum—betweenthehigherandlowerlevelsofcognition.CBRisanartificialintelligence(AI)methodthatdrawsupontheideaofsolvinganewproblemreusingsimilarpastexperiences.Inthispaper,were-formulatethenotionofCBRtohighlightthecommonalitiesbetweenhigher-levelcognitivetaskssuchasdiagnosis,andlower-levelcontrolsuchasvoluntarymovementsofanarm.Inthisview,CBRisenvisagedasagenericprocessindependentfromthecontentandthedetailedformatofcases.Diagnosticcasesandinternalrepresentationsunderlyingmotorcontrolconstitutetwoinstantiationsofthecaserepresentation.Inordertoclaimsuchagenericmechanism,theaccountofCBRneedstoberevisedsothatitspositioninnon-symbolicAIbecomesclearer.ThepaperreviewstheCBRliteraturethattargetslowerlevelsofcognitiontoshowhowCBRmaybeconsideredasasteptowardbridgingthegapbetweensymbolicandnon-symbolicAI.
1Introduction
Intheoriesandmodelsofcomputationalintelligence,cognitionandactionhavehistoricallybeeninvestigatedonseparategrounds.Withinthemethodareaofartificialintelligence(AI),symbol-processingapproacheshaveemphasizedhigher-levelcognition,whilesituatedandembodiedAIhasmorefocusonthebodilyactivitiesofanagent.TheissueofrepresentationhasbecomeaneffectivedeparturepointcontributingtotheescalationoftensionbetweenthesetwolinesofAI.Inrecentyears,however,atrendhasemergedthatislookingforsimilaritiesandcomplementaritiesbetweenunder-lyingmechanismsofhigher-levelcognitionandlower-levelmotorbehavior.Ongoingresearchincognitivescienceaimsatprovidingcluesofaseamlesslinkbetweencognitionandaction,resultingfromtheclosecooperationbetweenresearchersacrossdisciplinessuchascognitivepsychology,linguistics,neuroscienceandAI,whopreviouslyinvestigatedthesameproblemsinisolationfromeachother.Animportanthypothesisthathasariseninthiscontextistheequivalenceoftheneuralmechanismsunderlyingovertmotorbehaviorandmotorimagery(i.e.thinkingofamotoractionwithoutexecutingit),whichimplicatestheexistenceofunderlyingrepresentations.Bothcognitivepsychology(Robinetal.,2007),philosophy(Grush,2004)andneuroscience(Filimonetal.,2007;Zimmermann-Schlatteretal.,2008)findingsprovideconvincingevidencesupportingthishypothesis.Knowledgemodelsofhigher-levelreasoningtasksincludegeneralizedmodelssuchasrule-based,frame-based,ornetwork-basedmodels,butalsoinstance-basedmodelssuchascollectionsofsituation-specificexperiences.Thelatteraretypicallycapturedbycaserepresentationsandcase-basedreasoning(CBR)methods(Kolodner,1993;deMa´ntarasetal.,2005).CBRdrawsfromresearchincognitivescienceonhumanmemoryandwasoriginallytargetedatexplainingexperience-basedbehaviorathigherlevelsofcognition(Schank,1982).Itrestsontheassumptionthatsimilarproblemsarelikelytohavesimilarsolutions,whichcanbeusedtosolvenewsimilarproblems.TwokeyindicatorsofCBRarecontext-sensitiverepresentationscouplingaconcreteproblemsituationwithacorrespondingsolution,andsimilarity-basedsearchforreusablecases.Besidesaplethoraofapplicationsinhigher-levelcognitionsuchasmedicaldiagnosisandtherapy,design,jobschedulingandplanning,CBRhasalso,morerecently,beenappliedinhighlydynamicdomainsthatcallfornon-symbolicrepresentationssuchasnavigation,real-timestrategygames,robotsoccerandactuatormovement.AlthoughCBRhasprovidedoutstandingresultsinhigher-levelcognitivetasks,ithassofarscarcelybeenemployedforlower-levelcontrolandevenlesssoincooperationwithneuroscientistsstudyingmovementplanning,whohaveprovidedinterestingfindingsaboutinternalrepresentationpertinenttoactuator-levelplanning.ThispaperhasaspecialfocusonhowCBRisappliedatthelowerlevelsofcognition–actioncontinuum,andthroughareviewofthecurrentstateoftheart,arguesthatCBRisagenericmechanismthatcanbeusedintheentirecognition–actioncontinuum.ThenotionofrepresentationhastriggeredamajordebateinAI.Brooks(1991),forexample,bannedrepresentationsinrobotbehaviorandmaintainedthat‘theworldisitsownbestrepresenta-tion’and‘explicitrepresentationsandmodelsoftheworldsimplygetintheway.Itturnsouttobebettertousetheworldasitsownmodel’.Weconjecturethatunificationofsymbolicandnon-symbolicAIisconditionedonareconciliationofthedifferentviewsofrepresentation,andwhere/whentheyareusedintheentirecognition–actioncontinuum.Ourstandpointisthatrepresentationsinthebrainexistformotor-levelcontrolaswellandareusedinaCBRprocess.Planninginbothhigherandlowerlevelsofcognition–actioncontinuumrelyonrepresentationspertinenttotheirowntargetdomains,althoughthetwotypesofAIhave,atthefirstsight,irreconcilableperspectivesontherepresentationsunderlyingthesemodels.FollowingMarkmanandDietrich(2000),thispaperstressesthatthenon-symbolic‘models’shouldbeincludedinthedefinitionofrepresentationbecausetheyarestoredforlateruseandcanberecalleduponencounteringsimilarsituations.OurhypothesisisthatthecomputationalframeworkforCBRmayencompasslower-levelcognitivetaskssuchasmotorcontrol,aswellashigher-levelreasoningtasks.Thereasoningisthatactionsmaybeatvariousabstractionlevels(O¨ztu¨rk,2009)whiletheunderlyingactionselectionmechanismmaybesimilarinvitalways.Proposingmarriage,orderingabloodtest,goingtothecinemaandliftingateacupareallactions,albeitatratherdifferentlevelsofgranularity.Theideaweadvanceisthat‘actionselection’innon-deterministicdomainsincludingpurpo-sefulactuatormovementiscaseselection.ButwhyisapplyingCBRtosuchlow-levelmechanismsasmovementlearningandcontrolimportant?
Fromthebiologicalplausibilityperspective,theaforementionedresearchfindingsinneuroscienceindicatethatthemotorsystemdealsnot(only)withpurelyreactivestimulus-responsetypeofprocessesbutoftenwithstimulus-response-consequencetypeofprocesseswheretheconsequencesarecomputedthroughanticipation,animportantpartofthewholeenterprise(Herwig&Waszak,2009;Shinetal.,2010).Thenotionofanticipationinitselfisavitalissuewithrespecttothediscussionwhethertouserepresentationsornot,providingsupportingevidencetotheubiquityofrepresentationsintheentirecognition–actioncontinuum.Furthermore,studiesinneuroscienceonimitation-basedmotorcontrolalsosupporttheexistenceofmultiplecontext-sensitiverepresentationsunderlyingactuatormovement.Theoutlineofthepaperisasfollows.Followingtheintroduction,maincharacteristicsofaCBRsystemandthetasksofthesystemdesignerareintroducedinSection2.Section3conveystherationalebehindourhypothesisthatcognitionandactionconstituteacontinuuminvolvingactionselectionatvariouslevels,whyCBRunderliestheentirecognition–actioncontinuumandwhyitprovidesabridgetounifysymbolicandsub-symbolicAI.Section4reviewsCBRsystemsthatdealwithreal-timedynamicdomainssuchasnavigation,robotsoccerandreal-timestrategicalgames.Section5discussestheroleofimitationinvariousdisciplines,withaparticularfocuson
52P.O¨ZTU¨RKANDA.TIDEMANN
neuroscience,followedbyadiscussionoftheroleofrepresentationsinhumanmotorcontrolandplanninginSection6.Section7presentsanarchitectureaboutimitation-basedmotorcontrolandlearning,suggestedbyneuroscientists.ThisisapreparationfortheotherpartofthereviewofCBRsystems,whichfocusesonthelowerlevelsofcognition–actiontasks,inSections8,9and10.Finally,Section11discusseswhythereviewedCBRsystemsstrengthenthepossibilitythatCBRplaysanimportantroleinconnectingsymbolicandsub-symbolicAI.
2MaincharacteristicsanddesigntasksofCBRsystems
ThemainargumentofusingCBRingeneralisthatitdoesnotneedanextensiveanddeepdomainmodelandreliesinsteadonexperience-based,compiledknowledge,whichhumansareknowntogatherduringandafterproblemsolving.ThedistinctiveroleofCBRinsymbolicAIisitsabilityoffastreasoningcomparedwithreasoningwithdeepdomainknowledge.Anexperienceofsolvingaproblemenrichestheexperientialmemoryofhumans(andanimals,ingeneral)and,ifproperlystored,experiencescanbeusefulinsolvingnew,butsimilarproblems.ACBRprocesshasanumberofcharacteristicsubprocesses,asshowninFigure1.