灰色关联度matlab源程序完整版整理精品范本.docx
《灰色关联度matlab源程序完整版整理精品范本.docx》由会员分享,可在线阅读,更多相关《灰色关联度matlab源程序完整版整理精品范本.docx(16页珍藏版)》请在冰豆网上搜索。
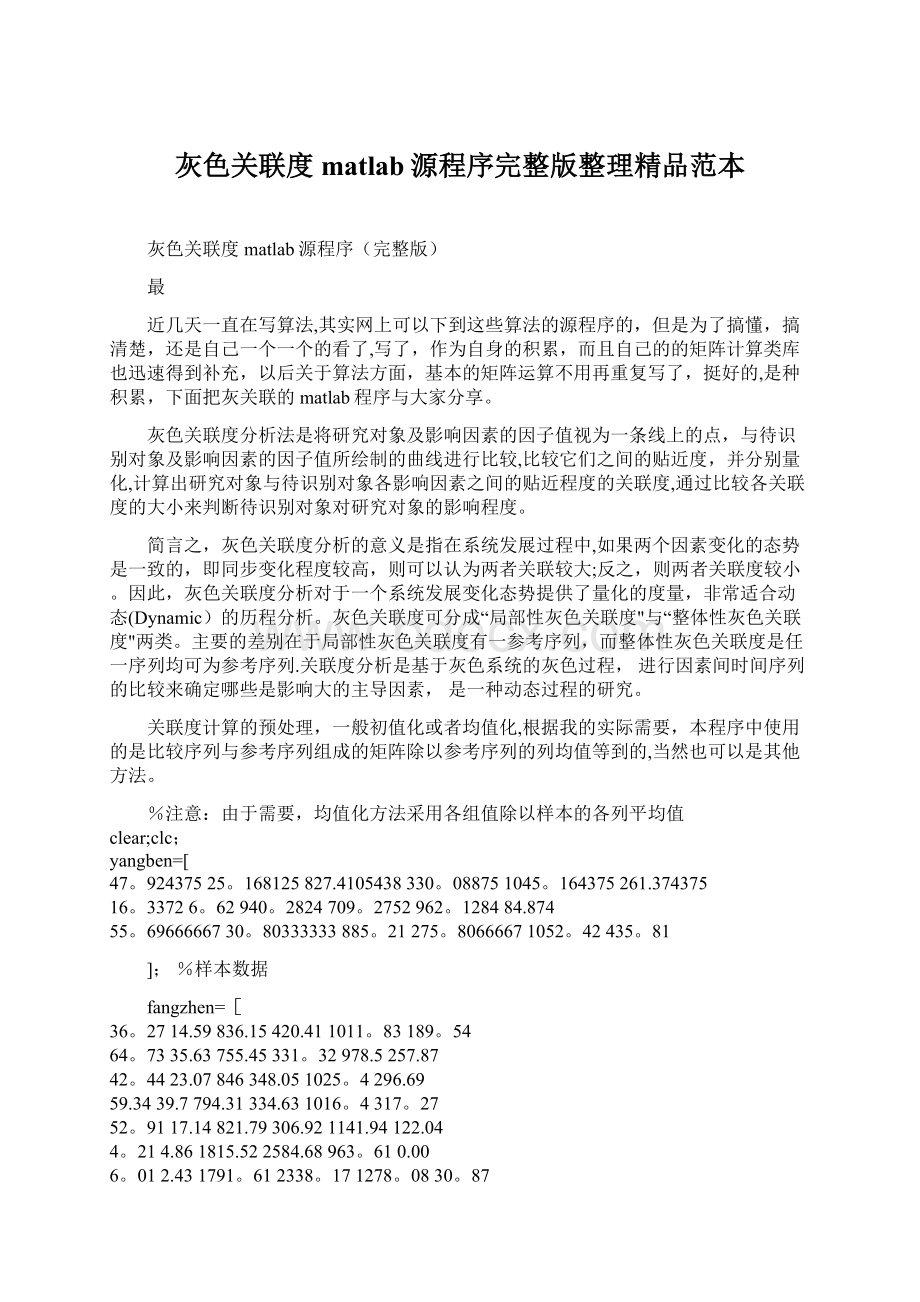
灰色关联度matlab源程序完整版整理精品范本
灰色关联度matlab源程序(完整版)
最
近几天一直在写算法,其实网上可以下到这些算法的源程序的,但是为了搞懂,搞清楚,还是自己一个一个的看了,写了,作为自身的积累,而且自己的的矩阵计算类库也迅速得到补充,以后关于算法方面,基本的矩阵运算不用再重复写了,挺好的,是种积累,下面把灰关联的matlab程序与大家分享。
灰色关联度分析法是将研究对象及影响因素的因子值视为一条线上的点,与待识别对象及影响因素的因子值所绘制的曲线进行比较,比较它们之间的贴近度,并分别量化,计算出研究对象与待识别对象各影响因素之间的贴近程度的关联度,通过比较各关联度的大小来判断待识别对象对研究对象的影响程度。
简言之,灰色关联度分析的意义是指在系统发展过程中,如果两个因素变化的态势是一致的,即同步变化程度较高,则可以认为两者关联较大;反之,则两者关联度较小。
因此,灰色关联度分析对于一个系统发展变化态势提供了量化的度量,非常适合动态(Dynamic)的历程分析。
灰色关联度可分成“局部性灰色关联度"与“整体性灰色关联度"两类。
主要的差别在于局部性灰色关联度有一参考序列,而整体性灰色关联度是任一序列均可为参考序列.关联度分析是基于灰色系统的灰色过程,进行因素间时间序列的比较来确定哪些是影响大的主导因素,是一种动态过程的研究。
关联度计算的预处理,一般初值化或者均值化,根据我的实际需要,本程序中使用的是比较序列与参考序列组成的矩阵除以参考序列的列均值等到的,当然也可以是其他方法。
%注意:
由于需要,均值化方法采用各组值除以样本的各列平均值
clear;clc;
yangben=[
47。
92437525。
168125827.4105438330。
088751045。
164375261.374375
16。
33726。
62940。
2824709。
2752962。
128484.874
55。
6966666730。
80333333885。
21275。
80666671052。
42435。
81
];%样本数据
fangzhen=[
36。
2714.59836.15420.411011。
83189。
54
64。
7335.63755.45331。
32978.5257.87
42。
4423.07846348.051025。
4296.69
59.3439.7794.31334.631016。
4317。
27
52。
9117.14821.79306.921141.94122.04
4。
214.861815.522584.68963。
610.00
6。
012.431791。
612338。
171278。
0830。
87
3。
011。
581220。
54956。
141244.753。
91
25。
657。
42790.17328.881026.0192.82
115.8027926.5350。
931079.49544。
38
12.638。
751055。
501379.00875。
101.65
];%待判数据
[rows,cols]=size(fangzhen);
p=0。
5; %分辨系数
[m,n]=size(yangben);
R=[];
forirow=1:
rows
yy=fangzhen(irow,:
);
data=[yy;yangben];
data_gyh1=mean(yangben)
fori=1:
m+1
forj=1:
n
data_gyh(i,j)=data(i,j)/data_gyh1(j);
end
end
fori=2:
m+1
forj=1:
n
Dij(i—1,j)=abs(data_gyh(1,j)-data_gyh(i,j));
end
end
Dijmax=max(max(Dij));
Dijmin=min(min(Dij));
fori=1:
m
forj=1:
n
Lij(i,j)=(Dijmin+p*Dijmax)/(Dij(i,j)+p*Dijmax);
end
end
LijRowSum=sum(Lij');
fori=1:
m
Rij(i)=LijRowSum(i)/n;
end
R=[R;Rij];
end
R
matlab求灰色关联度矩阵源代码
2010—12-1122:
57
functiongreyrelationaldegree(X,c)
%GRAYRELATIONALDEGREEthisfunctionisusedforcalculatingthegery
%relationbetweensquence
%rememeberthatthefirstcolumnoftheinputmatrixisthedesicion
%attributionsquences。
whatwewanttocalculateisthegreyralationaldegreebetween
%itandotherattributions
%Xisthesquencematrix,cistheparameterusedinthefunction
%inmostofthetime,thevalueofcis0.5
firstrow=X(1,:
);
reci_firstrow=1。
/firstrow;
reci_convert=diag(reci_firstrow);
initialMIRROR=X*reci_convert;%findtheinitialvaluemirrorofthesequcematrix
A=initialMIRROR'
[nrow,ncolumn]=size(A);
for(i=2:
nrow)
C=A(i,:
)—A(1,:
)
D=abs(C);
eval(['B’num2str(i)’=D']);
amax=max(eval([’B'num2str(i)]))
amin=min(eval([’B'num2str(i)]))
maxarray(i—1)=amax
minarray(i-1)=amin
end%findthedifferencesquenceandthemaxvalueandminvalueofeachsquence
maxmax=max(maxarray)
minmin=min(minarray)
for(i=2:
nrow)
for(j=1:
ncolumn)
eval([’greyrelationdegree’num2str(i)'(j)=(minmin+c*maxmax)/(B’num2str(i)'(j)+c*maxmax)'])
end
end%calculatethegreyralationaldegreeofeachdata
for(i=2:
nrow)
eval(['greyrelatioanaldegree_value’num2str(i)’=mean(greyrelationdegree'num2str(i)')’ ])
end
基于matlab灰色关联度计算的实现
2006年07月28日星期五上午11:
06
functionr=incident_degree(x0,x1)
%computetheincidentdegreeforgreymodel.
%DesignedbyNIXIUHUI,DalianFisherUniversity。
%17August,2004,LastmodifiedbyNXHat21August,2004
%数据初值化处理
x0_initial=x0./x0
(1);
temp=size(x1);
b=repmat(x1(:
1),[1temp
(2)]);
x1_initial=x1。
/b;
%分辨系数选择
K=0.1;
disp(’Thegreyinterconnectdegreeis:
');
x0_ext=repmat(x0_initial,[temp
(1)1]);
contrast_mat=abs(x0_ext—x1_initial);
delta_min=min(min(contrast_mat));%delta_min在数据初值化后实际为零
delta_max=max(max(contrast_mat));
a=delta_min+K*delta_max;
incidence_coefficient=a。
/(contrast_mat+K*delta_max);%得到关联系数
r=(sum(incidence_coefficient’))’/temp
(2); %得到邓氏面积关联度
我们根据图1的步骤和图2的数据进行编程实现,程序如下:
%清除内存空间等
clear;
closeall;
clc;
%载入源数据 %其实这里可以载入execl表格的
n=15; %参与评价的人数
m=4; %参与评价的指标个数
X_0=zeros(n,m); % 数据矩阵
X_2=zeros(n,m); %偏差结果的求取矩阵
X_3=zeros(n,m); % 相关系数计算矩阵
a1_0=[131817181717181713171813181318];
a2_0=[181817171813171318131713131717];
a3_0=[48.6743。
3343.5641。
8939。
4743.4437。
9741。
1439.6739。
8334。
1140.5834.1930.7521.22];
a4_0=[1010。
735.45。
40。
74。
20.59.30。
852。
95.454。
22。
76]; %指标数
X_1=[a1_0’,a2_0’,a3_0’,a4_0’]; %最后使用到的数据矩阵
%1寻找参考列
x0=[max(a1_0),max(a2_0),max(a3_0),max(a4_0)]; %取每列的最大值(指标的最大值)
%2计算偏差结果
i=1;
while(i~=m+1) %为什么这个地方会出问