A clustering guided ant colony optimization algorithmservice selection problemWord下载.docx
《A clustering guided ant colony optimization algorithmservice selection problemWord下载.docx》由会员分享,可在线阅读,更多相关《A clustering guided ant colony optimization algorithmservice selection problemWord下载.docx(14页珍藏版)》请在冰豆网上搜索。
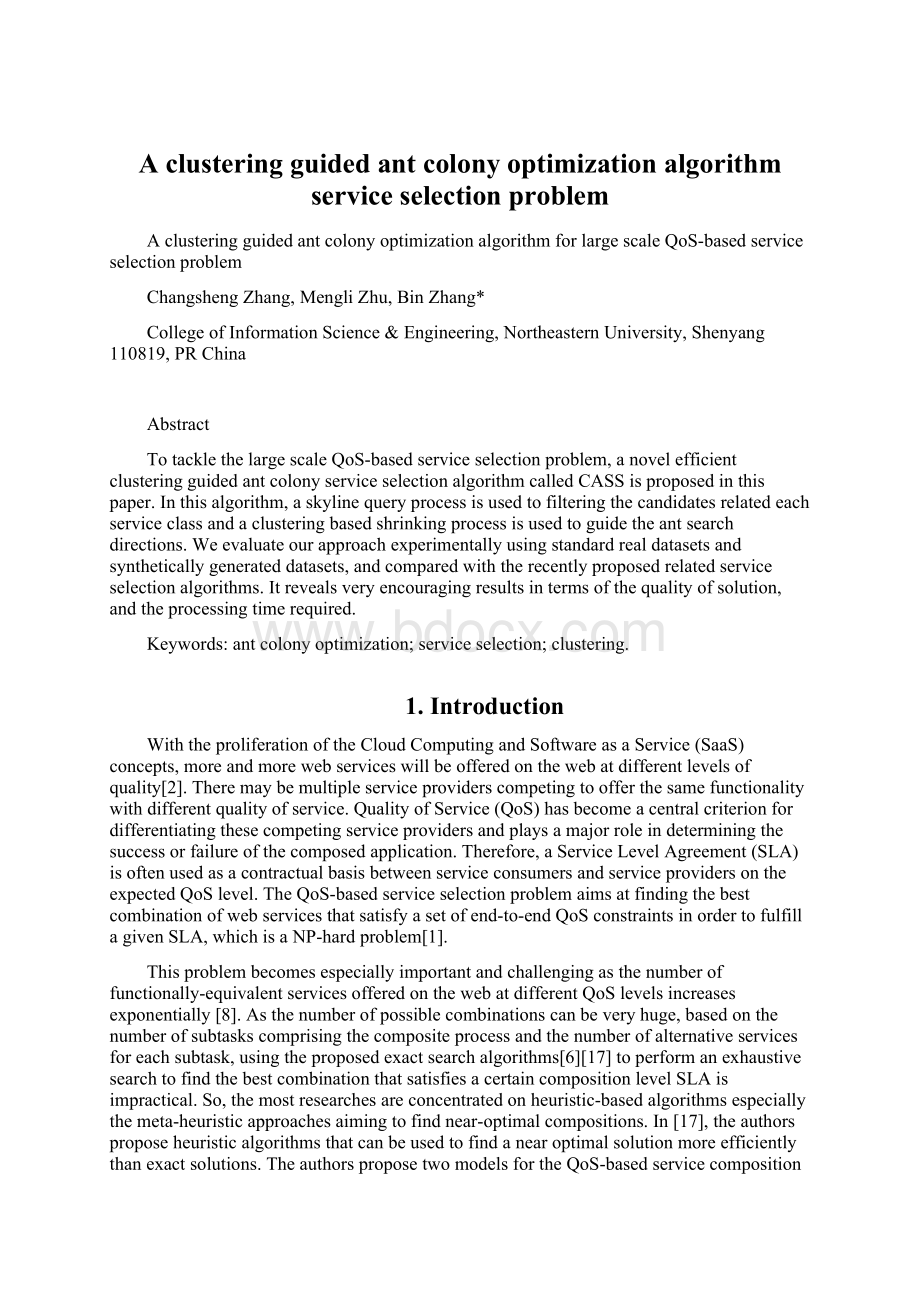
Abstract
TotacklethelargescaleQoS-basedserviceselectionproblem,anovelefficientclusteringguidedantcolonyserviceselectionalgorithmcalledCASSisproposedinthispaper.Inthisalgorithm,askylinequeryprocessisusedtofilteringthecandidatesrelatedeachserviceclassandaclusteringbasedshrinkingprocessisusedtoguidetheantsearchdirections.Weevaluateourapproachexperimentallyusingstandardrealdatasetsandsyntheticallygenerateddatasets,andcomparedwiththerecentlyproposedrelatedserviceselectionalgorithms.Itrevealsveryencouragingresultsintermsofthequalityofsolution,andtheprocessingtimerequired.
Keywords:
antcolonyoptimization;
serviceselection;
clustering.
1.Introduction
WiththeproliferationoftheCloudComputingandSoftwareasaService(SaaS)concepts,moreandmorewebserviceswillbeofferedonthewebatdifferentlevelsofquality[2].Theremaybemultipleserviceproviderscompetingtoofferthesamefunctionalitywithdifferentqualityofservice.QualityofService(QoS)hasbecomeacentralcriterionfordifferentiatingthesecompetingserviceprovidersandplaysamajorroleindeterminingthesuccessorfailureofthecomposedapplication.Therefore,aServiceLevelAgreement(SLA)isoftenusedasacontractualbasisbetweenserviceconsumersandserviceprovidersontheexpectedQoSlevel.TheQoS-basedserviceselectionproblemaimsatfindingthebestcombinationofwebservicesthatsatisfyasetofend-to-endQoSconstraintsinordertofulfillagivenSLA,whichisaNP-hardproblem[1].
Thisproblembecomesespeciallyimportantandchallengingasthenumberoffunctionally-equivalentservicesofferedonthewebatdifferentQoSlevelsincreasesexponentially[8].Asthenumberofpossiblecombinationscanbeveryhuge,basedonthenumberofsubtaskscomprisingthecompositeprocessandthenumberofalternativeservicesforeachsubtask,usingtheproposedexactsearchalgorithms[6][17]toperformanexhaustivesearchtofindthebestcombinationthatsatisfiesacertaincompositionlevelSLAisimpractical.So,themostresearchesareconcentratedonheuristic-basedalgorithmsespeciallythemeta-heuristicapproachesaimingtofindnear-optimalcompositions.In[17],theauthorsproposeheuristicalgorithmsthatcanbeusedtofindanearoptimalsolutionmoreefficientlythanexactsolutions.TheauthorsproposetwomodelsfortheQoS-basedservicecompositionproblemandintroduceaheuristicforeachmodel.In[5],theauthorspresentageneticalgorithmforthisproblem,includingthedesignofaspecialrelationmatrixcodingschemeofchromosomes,evolutionfunctionofpopulationandpopulationdiversityhandlingwithsimulatedannealing.In[18],anewcooperativeevolution(Co-evolution)algorithmconsistsofstochasticparticleswarmoptimization(SPSO)andsimulatedannealing(SA)ispresentedtosolvethisproblem.
Asameta-heuristicapproach,theACOalgorithmisdefinedbyM.Dorigo[12],motivatedbytheintelligentbehaviorofantsystem.Ithasbeenappliedtosolvemanyproblemsandobtainedsatisfyingresults[3][14].Inthispaper,theACOalgorithmisextendedforsolvingtheQoSbasedserviceselectionproblem.Inthisalgorithm,anunsupervisedclusteringprocessisusedforconstructingadirectedclusteringgraphtoguidetheantsmakingexploration,andadynamicexpandingprocessisusedtoenlargethispathforantsmakingexploitationbasedontheobtainedglobalinformation.Furthermore,theMulti-criteriaDominanceRelationships[7]isintroducedtoreducethesearchspaceaswellasant-basedclustering[11]tofurtherimprovetheserviceselectionefficiency.WehavecomparedourapproachwiththerecentlyproposedserviceselectionalgorithmDiGA[5]andSPSO[18].Theperformanceofthesealgorithmshasbeentestedonavarietyofdatasetsprovidedfromseveralreal-lifesituationsandsyntheticallygenerateddatasets.Thecomputationalresultsdemonstratetheeffectivenessofourapproachincomparisontotheseapproaches.Thispaperisorganizedasfollows.InSection2,wegivethedefinitionoftheQoS-basedserviceselectionproblemandthebasicantcolonyalgorithm.TheCASSalgorithmincludingitsmodelandconcretealgorithmdescriptionisprovidedinsection3.Section4presentexperimentalstudiesandcomparedtheCASSwithsomeotherrecentlyproposedalgorithms.Finally,Section5summarizesthecontributionofthispaperalongwithsomefutureresearchdirections.
2.Problemdefinitionandantcolonyalgorithm
2.1TheQoS-basedserviceselectionproblem
Foracompositeapplicationthatisspecifiedasabstractprocessescomposedofasetofabstractservices
eachabstractservicei,i[0,||
||-1]correspondstoaserviceclassSi={si1,si2,…,sin},andSiconsistsofallservicesthatdeliverthesamefunctionalitybutpotentiallydifferintermsQoSvalues.SincetheQoSattributeswhicharepublishedbytheserviceprovider,maybepositiveornegative.WeusethevectorQs={q1(s),q2(s),…,qr(s)}torepresenttheQoSvaluesofservices,andthefunctionqi(s)determinesthepublishedvalueofthei-thattributeoftheservices.Then,theQoSvectorforacompositeserviceconsistingofn,n[1,||
||]servicecomponentsCS={s1,s2,…,sn}isdefinedasQCS={q1(CS),q2(CS),…,qr(CS)},wheretheqi(CS)istheestimatedend-to-endvalueofthei-thQoSattributeandcanbecomputedbyaggregatingthecorrespondingvaluesofcomponentservices.
Definition1.(FeasibleSelection):
Foragivenabstractprocess
andavectorofglobalQoSconstraintsC={c1,c2,…,cm},1mr,whichrefertotheuser’srequirementsandexpressedintermsofavectorofupper(orlower)boundsfordifferentQoScriteria,weconsideraselectionofconcreteservicesCStobeafeasibleselection,iffitcontainsexactlyoneserviceforeachserviceclassSiof
anditsaggregatedQoSvaluessatisfytheglobalQoSconstraints,i.e.q1(CS)ck,k[1,m].
InordertoevaluatetheoverallqualityofagivenfeasibleselectionCS,autilityfunctionUisusedwhichmapsthequalityvectorQCSintoasinglerealvalueanddefinesasfollows:
(1)
with
beingtheweightofqktorepresentuser’spriorities,
and
(2)
beingtheminimumandmaximumaggregatedvaluesofthek-thQoSattributeforcompositeserviceCS,andFdenotinganaggregationfunctionthatdependsonQoScriteriashownasintable1.
Definition2.(ServiceSelection):
andavectorofglobalQoSconstraintsC={c1,c2,…,cm},1mr,theserviceselectionistofindthefeasibleselectionthatmaximizestheoverallutilityfunctionUvalue.
2.2Theantcolonyoptimizationalgorithm
Innature,foragerantscommunicateindirectlybydepositingandsensingpheromonetrails.Thissetsupapositivefeedbackloopthatreinforcespromisingpaths.TheACOalgorithmisinspiredbythisbehaviorofrealants,inwhichtheartificialantscompleteaseriesofwalksofadatastructure,knownasaconstructiongraph.Theylaypheromonetrailsonthisgraphedgesandchoosetheirpathwithrespecttoprobabilitiesthatdependonpheromonetrailsandthesepheromonetrailsprogressivelydecreasebyevaporation.Inmostcases,pheromonetrailsareupdatedonlyafterhavingconstructedacompletepathandnotduringthewalk,andtheamountofpheromonedepositedisusuallyafunctionofthequalityofthepath.Furthermore,theprobabilityforanartificialanttochooseanedgeoftendependsnotonlyonpheromones,butalsoonsomeproblem-specificlocalheuristics.TheclassicalACOalgorithmisshowasAlgorithm1.
Ateachcycle,eachantconstructsacompleteassignmentandthenpheromonetrailsareupdatedincludingthepheromonedepositingandevaporating.Thefitisafitnessfunctionusedtoevaluateanassignment.WecanseethatwhenusingtheACOmeta-heuristictosolveanewcombinatorialoptimizationproblem,oneofthemaintasksistomodeltheproblemasthesearchofafeasibleminimumcostpathoveraweightedgraph,wherethefeasibilityisdefinedwithrespecttoasetofconstraints.
3.Theclusteringbasedantcolonyalgorithmforserviceselection
Obviously,foranapplicationrequestwithnserviceclassesandlcandidateservicesperclass,therearelnpossiblecombinationstobeexamined.So,whenthenumberoffunctionally-equivalentservicesofferedbecomeslarge,howtoeffectivelyshrinkingthesolutionspaceandmakethesearchquicklygorightdirectionisveryimportant.IntheCASSalgorithm,askylinequeryprocessisusedtofilteringthecandidatesrelatedeachserviceclass,andanunsupervisedclusteringprocessisintroducedtopartitiontheskylineservicesinperserviceclass.Thenadirectedclusteringgraphisconstructedbasedonclusteringresulttoabstractthesearchspace,andusedtoguidetheantsglobalsearching.
Definition3.(SkylineServices):
TheskylineofaserviceclassS,denotedbySLS,comprisesthesetofthoseservicesinSthatarenotdominatedbyanyotherservice,i.e.,SLS={i∈S|¬
∃j∈S;
j≺i}.WeregardtheseservicesastheskylineservicesofS.
Definition4.(Dominance):
ConsideraserviceclassS,andtwoservicesi,jS,characterizedbyasetofQofQoSattributes.idominatesj,denotedasi≺j,ifiisasgoodorbetterthanjinallparametersinQandbetterinatleastoneparameterinQ,i.e.∀k∈[1,|Q|]:
qk(x)≤qk(y)and∃k∈[1,|Q|]:
qk(x)<
qk(y).
Sincenota