车牌识别外文翻译讲解学习.docx
《车牌识别外文翻译讲解学习.docx》由会员分享,可在线阅读,更多相关《车牌识别外文翻译讲解学习.docx(10页珍藏版)》请在冰豆网上搜索。
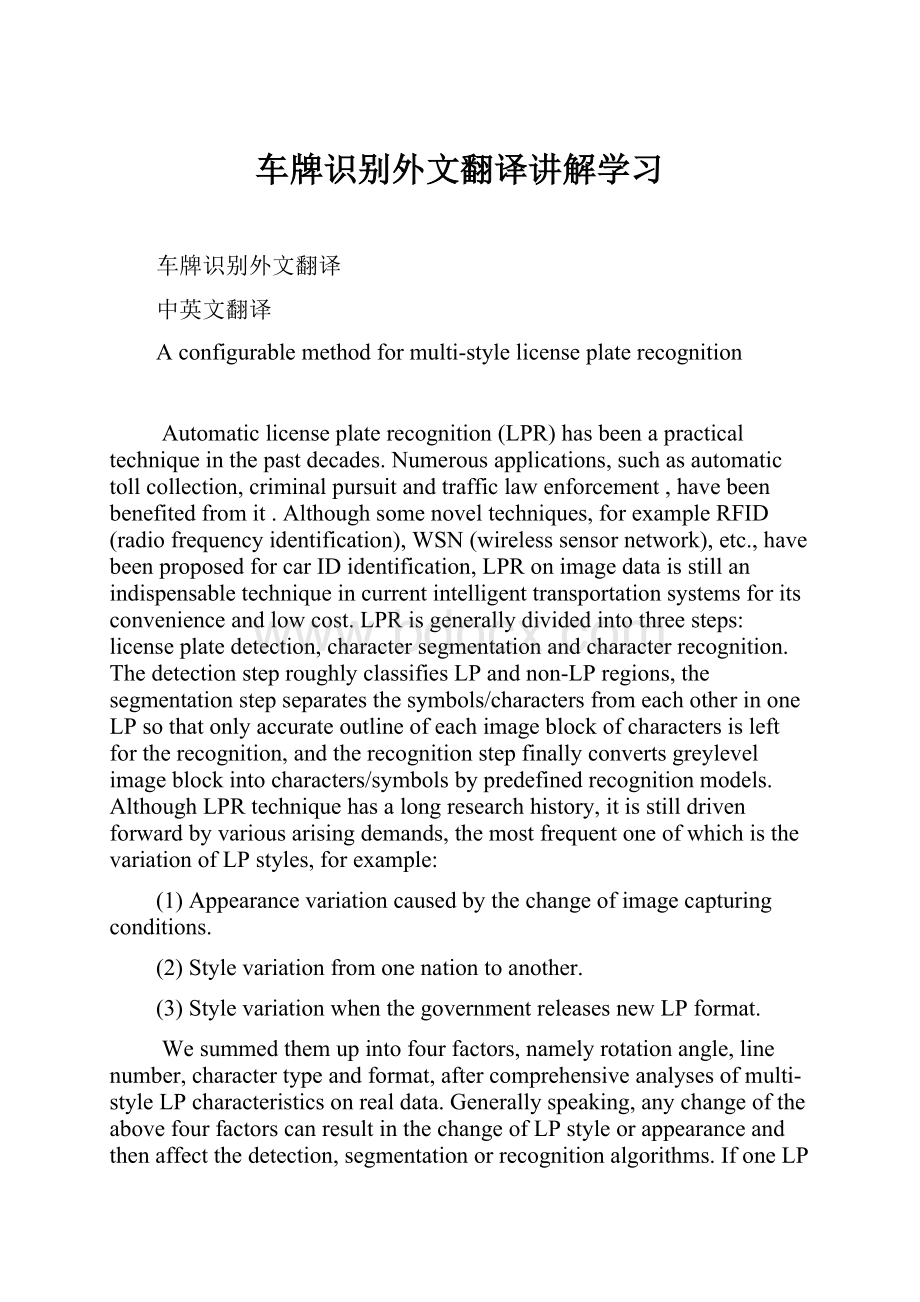
车牌识别外文翻译讲解学习
车牌识别外文翻译
中英文翻译
Aconfigurablemethodformulti-stylelicenseplaterecognition
Automaticlicenseplaterecognition(LPR)hasbeenapracticaltechniqueinthepastdecades.Numerousapplications,suchasautomatictollcollection,criminalpursuitandtrafficlawenforcement,havebeenbenefitedfromit.Althoughsomenoveltechniques,forexampleRFID(radiofrequencyidentification),WSN(wirelesssensornetwork),etc.,havebeenproposedforcarIDidentification,LPRonimagedataisstillanindispensabletechniqueincurrentintelligenttransportationsystemsforitsconvenienceandlowcost.LPRisgenerallydividedintothreesteps:
licenseplatedetection,charactersegmentationandcharacterrecognition.ThedetectionsteproughlyclassifiesLPandnon-LPregions,thesegmentationstepseparatesthesymbols/charactersfromeachotherinoneLPsothatonlyaccurateoutlineofeachimageblockofcharactersisleftfortherecognition,andtherecognitionstepfinallyconvertsgreylevelimageblockintocharacters/symbolsbypredefinedrecognitionmodels.AlthoughLPRtechniquehasalongresearchhistory,itisstilldrivenforwardbyvariousarisingdemands,themostfrequentoneofwhichisthevariationofLPstyles,forexample:
(1)Appearancevariationcausedbythechangeofimagecapturingconditions.
(2)Stylevariationfromonenationtoanother.
(3)StylevariationwhenthegovernmentreleasesnewLPformat.
Wesummedthemupintofourfactors,namelyrotationangle,linenumber,charactertypeandformat,aftercomprehensiveanalysesofmulti-styleLPcharacteristicsonrealdata.Generallyspeaking,anychangeoftheabovefourfactorscanresultinthechangeofLPstyleorappearanceandthenaffectthedetection,segmentationorrecognitionalgorithms.IfoneLPhasalargerotationangle,thesegmentationandrecognitionalgorithmsforhorizontalLPmaynotwork.IftherearemorethanonecharacterlinesinoneLP,additionallineseparationalgorithmisneededbeforeasegmentationprocess.Withthevariationofcharactertypeswhenweapplythemethodfromonenationtoanother,theabilitytore-definetherecognitionmodelsisneeded.Whatismore,thechangeofLPstylesrequiresthemethodtoadjustbyitselfsothatthesegmentedandrecognizedcharactercandidatescanmatchbestwithanLPformat.
Severalmethodshavebeenproposedformulti-nationalLPsormultiformatLPsinthepastyearswhilefewofthemcomprehensivelyaddressthestyleadaptationproblemintermsoftheabovementionedfactors.SomeofthemonlyclaimtheabilityofprocessingmultinationalLPsbyredefiningthedetectionandsegmentationrulesorrecognitionmodels.
Inthispaper,weproposeaconfigurableLPRmethodwhichisadaptablefromonestyletoanother,particularlyfromonenationtoanother,bydefiningthefourfactorsasparameters.Userscanconstrainthescopeofaparameterandatthesametimethemethodwilladjustitselfsothattherecognitioncanbefasterandmoreaccurate.SimilartoexistingLPRtechniques,wealsoprovidedetailsofdetection,segmentationandrecognitionalgorithms.ThedifferenceisthatweemphasizeontheconfigurableframeworkforLPRandtheextensibilityoftheproposedmethodformultistyleLPsinsteadoftheperformanceofeachalgorithm.
Inthepastdecades,manymethodshavebeenproposedforLPRthatcontainsdetection,segmentationandrecognitionalgorithms.Inthefollowingparagraphs,thesealgorithmsandLPRmethodsbasedonthemarebrieflyreviewed.
LPdetectionalgorithmscanbemainlyclassifiedintothreeclassesaccordingtothefeaturesused,namelyedgebasedalgorithms,colorbasedalgorithmsandtexture-basedalgorithms.ThemostcommonlyusedmethodforLPdetectioniscertainlythecombinationsofedgedetectionandmathematicalmorphology.Inthesemethods,gradient(edges)isfirstextractedfromtheimageandthenaspatialanalysisbymorphologyisappliedtoconnecttheedgesintoLPregions.AnotherwayiscountingedgesontheimagerowstofindoutregionsofdenseedgesortodescribethedenseedgesinLPregionsbyaHoughtransformation.Edgeanalysisisthemoststraightforwardmethodwithlowcomputationcomplexityandgoodextensibility.Comparedwithedgebasedalgorithms,colorbasedalgorithmsdependmoreontheapplicationconditions.SinceLPsinanationoftenhaveseveralpredefinedcolors,researchershavedefinedcolormodelstosegmentregionofinterestsastheLPregions.Thiskindofmethodcanbeaffectedalotbylightingconditions.Towinbothhighrecallandlowfalsepositiverates,textureclassificationhasbeenusedforLPdetection.InRef.Kimetal.usedanSVMtotraintextureclassifierstodetectimageblockthatcontainsLPpixels.InRef.theauthorsusedGaborfilterstoextracttexturefeaturesinmultiscalesandmultiorientationstodescribethetexturepropertiesofLPregions.InRef.ZhangusedXandYderivativefeatures,grey-valuevarianceandAdaboostclassifiertoclassifyLPandnon-LPregionsinanimage.InRefs.waveletfeatureanalysisisappliedtoidentifyLPregions.Despitethegoodperformanceofthesemethodsthecomputationcomplexitywilllimittheirusability.Inaddition,texture-basedalgorithmsmaybeaffectedbymulti-lingualfactors.
Multi-lineLPsegmentationalgorithmscanalsobeclassifiedintothreeclasses,namelyalgorithmsbasedonprojection,binarizationandglobaloptimization.Intheprojectionalgorithms,gradientorcolorprojectiononverticalorientationwillbecalculatedatfirst.The“valleys”ontheprojectionresultareregardedasthespacebetweencharactersandusedtosegmentcharactersfromeachother.SegmentedregionsarefurtherprocessedbyverticalprojectiontoobtainpreciseboundingboxesoftheLPcharacters.SincesimplesegmentationmethodsareeasilyaffectedbytherotationofLP,segmentingtheskewedLPbecomesakeyissuetobesolved.Inthebinarizationalgorithms,globalorlocalmethodsareoftenusedtoobtainforegroundfrombackgroundandthenregionconnectionoperationisusedtoobtaincharacterregions.Inthemostrecentwork,localthresholddeterminationandslidewindowtechniquearedevelopedtoimprovethesegmentationperformance.Intheglobaloptimizationalgorithms,thegoalisnottoobtaingoodsegmentationresultforindependentcharactersbuttoobtainacompromiseofcharacterspatialarrangementandsinglecharacterrecognitionresult.HiddenMarkovchainhasbeenusedtoformulatethedynamicsegmentationofcharactersinLP.Theadvantageofthealgorithmisthattheglobaloptimizationwillimprovetherobustnesstonoise.Andthedisadvantageisthatpreciseformatdefinitionisnecessarybeforeasegmentationprocess.
CharacterandsymbolrecognitionalgorithmsinLPRcanbecategorizedintolearning-basedonesandtemplatematchingones.Fortheformerone,artificialneuralnetwork(ANN)isthemostlyusedmethodsinceitisprovedtobeabletoobtainverygoodrecognitionresultgivenalargetrainingset.AnimportantfactorintraininganANNrecognitionmodelforLPistobuildreasonablenetworkstructurewithgoodfeatures.SVM-basedmethodisalsoadoptedinLPRtoobtaingoodrecognitionperformancewithevenfewtrainingsamples.Recently,cascadeclassifiermethodisalsousedforLPrecognition.Templatematchingisanotherwidelyusedalgorithm.Generally,researchersneedtobuildtemplateimagesbyhandfortheLPcharactersandsymbols.Theycanassignlargerweightsfortheimportantpoints,forexample,thecornerpoints,inthetemplatetoemphasizethedifferentcharacteristicsofthecharacters.Invarianceoffeaturepointsisalsoconsideredinthetemplatematchingmethodtoimprovetherobustness.Thedisadvantageisthatitisdifficulttodefinenewtemplatebytheuserswhohavenoprofessionalknowledgeonpatternrecognition,whichwillrestricttheapplicationofthealgorithm.
Basedontheabovementionedalgorithms,lotsofLPRmethodshavebeendeveloped.However,thesemethodsaremainlydevelopedforspecificnationorspecialLPformats.InRef.theauthorsfocusonrecognizingGreekLPsbyproposingnewsegmentationandrecognitionalgorithms.ThecharactersonLPsarealphanumericswithseveralfixedformats.InRef.Zhangetal.developedalearning-basedmethodforLPdetectionandcharacterrecognition.TheirmethodismainlyforLPsofKoreanstyles.InRef.opticalcharacterrecognition(OCR)techniqueareintegratedintoLPRtodevelopgeneralLPRmethod,whiletheperformanceofOCRmaydropwhenfacingLPsofpoorimagequalitysinceitisdifficulttodiscriminaterealcharacterfromcandidateswithoutformatsupervision.ThismethodcanonlyselectcandidatesofbestrecognitionresultsasLPcharacterswithoutrecoveryprocess.Wangetal.developedamethodtorecognizeLPRwithvariousviewingangles.Skewfactorisconsideredintheirmethod.InRef.theauthorsproposedanautomaticLPRmethodwhichcantreatthecasesofchangesofillumination,vehiclespeed,routesandbackgrounds,whichwasrealizedbydevelopingnewdetectionandsegmentationalgorithmswithrobustnesstotheilluminationandimageblurring.Theperformanceofthemethodisencouragingwhiletheauthorsdonotpresenttherecognitionresultinmultinationormultistyleconditions.InRef.theauthorsproposeanLPRmethodinmultinationalenvironmentwithcharactersegmentationandformatindependentrecognition.Sincenorecognitioninformationisusedincharactersegmentation,falsesegmentedcharactersfrombackgroundnoisemaybeproduced.Whatismore,therecognitionmethodisnotalearning-basedmethod,whichwilllimititsextensibility.InRef.Mecoccietal.proposeagenerativerecognitionmethod.Generati