chapter4MapReduceandDesignPatternsWord文件下载.docx
《chapter4MapReduceandDesignPatternsWord文件下载.docx》由会员分享,可在线阅读,更多相关《chapter4MapReduceandDesignPatternsWord文件下载.docx(29页珍藏版)》请在冰豆网上搜索。
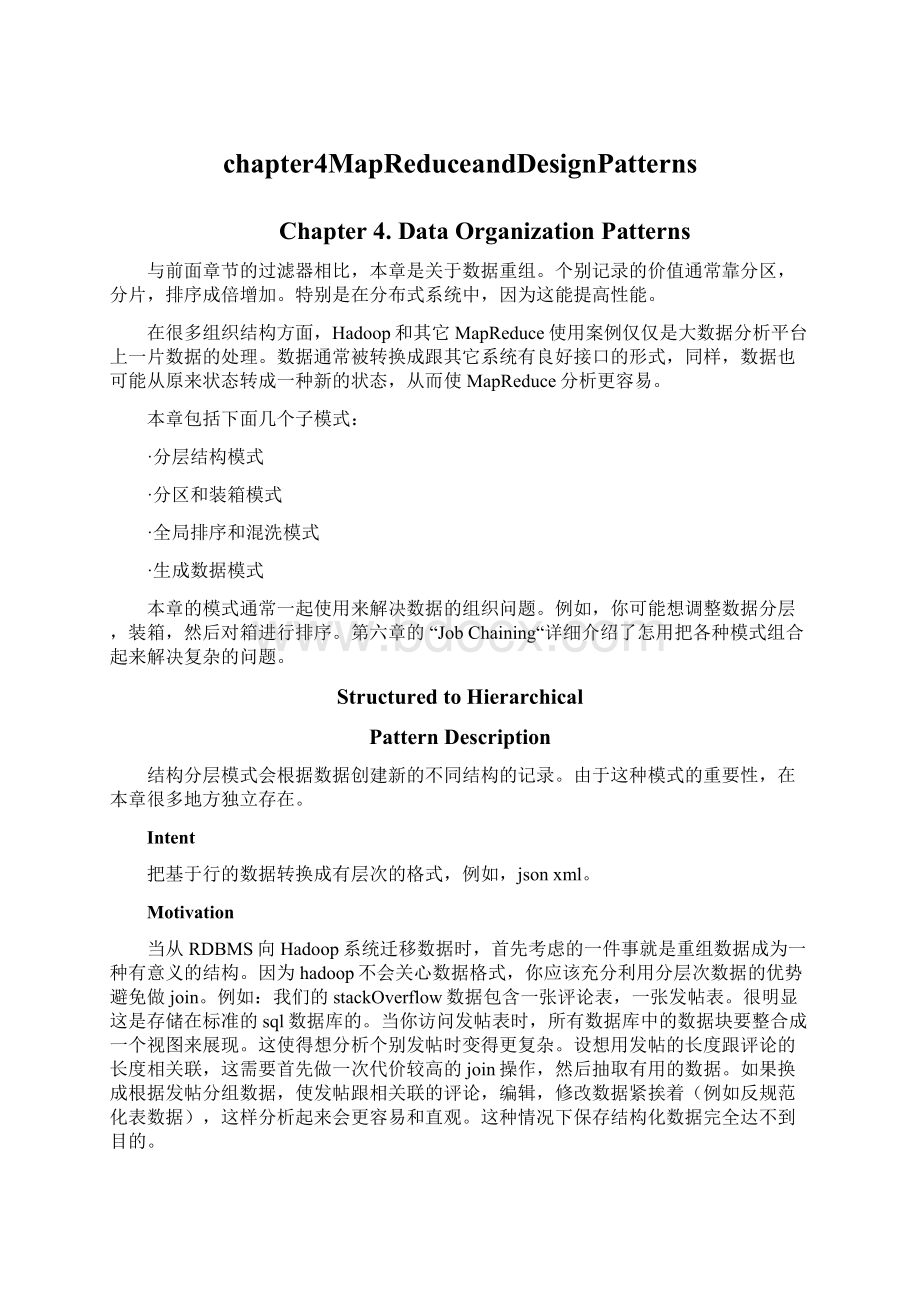
这使得想分析个别发帖时变得更复杂。
设想用发帖的长度跟评论的长度相关联,这需要首先做一次代价较高的join操作,然后抽取有用的数据。
如果换成根据发帖分组数据,使发帖跟相关联的评论,编辑,修改数据紧挨着(例如反规范化表数据),这样分析起来会更容易和直观。
这种情况下保存结构化数据完全达不到目的。
不幸的是,数据不总是分组在一块。
当某人回复了stackOverflow的某个问题,hadoop不能够把这条记录立刻插到层次数据中。
因此,用MapReduce创建非结构化记录只适用于周期性的批处理形式的业务逻辑。
另一种能平稳更新数据的方式是用Hbase。
Hbase能存储半结构化和层次样式的数据。
MongoDB也能很好的处理这种数据的排序。
Applicability
这种模式适合的场景:
你的数据靠很多外键相联系
你的数据是结构化的基于行的
Structure
图4-1展示了这种模式的结构,每部分组件描述如下:
如果你想合并多个数据源成为一个有层次的数据结构,hadoop中有个类:
org.apache.hadoop.mapreduce.lib.input.MultipleInputs很适合使用(貌似很老的包)。
Mutipleinputs允许你对不同的input使用不同的inputpath和不同的mapper。
Driver里完成配置。
如果只有一个来源,则不需要这一步。
mapper加载数据并解析成紧凑的格式从而使reducer更容易。
输出key应该是你想要标识的每一条层次记录的根。
例如,在stackOverflow例子里,根是发帖id。
也需要给没片数据标注来源信息。
也需要标识输出记录是发帖还是评论。
这样的话,就能简单连接这些标签并输出值。
通常,这里用combiner起不了多大作用。
可以用相同的key对条目分组,一起发送,但这样没有压缩所起到的好处,因为要做的知识连接字符串,所以输出大小不变。
reducer按key从不同的源接收数据。
所有的数据对指定的分组,每组都会产生一个迭代器,剩下你需要做的就是用数据条目构建有层次的数据结构。
使用xml或json,可以构建一个简单的对象并输出。
这部分的例子使用xml,提供了几个方便的方法处理结构化数据。
如果使用其他格式,例如自定义的格式,也要使用合适的构建对象的方法和序列化方法。
Consequences
输出是一种有层次的形式,根据指定的key分组的。
注意很多格式例如xmljson都有某种顶层根元素包在所有记录外面。
如果想让文档从根到底部都有良好的格式,也比较容易在特定的处理阶段加上头部或尾部。
Figure4-1.Thestructureofthestructuredtohierarchicalpattern
Knownuses
Pre-joiningdata
数据是杂乱的结构化数据集,为了分析,也很容易把数据组合成更复杂的对象。
通过这样,你设置好数据来充分利用分析nosql模型的优势。
PreparingdataforHBaseorMongoDB
Hbase是很自然的存储这类数据的方式。
所以可以用这种方法把数据搞到一起,作为加载到hbase或mongoDB的准备工作。
创建hbase表,然后通过MapReduce执行大量导入是很高效的。
另一种方案是分几次导入,可能效率较低。
Resemblances
Sql
RDB中这样的事情是很少见的,因为这样存储用sql分析不是很方便。
然而,这种方式可以解决RDBMS中的类似问题,比如做join然后在结果上做分析。
Pig
Pig对层次数据有适当的支持,可以取到层次的包和元组,然后就能容易的展现出层次结构和列出单条记录的对象。
Pig中的cogroup方法会保存原始结构做大量的合并数据的操作。
然而,使用这个关键词在复杂记录上做任何种类的实时分析都会有挑战性。
所以,自定义个方法是好的选择。
基本做法是使用pig创建并分组记录,然后用udf去分析数据。
data_a=LOAD'
/data/comments/'
ASPigStorage('
|'
);
data_b=LOAD'
/data/posts/'
'
grouped=COGROUPdata_aBY$2,data_bBY$1;
analyzed=FOREACHgroupedGENERATEudfs.analyze(group,$1,$2);
。
Performanceanalysis
使用这种模式时有两个性能关注点。
第一个,需要知道mapper发送到reducer的数据量,第二个,要清楚reducer创建的对象的内存使用情况。
因为要使用key分组的记录可以遍布在数据集的任何地方,很多数据会通过网络传输。
基于此原因,你需要注意使用适当的reducer的数量。
策略跟其它模式中的一样。
下一个主要关注的是数据的热点问题的可能性,它可以导致记录的暴增。
对大的数据集,一个特殊的输出记录会有很多数据与之关联是很可能出现的情况。
设想下stackOverflow上发了一个帖子,有一百万的评论。
这种情况相对少见,但不是不可能的。
如果你在创建某种xml对象,所有的评论某一时刻在输出之前都会存储在内存里。
这可能造成jvm的OOM,这种情况应该避免。
另一个热点问题是reducer处理的数据的倾斜问题。
这跟普通MapReducejob可能遇到的问题相似。
很多时候,倾斜问题可以忽略,如果倾斜很严重就写个自定义的partitioner使数据分发得更均匀。
StructuredtoHierarchicalExamples
Post/commentbuildingonStackOverflow
这个例子里,我们将拿到stackOverflow的发帖和评论数据并分组。
层次结构如下所示:
Posts
Post
Comment
问题:
给出用户发帖和评论数据,创建结构化的xml层次结构,将相关的评论嵌套在对应的发帖之内。
Drivercode。
我们通常不会描述驱动代码,但这个例子中会加入新的东西:
MultipleInput。
创建这个对象,把评论数据和发帖数据的路径加到指定的mapper。
这两个路径是通过命令行提供的,通过args数组获得。
publicstaticvoidmain(String[]args)throwsException{
Configurationconf=newConfiguration();
Jobjob=newJob(conf,"
PostCommentHierarchy"
job.setJarByClass(PostCommentBuildingDriver.class);
MultipleInputs.addInputPath(job,newPath(args[0]),
TextInputFormat.class,PostMapper.class);
MultipleInputs.addInputPath(job,newPath(args[1]),
TextInputFormat.class,CommentMapper.class);
job.setReducerClass(UserJoinReducer.class);
job.setOutputFormatClass(TextOutputFormat.class);
TextOutputFormat.setOutputPath(job,newPath(args[2]));
job.setOutputKeyClass(Text.class);
job.setOutputValueClass(Text.class);
System.exit(job.waitForCompletion(true)?
0:
2);
}
Mappercode。
这里有两个mapper类,分别是评论和发帖类。
两者都用发帖id作为输出key。
输出值用字符(p代表发帖,c代表评论),我们在reduce阶段就会知道数据来自哪里。
publicstaticclassPostMapperextendsMapper<
Object,Text,Text,Text>
{
privateTextoutkey=newText();
privateTextoutvalue=newText();
publicvoidmap(Objectkey,Textvalue,Contextcontext)
throwsIOException,InterruptedException{
Map<
String,String>
parsed=MRDPUtils.transformXmlToMap(value
.toString());
//TheforeignjoinkeyisthepostID
outkey.set(parsed.get("
Id"
));
//Flagthisrecordforthereducerandthenoutput
outvalue.set("
P"
+value.toString());
context.write(outkey,outvalue);
}
}
publicstaticclassCommentMapperextendsMapper<
parsed=MRDPUtils.transformXmlToMap(value.toString());
PostId"
C"
Reducercode。
Reducer创建有层次的xml对象。
所有的值被迭代,来得到发帖记录和对应的评论列表。
我们知道根据标识分别记录并加到值里。
标识在指派为帖子(对应为帖子)或加到list(对应为评论)时移除掉。
如果帖子不为空,就按帖子为父节点,评论为子节点创建xml记录。
紧随其后的是nestElements。
使用xmlapi创建xml记录,你可以在需要时尽情使用。
publicstaticclassPostCommentHierarchyReducerextends
Reducer<
Text,Text,Text,NullWritable>
privateArrayList<
String>
comments=newArrayList<
();
privateDocumentBuilderFactorydbf=DocumentBuilderFactory
.newInstance();
privateStringpost=null;
publicvoidreduce(Textkey,Iterable<
Text>
values,Contextcontext)
//Resetvariables
post=null;
comments.clear();
//Foreachinputvalue
for(Textt:
values){
//Ifthisisthepostrecord,storeit,minustheflag
if(t.charAt(0)=='
P'
){
post=t.toString().substring(1,t.toString().length())
.trim();
}else{
//Else,itisacommentrecord.Addittothelist,minus
//theflag
comments.add(t.toString()
.substring(1,t.toString().length()).trim());
}
}
//Iftherearenocomments,thecommentslistwillsimplybeempty.
//Ifpostisnotnull,combinepostwithitscomments.
if(post!
=null){
//nestthecommentsunderneaththepostelement
StringpostWithCommentChildren=nestElements(post,comments);
//writeouttheXML
context.write(newText(postWithCommentChildren),
NullWritable.get());
nestElements方法拿到帖子和评论的列表,创建一个xml字符串并输出。
使用DocumentBuilder和一些额外的帮助方法拷贝Element对象为新的,还有他们的属性。
这种拷贝发生在数据行转为帖子或评论时的重命名标签时。
最终的document被转换成了xml。
privateStringnestElements(Stringpost,List<
comments){
//CreatethenewdocumenttobuildtheXML
DocumentBuilderbldr=dbf.newDocumentBuilder();
Documentdoc=bldr.newDocument();
//Copyparentnodetodocument
ElementpostEl=getXmlElementFromString(post);
ElementtoAddPostEl=doc.createElement("
post"
//Copytheattributesoftheoriginalpostelementtothenewone
copyAttributesToElement(postEl.getAttributes(),toAddPostEl);
//Foreachcomment,copyittothe"
node
for(StringcommentXml:
ElementcommentEl=getXmlElementFromString(commentXml);
ElementtoAddCommentEl=doc.createElement("
comments"
//Copytheattributesoftheoriginalcommentelementto
//thenewone
copyAttributesToElement(commentEl.getAttributes(),
toAddCommentEl);
//Addthecopiedcommenttothepostelement
toAddPostEl.appendChild(toAddCommentEl);
//Addthepostelementtothedocument
doc.appendChild(toAddPostEl);
//TransformthedocumentintoaStringofXMLandreturn
returntransformDocumentToString(doc);
privateElementgetXmlElementFromString(Stringxml){
//Createanewdocumentbuilder
returnbldr.parse(newInputSource(newStringReader(xml)))
.getDocumentElement();
privatevoidcopyAttributesToElement(NamedNodeMapattributes,
Elementelement){
//Foreachattribute,copyittotheelement
for(inti=0;
i<
attributes.getLength();
++i){
AttrtoCopy=(Attr)attributes.item(i);
element.setAttribute(toCopy.getName(),toCopy.getValue());
privateStringtransformDocumentToString(Documentdoc){
TransformerFactorytf=TransformerFactory.newInstance();
Transformertransformer=tf.newTransformer();
transformer.setOutputProperty(OutputKeys.OMIT_XML_DECLARATION,
"
yes"
StringWriterwriter=newStringWriter();
transformer.transform(newDOMSource(doc),newStreamResult(writer));
//Replaceallnewlinecharacterswithanemptystringtohave
//onerecordperline.
returnwriter.getBuffer().toString().replaceAll("
\n|\r"
"
"
Question/answerbuildingonStackOverflow
本例是前面例子的后续处理,会使用前面例子的输出作为本例的输入数据。
现在,我们已经得到了帖子和跟帖子关联的评论,现在我们要关联帖子的提问和帖子的回答。
这是需要做的,因为帖子由回答帖和提问帖构成,并根据postTypeId区分。
我们将根据提问的id和回答的父id分组到一起。
使用前面例子的输出,执行自关联操作,创建问题帖,回答帖,和评论的层次结构。
首先决定记录是问题还是回答,因为这两种记录的行为不同。
对于问题,抽取它的id作为key,并标记为问题。
对于回答,抽取父id作为key,并标记为回答。
publicclassQuestionAnswerBuildingDriver{
publicstaticclassPostCommentMapperextends
Mapper<
//Parsethepost/commentXMLhierarc