协同过滤外文文献翻译.docx
《协同过滤外文文献翻译.docx》由会员分享,可在线阅读,更多相关《协同过滤外文文献翻译.docx(12页珍藏版)》请在冰豆网上搜索。
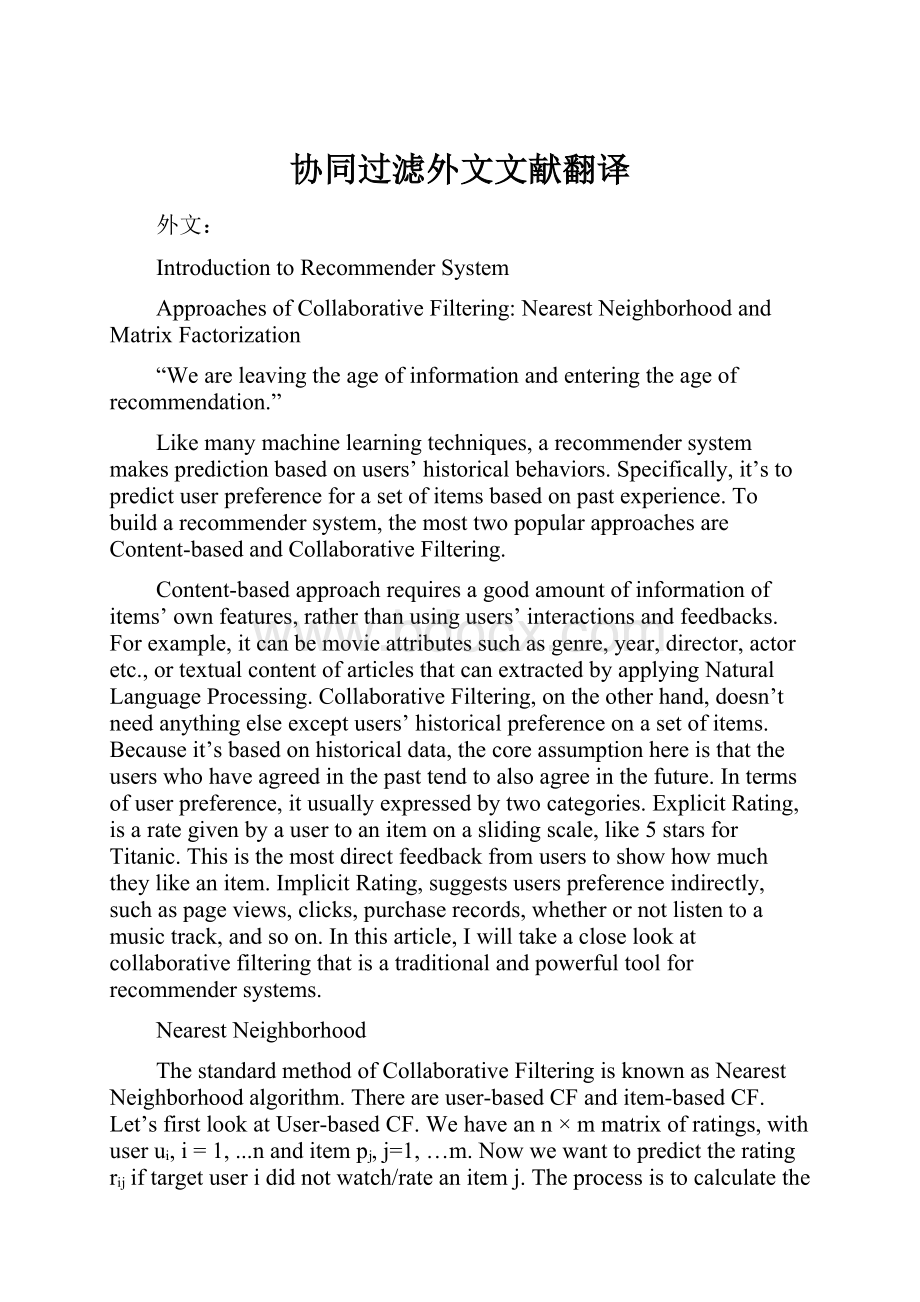
协同过滤外文文献翻译
外文:
IntroductiontoRecommenderSystem
ApproachesofCollaborativeFiltering:
NearestNeighborhoodandMatrixFactorization
“Weareleavingtheageofinformationandenteringtheageofrecommendation.”
Likemanymachinelearningtechniques,arecommendersystemmakespredictionbasedonusers’historicalbehaviors.Specifically,it’stopredictuserpreferenceforasetofitemsbasedonpastexperience.Tobuildarecommendersystem,themosttwopopularapproachesareContent-basedandCollaborativeFiltering.
Content-based approachrequiresagoodamountofinformationofitems’ownfeatures,ratherthanusingusers’interactionsandfeedbacks.Forexample,itcanbemovieattributessuchasgenre,year,director,actoretc.,ortextualcontentofarticlesthatcanextractedbyapplyingNaturalLanguageProcessing. CollaborativeFiltering,ontheotherhand,doesn’tneedanythingelseexceptusers’historicalpreferenceonasetofitems.Becauseit’sbasedonhistoricaldata,thecoreassumptionhereisthattheuserswhohaveagreedinthepasttendtoalsoagreeinthefuture.Intermsofuserpreference,itusuallyexpressedbytwocategories. ExplicitRating,isarategivenbyausertoanitemonaslidingscale,like5starsforTitanic.Thisisthemostdirectfeedbackfromuserstoshowhowmuchtheylikeanitem. ImplicitRating,suggestsuserspreferenceindirectly,suchaspageviews,clicks,purchaserecords,whetherornotlistentoamusictrack,andsoon.Inthisarticle,Iwilltakeacloselookatcollaborativefilteringthatisatraditionalandpowerfultoolforrecommendersystems.
NearestNeighborhood
ThestandardmethodofCollaborativeFilteringisknownas NearestNeighborhood algorithm.Thereareuser-basedCFanditem-basedCF.Let’sfirstlookat User-basedCF.Wehaveann×mmatrixofratings,withuseruᵢ,i=1,...nanditempⱼ,j=1,…m.Nowwewanttopredicttheratingrᵢⱼiftargetuserididnotwatch/rateanitemj.Theprocessistocalculatethesimilaritiesbetweentargetuseriandallotherusers,selectthetopXsimilarusers,andtaketheweightedaverageofratingsfromtheseXuserswithsimilaritiesasweights.
Whiledifferentpeoplemayhavedifferentbaselineswhengivingratings,somepeopletendtogivehighscoresgenerally,someareprettystricteventhoughtheyaresatisfiedwithitems.Toavoidthisbias,wecansubtracteachuser’saverageratingofallitemswhencomputingweightedaverage,andadditbackfortargetuser,shownasbelow.
Twowaystocalculatesimilarityare PearsonCorrelation and CosineSimilarity.
Basically,theideaistofindthemostsimilaruserstoyourtargetuser(nearestneighbors)andweighttheirratingsofanitemasthepredictionoftheratingofthisitemfortargetuser.
Withoutknowinganythingaboutitemsandusersthemselves,wethinktwousersaresimilarwhentheygivethesameitemsimilarratings.Analogously,for Item-basedCF,wesaytwoitemsaresimilarwhentheyreceivedsimilarratingsfromasameuser.Then,wewillmakepredictionforatargetuseronanitembycalculatingweightedaverageofratingsonmostXsimilaritemsfromthisuser.OnekeyadvantageofItem-basedCFisthestabilitywhichisthattheratingsonagivenitemwillnotchangesignificantlyovertime,unlikethetastesofhumanbeings.
Therearequiteafewlimitationsofthismethod.Itdoesn’thandlesparsitywellwhennooneintheneighborhoodratedanitemthatiswhatyouaretryingtopredictfortargetuser.Also,it’snotcomputationalefficientasthegrowthofthenumberofusersandproducts.
MatrixFactorization
SincesparsityandscalabilityarethetwobiggestchallengesforstandardCFmethod,itcomesamoreadvancedmethodthatdecomposetheoriginalsparsematrixtolow-dimensionalmatriceswithlatentfactors/featuresandlesssparsity.ThatisMatrixFactorization.
Besidesolvingtheissuesofsparsityandscalability,there’sanintuitiveexplanationofwhyweneedlow-dimensionalmatricestorepresentusers’preference.AusergavegoodratingstomovieAvatar,Gravity,andInception.Theyarenotnecessarily3separateopinionsbutshowingthatthisusersmightbeinfavorofSci-FimoviesandtheremaybemanymoreSci-Fimoviesthatthisuserwouldlike.Unlikespecificmovies,latentfeaturesisexpressedbyhigher-levelattributes,andSci-Ficategoryisoneoflatentfeaturesinthiscase. Whatmatrixfactorizationeventuallygivesusishowmuchauserisalignedwithasetoflatentfeatures,andhowmuchamoviefitsintothissetoflatentfeatures. Theadvantageofitoverstandardnearestneighborhoodisthateventhoughtwousershaven’tratedanysamemovies,it’sstillpossibletofindthesimilaritybetweenthemiftheysharethesimilarunderlyingtastes,againlatentfeatures.
Toseehowamatrixbeingfactorized,firstthingtounderstandis SingularValueDecomposition(SVD).BasedonLinearAlgebra,anyrealmatrixRcanbedecomposedinto3matricesU,Σ,andV.Continuingusingmovieexample,Uisann×ruser-latentfeaturematrix,Visanm×rmovie-latentfeaturematrix.Σisanr×rdiagonalmatrixcontainingthesingularvaluesoforiginalmatrix,simplyrepresentinghowimportantaspecificfeatureistopredictuserpreference.
TosortthevaluesofΣbydecreasingabsolutevalueandtruncatematrixΣtofirstkdimensions(ksingularvalues),wecanreconstructthematrixasmatrixA.TheselectionofkshouldmakesurethatAisabletocapturethemostofvariancewithintheoriginalmatrixR,sothatAistheapproximationofR,A≈R.ThedifferencebetweenAandRistheerrorthatisexpectedtobeminimized.ThisisexactlythethoughtofPrincipleComponentAnalysis.
WhenmatrixRisdense,UandVcouldbeeasilyfactorizedanalytically.However,amatrixofmovieratingsissupersparse.Althoughtherearesomeimputationmethodstofillinmissingvalues,wewillturntoaprogrammingapproachtojustlivewiththosemissingvaluesandfindfactormatricesUandV.InsteadoffactorizingRviaSVD,wearetryingfindUandVdirectlywiththegoalthatwhenUandVmultipliedbacktogethertheoutputmatrixR’istheclosestapproximationofRandnomoreasparsematrix.Thisnumericalapproximationisusuallyachievedwith Non-NegativeMatrixFactorization forrecommendersystemssincethereisnonegativevaluesinratings.
Seetheformulabelow.Lookingatthepredictedratingforspecificuseranditem,itemiisnotedasavectorqᵢ,anduseruisnotedasavectorpᵤsuchthatthedotproductofthesetwovectorsisthepredictedratingforuseruonitemi.ThisvalueispresentedinthematrixR’atrowuandcolumni.
Howdowefindoptimalqᵢandpᵤ?
Likemostofmachinelearningtask,alossfunctionisdefinedtominimizethecostoferrors.
rᵤᵢisthetrueratingsfromoriginaluser-itemmatrix.OptimizationprocessistofindtheoptimalmatrixPcomposedbyvectorpᵤandmatrixQcomposedbyvectorqᵢinordertominimizethesumsquareerrorbetweenpredictedratingsrᵤᵢ’andthetrueratingsrᵤᵢ.Also,L2regularizationhasbeenaddedtopreventoverfittingofuseranditemvectors.It’salsoquitecommontoaddbiastermwhichusuallyhas3majorcomponents:
averageratingofallitemsμ,averageratingofitemiminusμ(notedasbᵤ),averageratinggivenbyuseruminusu(notedasbᵢ).
Optimization
AfewoptimizationalgorithmshavebeenpopulartosolveNon-NegativeFactorization. AlternativeLeastSquare isoneofthem.Sincethelossfunctionisnon-convexinthiscase,there’snowaytoreachaglobalminimum,whileitstillcanreachagreatapproximationbyfindinglocalminimums.AlternativeLeastSquareistoholduserfactormatrixconstant,adjustitemfactormatrixbytakingderivativesoflossfunctionandsettingitequalto0,andthensetitemfactormatrixconstantwhileadjustinguserfactormatrix.Repeattheprocessbyswitchingandadjustingmatricesbackandforthuntilconvergence.IfyouapplyScikit-learnNMFmodel,youwillseeALSisthedefaultsolvertouse,whichisalsocalledCoordinateDescent.PysparkalsooffersprettyneatdecompositionpackagesthatprovidesmoretuningflexibilityofALSitself.
SomeThoughts
CollaborativeFilteringprovidesstrongpredictivepowerforrecommendersystems,andrequirestheleastinformationatthesametime.However,ithasafewlimitationsinsomeparticularsituations.
First,theunderlyingtastesexpressedbylatentfeaturesareactuallynotinterpretablebecausethereisnocontent-relatedpropertiesofmetadata.Formovieexample,itdoesn’tnecessarilytobegenrelikeSci-Fiinmyexample.Itcanbehowmotivationalthesoundtrackis,howgoodtheplotis,andsoon.CollaborativeFilteringislackoftransparencyandexplainabilityofthislevelofinformation.
Ontheotherhand,CollaborativeFilteringisfacedwithcoldstart.Whenanewitemcomingin,untilithastoberatedbysubstantialnumberofusers,themodelisnotabletomakeanypersonalizedrecommendations.Similarly,foritemsfromthetailthatdidn’tgettoomuchdata,themodeltendstogivelessweightonthemandhavepopularitybiasbyrecommendingmorepopularitems.
It’susuallyagoodideatohaveensemblealgorithmstobuildamorecomprehensivemachinelearningmodelsuchascombiningcontent-basedfilteringbyaddingsomedimensionsofkeywordsthatareexplainable,butweshouldalwaysconsiderthetradeoffbetweenmodel/computationalcomplexityandtheeffectivenessofperformanceimprovement.
中文翻译
推荐系统介绍
协同过滤的方法:
最近邻域和矩阵分解
“我们正在离开信息时代,而进入推荐时代。
”
像许多机器学习技术一样,推荐系统根据用户的历史行为进行预测。
具体来说,是根据过去的经验来预测用户对一组商品的偏好。
要构建推荐系统,最流行的两种方法是基于内容的过滤和协作过滤。
基于内容的方法需要大量项目自身功能的信息,而不是使用用户的交互和反馈。
例如,它可以是电影属性(例如流派,年份,导演,演员等)或可以通过应用自然语言