多水平统计模型 第5章.docx
《多水平统计模型 第5章.docx》由会员分享,可在线阅读,更多相关《多水平统计模型 第5章.docx(15页珍藏版)》请在冰豆网上搜索。
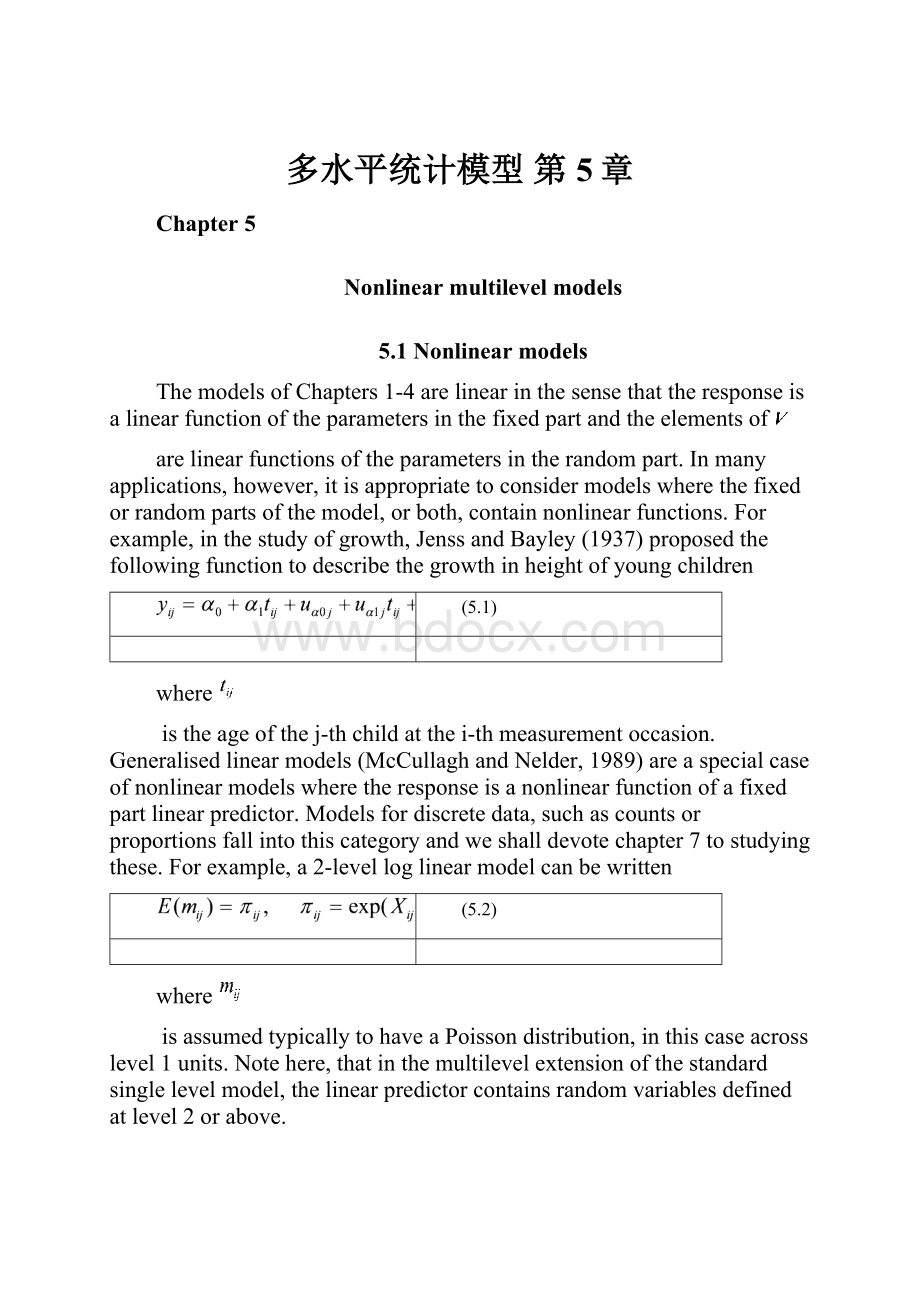
多水平统计模型第5章
Chapter5
Nonlinearmultilevelmodels
5.1Nonlinearmodels
ThemodelsofChapters1-4arelinearinthesensethattheresponseisalinearfunctionoftheparametersinthefixedpartandtheelementsof
arelinearfunctionsoftheparametersintherandompart.Inmanyapplications,however,itisappropriatetoconsidermodelswherethefixedorrandompartsofthemodel,orboth,containnonlinearfunctions.Forexample,inthestudyofgrowth,JenssandBayley(1937)proposedthefollowingfunctiontodescribethegrowthinheightofyoungchildren
(5.1)
where
istheageofthej-thchildatthei-thmeasurementoccasion.Generalisedlinearmodels(McCullaghandNelder,1989)areaspecialcaseofnonlinearmodelswheretheresponseisanonlinearfunctionofafixedpartlinearpredictor.Modelsfordiscretedata,suchascountsorproportionsfallintothiscategoryandweshalldevotechapter7tostudyingthese.Forexample,a2-levelloglinearmodelcanbewritten
(5.2)
where
isassumedtypicallytohaveaPoissondistribution,inthiscaseacrosslevel1units.Notehere,thatinthemultilevelextensionofthestandardsinglelevelmodel,thelinearpredictorcontainsrandomvariablesdefinedatlevel2orabove.
Inthischapterweconsiderageneralnonlinearmodel.Laterchapterswillusetheresultsforparticularapplications.
5.2Nonlinearfunctionsoflinearcomponents
ThefollowingresultsareanextensionofthosepresentedbyGoldstein(1991)andappendix5.1givesdetails.Wheretherandomvariablesarenotpartofthenonlinearfunction,theproceduregivesmaximumlikelihoodestimates(seeappendix5.1).Inthecasewherethelevel1variationisnonNormaltheprocedurecanberegardedasageneralisationofquasilikelihoodestimation(McCullaghandNelder,1989)andsuchmodelsarediscussedinchapter7.
Restrictingattentiontoa2-levelstructurewecanwriteafairlygeneralmodelasfollows
(5.3)
wherethefunction
isnonlinearandwherethe
indicatesthatadditionalnonlinearfunctionscanbeincluded,involvingfurtherfixedpartexplanatoryvariables
orrandompartexplanatoryvariablesatlevels1and2,respectively
.ThemodelisfirstlinearisedbyasuitableTaylorseriesexpansionandthisleadstoconsiderationofalinearmodelwheretheexplanatoryvariablesin
aretransformedusingfirstandsecondderivativesofthenonlinearfunction.Notethatthelinearcomponentof(5.3)istreatedinthestandardway,andthattherandomvariablesatagivenlevelinthelinearandnonlinearcomponentsmaybecorrelated.
Considerthenonlinearfunction
.Appendix5.1showsthatwecanwritethisasthesumofafixedpartcomponentandarandompart.TheTaylorexpansionfortherandompartuptoasecondorderapproximationfortheij-thunitisasfollows
(5.4)
Thefirsttermontherighthandsideisthefixedpartvalueof
atthecurrent((t+1)-th)iterationoftheIGLSorRIGLSalgorithm,thatisignoringtherandompart.Theothertwotermsinvolvethefirstandseconddifferentialsofthenonlinearfunctionevaluatedatthecurrentvaluesfromthepreviousiteration.Wehave
(5.5)
Wewritetheexpansionforthefixedpartvalueas
(5.6)
where
arethecurrentandpreviousiterationvaluesofthefixedpartcoefficients.
Wecanchoose
tobeeitherthecurrentvalueofthefixedpartpredictor,thatis
orwecanaddthecurrentestimatedresidualstoobtainanimprovedapproximationtothenonlinearcomponentforeachunit.Theformerisreferredtoasa'marginal'(quasilikelihood)modelandthelatterasa'penalised'or'predictive'(quasilikelihood)model(seeBreslowandClayton,1993,forafurtherdiscussion).Wecanalsochoosewhetherornottoincludethetermin(5.4)involvingthesecondderivativeandwewouldexpectitsinclusioningeneraltoimprovetheestimates.Itsinclusiondefinesafurtheroffsetforthefixedpartandonefortherandompart(seeappendix5.1).Weshallillustratetheeffectofthesechoicesintheexamplesgiveninchapter7.FurtherdetailsoftheestimationprocedurearegiveninAppendix5.1.Inpracticegeneralmodelssuchas(5.1)mayposeconsiderableestimationproblems.Wenoticethatthesameexplanatoryvariablesoccurinthelinearandnonlinearcomponentsandthiscanleadtoinstabilityandfailuretoconverge.Furtherworkinthisareaisrequired.
Table5.1givesexpressionsforthefirstandseconddifferentialsforsomecommonlyusednonlinearmodels.
Table5.1Differentialsforsomecommonnonlinearmodels.
Model
Function
Firstdifferential
Seconddifferential
loglinear
logit
log-log
inverse
5.3Estimatingpopulationmeans
Considertheexpectedvalueoftheresponseforagivensetofcovariatevalues.Becauseofthenonlinearitythisisnotingeneralequaltothepredictedvaluewhentherandomvariablesinthenonlinearfunctionarezero.Forexample,ifwewritethevariancecomponentsmodel(5.2)
andassumingNormalityfor
weobtain
Where
isthedensityfunctionoftheNormaldistribution.Zegeretal(1988)considerthisissueandproposea‘populationaverage’modelfordirectlyobtainingpopulationpredictedvaluesbyeliminatingrandomvariablesfromthenonlinearcomponent.Ingeneral,however,thisapproachislessefficientwhenthefullmodelwithrandomvariableswithinthenonlinearfunctionisthecorrectmodel.Thepopulationpredictedvalues,conditionaloncovariates,canbeobtainedifrequired,asabove,bytakingexpectationsoverthepopulation.Anapproximationtothiscanbeobtainedfromthesecondordertermsin(5.1.4)withhigherordertermsintroducedifnecessarytoobtainabetterapproximation.Alternativelywemaygeneratealargenumberofsimulatedsetsofvaluesfortherandomvariablesandforeachsetevaluatetheresponsefunctiontoobtainanestimateofthefullpopulationdistribution.
5.4Nonlinearfunctionsforvariancesandcovariances
Wesawinchapter3howwecouldmodelcomplexfunctionsofthelevel1variance.Aswiththelinearcomponentofthemodel,therearecaseswherewemaywishtomodelvariancesorcovariancesasnonlinearfunctions.Inprinciplewecandothisatanylevelbutwerestrictourattentiontolevel1andtothevarianceonly.Inchapter6wegiveanexamplewherethecovariancesaremodelledinthisway.
Supposethatthelevel1variancedecreaseswithincreasingvaluesofanexplanatoryvariablesuchthatitapproachesafixedvalueasymptotically.Wecouldthenmodelthisfora2-levelmodel,say,asfollows
where
areparameterstobeestimated.Suchamodelalsoguaranteesthatthelevel1varianceispositivewhichisnotthecasewithlinearmodels,suchasthosebasedonpolynomials.TheestimationprocedureisanalogoustothatdescribedaboveanddetailsaregiveninAppendix5.1.
5.5Examplesofnonlineargrowthandnonlinearlevel1variance
Wegivefirstanexampleofamodelwithanonlinearfunctionforthelinearcomponentandwethenconsiderthecaseofanonlinearlevel1variancefunction.
Weuseanexamplefromchildgrowth,consistingof577repeatedmeasurementsofheighton197FrenchCanadianboysagedfrom5to10years(Demirjianetal,1982)withbetween3and7measurementseach.Thisisa2-levelstructurewithmeasurementoccasionsnestedwithinchildren.WefitthefollowingversionoftheJenss-Bayleycurvetoillustratetheprocedure
(5.7)
sothatthefixedpartisaninterceptplusanonlinearcomponentandtherandompartvarianceatlevel2ispartofthenonlinearcomponent.Theresultsaregivenintable5.2,usingthefirstorderapproximationwithpredictionbaseduponthefixedpartonly.Weshallcomparetheperformanceofthedifferentapproximationsinchapter7.
Thelevel1varianceissmallandoftheorderofthemeasurementerrorofheightmeasurements.Thestartingvaluesforthismodelneedtobechosenwithcare,andinthepresentcasethemodelwasruntoconvergencewithoutthelinearintercept
whichwasthenaddedwithastartingvalueof100.Bock(1992)usesanEMalgorithmtofitanonlinear2-levelmodeltogrowthdatafromage2yearstoadulthoodusingamixtureofthreelogisticcurves.
ThesecondexampleusestheJSPdatasetwherewestudiedthelevel1varianceinchapter3.WewillfitmodelBofTable3.1withanonlinearfunctionofthelevel1varianceinsteadofthelevel1varianceasaquadraticfunctionofthe8-year-score.Thislevel1variancefortheij-thlevel1unitis
andtable5.3showsthemodelestimates.
TheestimatesarealmostidenticaltothoseofmodelBoftable3.1asisthelikelihoodvalue.
Figure5.1showsthepredictedlevel1varianceforthismodelandmodelBofTable3.1.
Fig5.1Level1varianceasafunctionof8-yearMathsscore
Table5.2Nonlinearmodelestimateswithfirstorderfixedpartprediction.Ageismeasuredabout8.0years.
Fixedcoefficient
Estimate(s.e.)
Intercept(linear)
90.3
Intercept(nonlinear)
3.58
Age
0.15(0.10)
Agesquared
-0.016(0.02)
Agecubed
0.002(0.004)
Nonlinearmodellevel2covariancematrix(s.e.)
Intercept
Age
Intercept
0.025(0.003)
Age
-0.0027(0.0003)
0.00036(0.00005)
Level1variance=0.25
Inthesedatathenonlinearfunctiongivesverysimilarresultstothequadraticone.Itisclear,however,thatwherethevarianceasymptoticallyapproachesaconstantvalue,forextremevaluesofanexplanatoryvariable,alinearorevenquadraticapproximationmaybeexpectedtofail.Inthepresentcasealinearfunctiondoespredictanegativelevel1variancewithintherangeofthedata.Anexamplewhereanonlinearfunctionisnecessar