观察性研究中的因果推断方法二30分钟_精品文档PPT格式课件下载.ppt
《观察性研究中的因果推断方法二30分钟_精品文档PPT格式课件下载.ppt》由会员分享,可在线阅读,更多相关《观察性研究中的因果推断方法二30分钟_精品文档PPT格式课件下载.ppt(39页珍藏版)》请在冰豆网上搜索。
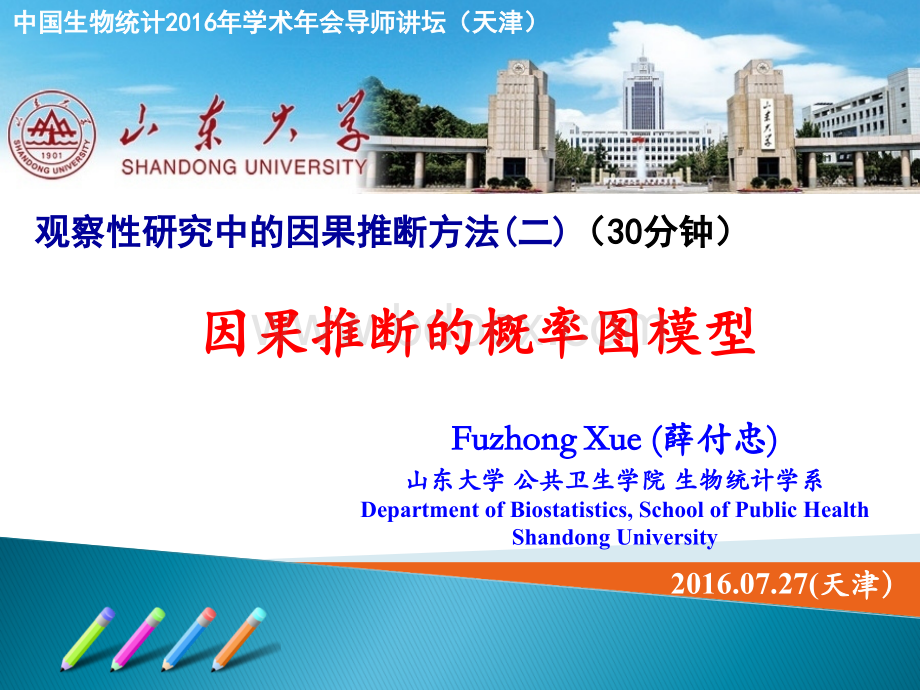
优优点是利用点是利用“图图+概率概率”的方式直观清晰的表达变的方式直观清晰的表达变量之间的时序关系、相关关系或因果关系等多种量之间的时序关系、相关关系或因果关系等多种语义,特别清晰地表达交互效应、效应修饰、中语义,特别清晰地表达交互效应、效应修饰、中介效应、混杂偏倚、选择偏倚和信息偏倚等多种介效应、混杂偏倚、选择偏倚和信息偏倚等多种因果推断关键问题。
缺点是主要适于分类变量间因果推断关键问题。
缺点是主要适于分类变量间的因果推断。
的因果推断。
l反事实病因模型(反事实病因模型(potential-outcomepotential-outcomecounterfactual)modelscounterfactual)models):
我们只能得到个体我们只能得到个体uu受到干预的数据受到干预的数据YtYt,或者个体,或者个体uu没有受到干预的没有受到干预的数据数据YcYc,但不能同时得到这两个数据。
因此,在,但不能同时得到这两个数据。
因此,在没有假设的前提下,不可能在个体层面上进行因没有假设的前提下,不可能在个体层面上进行因果推断。
方法是假设两个个体是相同的,采用人果推断。
方法是假设两个个体是相同的,采用人工随机化或自然随机化方式分组,观察暴露与结工随机化或自然随机化方式分组,观察暴露与结局的因果关系。
优点是能定量分析因果关系。
局的因果关系。
GreenlandS,etal.IntJEpidemiol.2002;
31(5):
1030-7.4Introductiontostrategiesforcausalinferences因果推断的四种基本策略因果推断的四种基本策略l充分充分/组合病因模型(组合病因模型(sufficient-componentsufficient-componentcausemodelscausemodels):
任何疾病涉及许多组合病因的任何疾病涉及许多组合病因的结合结合,而这些病因成分的联合作用而这些病因成分的联合作用,即充分病因自即充分病因自身的群集效应。
在解释一些复杂病因关系上身的群集效应。
在解释一些复杂病因关系上,具有具有很好的直观性和合理性很好的直观性和合理性,是病因网说的一大发展是病因网说的一大发展,并具有一定的疾病防治意义。
并具有一定的疾病防治意义。
l结构方程病因模型(结构方程病因模型(structural-equationsstructural-equationsmodelsmodels):
主要是为了验证假设的因果关系主要是为了验证假设的因果关系,融合,融合了因素分析和路径分析的多元统计技术,整合了由了因素分析和路径分析的多元统计技术,整合了由因子分析所代表的潜在变量研究模型与路径分析所因子分析所代表的潜在变量研究模型与路径分析所代表的传统线性因果关系模型,特别适于定量因果代表的传统线性因果关系模型,特别适于定量因果关系的验证。
关系的验证。
1030-7.5IntroductiontostrategiesforcausalinferencesIntroductiontostrategiesforcausalinferencesDenitionsofcausationinthestatisticalliteratureDenitionsofcausationinthestatisticalliteratureBLDeStavola|CausalmodellingCausaldiagramCausalDirectedAcyclicGraphs(DAG)DenitionsofcausationinthestatisticalliteratureDenitionsofcausationinthestatisticalliteratureBLDeStavola|CausalmodellingCausaldiagramCausalDirectedAcyclicGraphs(DAG)DenitionsofcausationinthestatisticalliteratureDenitionsofcausationinthestatisticalliteratureBLDeStavola|CausalmodellingnCausalgraphmodels(JudeaPearlsframework)Causalgraphmodels(JudeaPearlsframework)directedacyclicgraphMathematicallyformalizedbynPearl(1988,1995,2000)nSprites,Glymour,andScheines(1993,2000)9UniversityofCalifornia,LosAngeles(UCLA)CausaldiagramCausalDirectedAcyclicGraphs(DAG)nCausalgraphmodels(JudeaPearlsframework)Causalgraphmodels(JudeaPearlsframework)10CausaldiagramCausalDirectedAcyclicGraphs(DAG)nCausalgraphmodels(JudeaPearlsframework)Causalgraphmodels(JudeaPearlsframework)BLDeStavola|Causalmodelling11CausaldiagramCausalDirectedAcyclicGraphs(DAG)WhyDAGs?
WhyDAGs?
Elwertwisc.edu.Version5/2013ngnDAGsgraphicallyrepresentDAGsgraphicallyrepresentnon-parametricstructuralequationmodelsnon-parametricstructuralequationmodels.Theymaylooklikethepathmodelsofyore,buttheyarefarmoregeneral.Theymaylooklikethepathmodelsofyore,buttheyarefarmoregeneral.Rigorousmathematicalobjects,supportproofsRigorousmathematicalobjects,supportproofsVerygeneral(nonparametric)Verygeneral(nonparametric)Formanypurposes,DAGsaremoreaccessibleFormanypurposes,DAGsaremoreaccessiblethanpotentialoutcomesnotationthanpotentialoutcomesnotationAllpictures,noalgebraAllpictures,noalgebraFocusattentiononcausalassumptionsFocusattentiononcausalassumptions(languageofappliedscientists)(languageofappliedscientists)Greatforderiving(nonparametric)Greatforderiving(nonparametric)identificationresultsidentificationresultsGreatforderivingthetestableimplicationsofaGreatforderivingthetestableimplicationsofacausalmodelcausalmodelIntuitionforunderstandingmanyproblemsinIntuitionforunderstandingmanyproblemsincausalinference.causalinference.ParticularlyhelpfulforcomplexcausalmodelsParticularlyhelpfulforcomplexcausalmodelsLimitationsLimitationsDontdisplaytheparametricDontdisplaytheparametricassumptionsthatareoftenassumptionsthatareoftennecessaryforestimationinnecessaryforestimationinpractice.practice.GeneralitycanobscureGeneralitycanobscureimportantdistinctionsbetweenimportantdistinctionsbetweenestimands.estimands.CausaldiagramCausaldiagramCausalDirectedAcyclicGraphs(DAG)CausalDirectedAcyclicGraphs(DAG)R1D1S1D2R2D1dxD2dxS2I1I2?
AnExample:
acausaldiagramforgastroesophagealreflux(胃食管反流)andesophagealdisease(食管疾病).R=reflux(反流)S=symptoms(症状)T=treatment(治疗)I=imaging(影像表型)D=esophagusstatus(食管病变