数据库聚类分析外文翻译文献.docx
《数据库聚类分析外文翻译文献.docx》由会员分享,可在线阅读,更多相关《数据库聚类分析外文翻译文献.docx(13页珍藏版)》请在冰豆网上搜索。
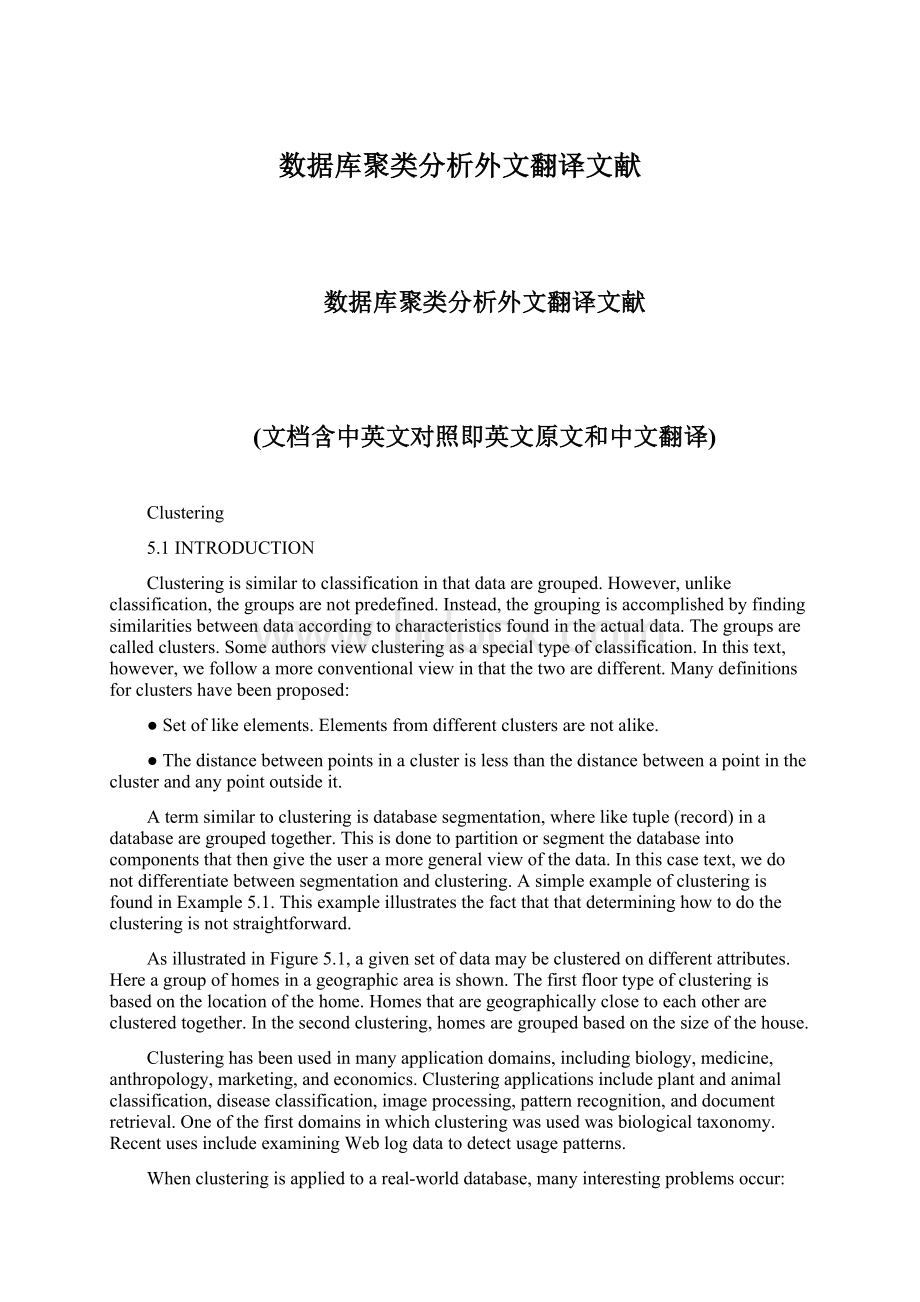
数据库聚类分析外文翻译文献
数据库聚类分析外文翻译文献
(文档含中英文对照即英文原文和中文翻译)
Clustering
5.1INTRODUCTION
Clusteringissimilartoclassificationinthatdataaregrouped.However,unlikeclassification,thegroupsarenotpredefined.Instead,thegroupingisaccomplishedbyfindingsimilaritiesbetweendataaccordingtocharacteristicsfoundintheactualdata.Thegroupsarecalledclusters.Someauthorsviewclusteringasaspecialtypeofclassification.Inthistext,however,wefollowamoreconventionalviewinthatthetwoaredifferent.Manydefinitionsforclustershavebeenproposed:
●Setoflikeelements.Elementsfromdifferentclustersarenotalike.
●Thedistancebetweenpointsinaclusterislessthanthedistancebetweenapointintheclusterandanypointoutsideit.
Atermsimilartoclusteringisdatabasesegmentation,whereliketuple(record)inadatabasearegroupedtogether.Thisisdonetopartitionorsegmentthedatabaseintocomponentsthatthengivetheuseramoregeneralviewofthedata.Inthiscasetext,wedonotdifferentiatebetweensegmentationandclustering.AsimpleexampleofclusteringisfoundinExample5.1.Thisexampleillustratesthefactthatthatdetermininghowtodotheclusteringisnotstraightforward.
AsillustratedinFigure5.1,agivensetofdatamaybeclusteredondifferentattributes.Hereagroupofhomesinageographicareaisshown.Thefirstfloortypeofclusteringisbasedonthelocationofthehome.Homesthataregeographicallyclosetoeachotherareclusteredtogether.Inthesecondclustering,homesaregroupedbasedonthesizeofthehouse.
Clusteringhasbeenusedinmanyapplicationdomains,includingbiology,medicine,anthropology,marketing,andeconomics.Clusteringapplicationsincludeplantandanimalclassification,diseaseclassification,imageprocessing,patternrecognition,anddocumentretrieval.Oneofthefirstdomainsinwhichclusteringwasusedwasbiologicaltaxonomy.RecentusesincludeexaminingWeblogdatatodetectusagepatterns.
Whenclusteringisappliedtoareal-worlddatabase,manyinterestingproblemsoccur:
●Outlierhandlingisdifficult.Heretheelementsdonotnaturallyfallintoanycluster.Theycanbeviewedassolitaryclusters.However,ifaclusteringalgorithmattemptstofindlargerclusters,theseoutlierswillbeforcedtobeplacedinsomecluster.Thisprocessmayresultinthecreationofpoorclustersbycombiningtwoexistingclustersandleavingtheoutlierinitsowncluster.
●Dynamicdatainthedatabaseimpliesthatclustermembershipmaychangeovertime.
●Interpretingthesemanticmeaningofeachclustermaybedifficult.Withclassification,thelabelingoftheclassesisknownaheadoftime.However,withclustering,thismaynotbethecase.Thus,whentheclusteringprocessfinishescreatingasetofclusters,theexactmeaningofeachclustermaynotbeobvious.Hereiswhereadomainexpertisneededtoassignalabelorinterpretationforeachcluster.
●Thereisnoonecorrectanswertoaclusteringproblem.Infact,manyanswersmaybefound.Theexactnumberofclustersrequiredisnoteasytodetermine.Again,adomainexpertmayberequired.Forexample,supposewehaveasetofdataaboutplantsthathavebeencollectedduringafieldtrip.Withoutanypriorknowledgeofplantclassification,ifweattempttodividethissetofdataintosimilargroupings,itwouldnotbeclearhowmanygroupsshouldbecreated.
●Anotherrelatedissueiswhatdatashouldbeusedofclustering.Unlikelearningduringaclassificationprocess,wherethereissomeaprioriknowledgeconcerningwhattheattributesofeachclassificationshouldbe,inclusteringwehavenosupervisedlearningtoaidtheprocess.Indeed,clusteringcanbeviewedassimilartounsupervisedlearning.
Wecanthensummarizesomebasicfeaturesofclustering(asopposedtoclassification):
●The(best)numberofclustersisnotknown.
●Theremaynotbeanyaprioriknowledgeconcerningtheclusters.
●Clusterresultsaredynamic.
TheclusteringproblemisstatedasshowninDefinition5.1.Hereweassumethatthenumberofclusterstobecreatedisaninputvalue,k.Theactualcontent(andinterpretation)ofeachcluster,
isdeterminedasaresultofthefunctiondefinition.Withoutlossofgenerality,wewillviewthattheresultofsolvingaclusteringproblemisthatasetofclustersiscreated:
K={
}.
DEFINITION5.1.GivenadatabaseD={
}oftuplesandanintegervaluek,theclusteringproblemistodefineamappingf:
whereeach
isassignedtoonecluster
.Acluster
containspreciselythosetuplesmappedtoit;thatis,
={
and
}.
AclassificationofthedifferenttypesofclusteringalgorithmsisshowninFigure5.2.Clusteringalgorithmsthemselvesmaybeviewedashierarchicalorpartitional.Withhierarchicalclustering,anestedsetofclustersiscreated.Eachlevelinthehierarchyhasaseparatesetofclusters.Atthelowestlevel,eachitemisinitsownuniquecluster.Atthehighestlevel,allitemsbelongtothesamecluster.Withhierarchicalclustering,thedesirednumberofclustersisnotinput.Withpartitionalclustering,thealgorithmcreatesonlyonesetofclusters.Theseapproachesusethedesirednumberofclusterstodrivehowthefinalsetiscreated.Traditionalclusteringalgorithmstendtobetargetedtosmallnumericdatabasethatfitintomemory.Thereare,however,morerecentclusteringalgorithmsthatlookatcategoricaldataandaretargetedtolarger,perhapsdynamic,databases.Algorithmstargetedtolargerdatabasesmayadapttomemoryconstraintsbyeithersamplingthedatabaseorusingdatastructures,whichcanbecompressedorprunedtofitintomemoryregardlessofthesizeofthedatabase.Clusteringalgorithmsmayalsodifferbasedonwhethertheyproduceoverlappingornonoverlappingclusters.Eventhoughweconsideronlynonoverlappingclusters,itispossibletoplaceaniteminmultipleclusters.Inturn,nonoverlappingclusterscanbeviewedasextrinsicorintrinsic.Extrinsictechniquesuselabelingoftheitemstoassistintheclassificationprocess.Thesealgorithmsarethetraditionalclassificationsupervisedlearningalgorithmsinwhichaspecialinputtrainingsetisused.Intrinsicalgorithmsdonotuseanyaprioricategorylabels,butdependonlyontheadjacencymatrixcontainingthedistancebetweenobjects.Allalgorithmsweexamineinthischapterfallintotheintrinsicclass.
Thetypesofclusteringalgorithmscanbefurtheredclassifiedbasedontheimplementationtechniqueused.Hierarchicalalgorithmscanbecategorizedasagglomerativeordivisive.”Agglomerative”impliesthattheclustersarecreatedinabottom-upfashion,whiledivisivealgorithmsworkinatop-downfashion.Althoughbothhierarchicalandpartitionalalgorithmscouldbedescribedusingtheagglomerativevs.divisivelabel,ittypicallyismoreassociatedwithhierarchicalalgorithms.Anotherdescriptivetagindicateswhethereachindividualelementishandledonebyone,serial(sometimescalledincremental),orwhetherallitemsareexaminedtogether,simultaneous.Ifaspecifictupleisviewedashavingattributevaluesforallattributesintheschema,thenclusteringalgorithmscoulddifferastohowtheattributevaluesareexamined.Asisusuallydonewithdecisiontreeclassificationtechniques,somealgorithmsexamineattributevaluesoneatatime,monothetic.Polytheticalgorithmsconsiderallattributevaluesatonetime.Finally,clusteringalgorithmscanbelabeledbaseonthemathematicalformulationgiventothealgorithm:
graphtheoreticormatrixalgebra.Inthischapterwegenerallyusethegraphapproachanddescribetheinputtotheclusteringalgorithmasanadjacencymatrixlabeledwithdistancemeasure.
Wediscussmanyclusteringalgorithmsinthefollowingsections.Thisisonlyarepresentativesubsetofthemanyalgorithmsthathavebeenproposedintheliterature.Beforelookingatthesealgorithms,wefirstexaminepossiblesimilaritymeasuresandexaminetheimpactofoutliers.
5.2SIMILARITYANDDISTANCEMEASURES
Therearemanydesirablepropertiesfortheclusterscreatedbyasolutiontoaspecificclusteringproblem.Themostimportantoneisthatatuplewithinoneclusterismoreliketupleswithinthatclusterthanitissimilartotuplesoutsideit.Aswithclassification,then,weassumethedefinitionofasimilaritymeasure,sim(
),definedbetweenanytwotuples,
.Thisprovidesamorestrictandalternativeclusteringdefinition,asfoundinDefinition5.2.Unlessotherwisestated,weusethefirstdefinitionratherthanthesecond.Keepinmindthatthesimilarityrelationshipstatedwithintheseconddefinitionisadesirable,althoughnotalwaysobtainable,property.
Adistancemeasure,dis(
),asopposedtosimilarity,isoftenusedinclustering.Theclusteringproblemthenhasthedesirablepropertythatgivenacluster,
and
.
Someclusteringalgorithmslookonlyatnumericdata,usuallyassumingmetricdatapoints.Metricattributessatisfythetriangularinequality.Theclustercanthenbedescribedbyusingseveralcharacteristicvalues.Givenacluster,
ofNpoints{
},wemakethefollowingdefinitions[ZRL96]:
Herethecentroidisthe“middle”ofthecluster;itneednotbeanactualpointinthecluster.Someclusteringalgorithmsalternativelyassumethattheclusterisrepresentedbyonecentrallylocatedobjectintheclustercalledamedoid.Theradiusisthesquarerootoftheaveragemeansquareddistancefromanypointintheclustertothecentroid,andofpointsinthecluster.Weusethenotation
toindicatethemedoidforcluster
.
Manyclusteringalgorithmsrequirethatthedistancebetweenclusters(ratherthanelements)