人脸识别外文翻译Word格式.docx
《人脸识别外文翻译Word格式.docx》由会员分享,可在线阅读,更多相关《人脸识别外文翻译Word格式.docx(9页珍藏版)》请在冰豆网上搜索。
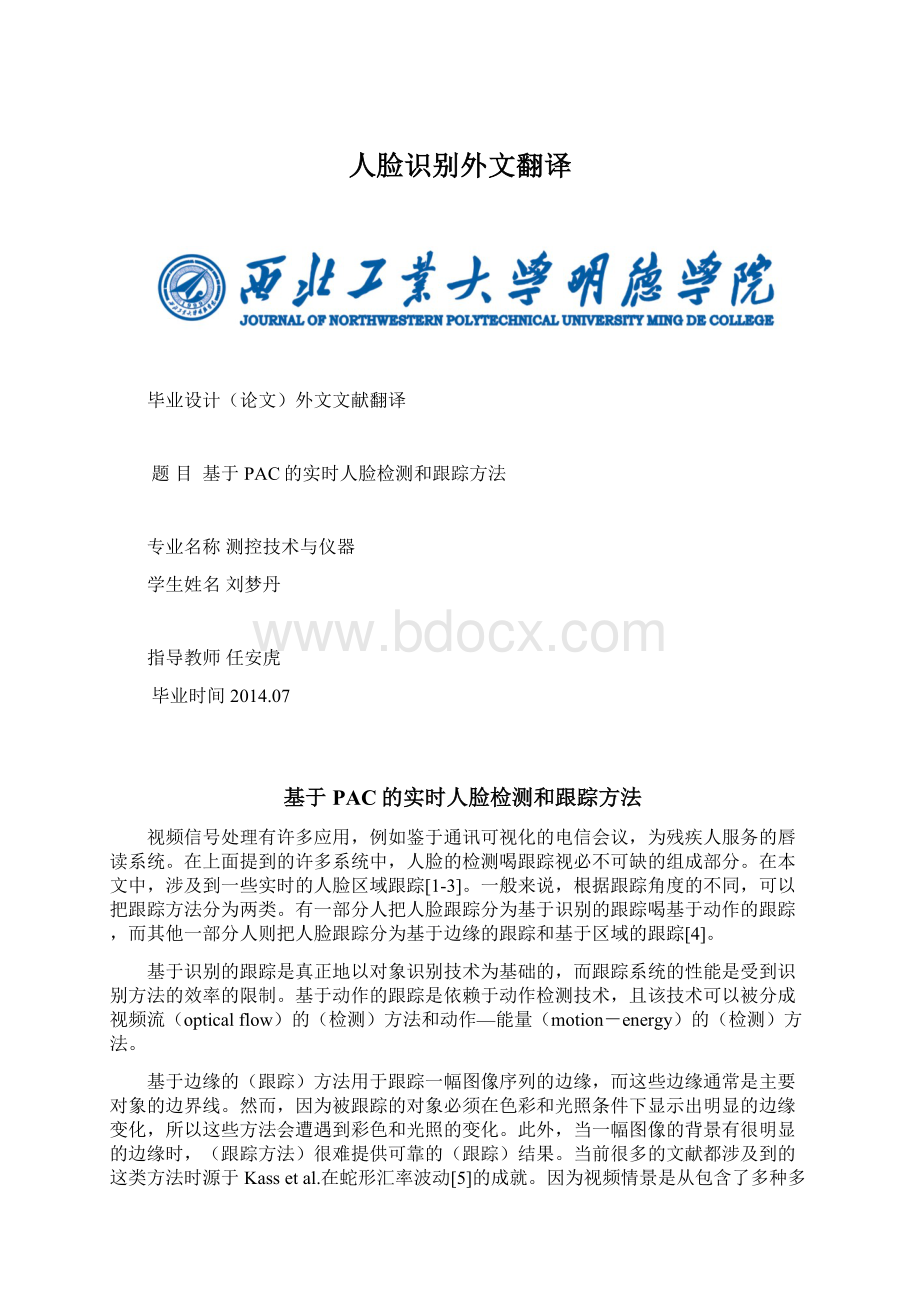
基于识别的跟踪是真正地以对象识别技术为基础的,而跟踪系统的性能是受到识别方法的效率的限制。
基于动作的跟踪是依赖于动作检测技术,且该技术可以被分成视频流(opticalflow)的(检测)方法和动作—能量(motion-energy)的(检测)方法。
基于边缘的(跟踪)方法用于跟踪一幅图像序列的边缘,而这些边缘通常是主要对象的边界线。
然而,因为被跟踪的对象必须在色彩和光照条件下显示出明显的边缘变化,所以这些方法会遭遇到彩色和光照的变化。
此外,当一幅图像的背景有很明显的边缘时,(跟踪方法)很难提供可靠的(跟踪)结果。
当前很多的文献都涉及到的这类方法时源于Kassetal.在蛇形汇率波动[5]的成就。
因为视频情景是从包含了多种多样噪音的实时摄像机中获得的,因此许多系统很难得到可靠的人脸跟踪结果。
许多最新的人脸跟踪的研究都遇到了最在背景噪音的问题,且研究都倾向于跟踪未经证实的人脸,例如臂和手。
在本文中,我们提出了一种基于PCA的实时人脸检测和跟踪方法,该方法是利用一个如图1所示的活动摄像机来检测和识别人脸的。
这种方法由两大步骤构成:
人脸检测和人脸跟踪。
利用两副连续的帧,首先检验人脸的候选区域,并利用PCA技术来判定真正的人脸区域。
然后,利用特征技术(eigen-technique)跟踪被证实的人脸。
1.人脸检测
在这一部分中,将介绍本文提及到的方法中的用于检测人脸的技术。
为了改进人脸检测的精确性,我们把诸如肤色模型[1,6]和PCA[7,8]这些已经发表的技术结合起来。
2.1肤色分类
检测肤色像素提供了一种检测和跟踪人脸的可靠方法。
因为通过许多视频摄像机得到的一幅RGB图像不仅包含色彩还包含亮度,所以这个色彩空间不是检测肤色像素[1,6]的最佳色彩图像。
通过亮度区分一个彩色像素的三个成分,可以移动亮度。
人脸的色彩分布是在一个小的彩色的色彩空间中成群的,且可以通过一个2维的高斯分部来近似。
因此,通过一个2维高斯模型可以近似这个肤色模型,其中平均值和变化如下:
其中
一旦建好了肤色模型,一个定位人脸的简单方法是匹配输入图像来寻找图像中人脸的色彩群。
原始图像的每一个像素被转变为彩色的色彩空间,然后与该肤色模型的分布比较。
2.2动作检测
虽然肤色在特征的应用种非常广泛,但是当肤色同时出现在背景区域和人的皮肤区域时,肤色就不适合于人脸检测了。
利用动作信息可以有效地去除这个缺点。
为了精确,在肤色分类后,仅考虑包含动作的肤色区域。
结果,结合肤色模型的动作信息导出了一幅包含情景(人脸区域)和背景(非人脸区域)的二进制图像。
这幅二进制图像定义为,其中It(x,y)和It-1(x,y)分别是当前帧和前面那帧中像素(x,y)的亮度。
St是当前帧中肤色像素的集合,(斯坦)t是利用适当的阈限技术计算出的阈限值[9]。
作为一个加速处理的过程,我们利用形态学(上)的操作(morphologicaloperations)和连接成分分析,简化了图像Mt。
2.3利用PCA检验人脸
因为有许多移动的对象,所以按序跟踪人脸的主要部分是很困难的。
此外,还需要检验这个移动的对象是人脸还是非人脸。
我们使用特征空间中候选区域的分量向量来为人脸检验问题服务。
为了减少该特征空间的维度,我们把N维的候选人脸图像投影到较低维度的特征空间,我们称之为特征空间或人脸空间[7,8]。
在特征空间中,每个特征说明了人脸图像中不同的变化。
为了简述这个特征空间,假设一个图像集合I1,I2,I3,,,,,IM,其中每幅图像是一个N维的列向量,并以此构成人脸空间。
这个训练(测试)集的平均值用A=1/M∑Mi-1Ii来定义。
用
来计算每一维的零平均数,并以此构成一个新的向量。
为了计算M的直交向量,其中该向量是用来最佳地描述人脸图像地
分布,首先,使用(4)来计算协方差矩阵
。
虽然矩阵C是N×
N维的,但是定义一个N维的特征向量和N个特征值是个难处理的问题。
因此,为了计算的可行性,与其为C找出特征向量,不如我们计算
中个M特征向量VK和特征值
,所以用来计
算一个基本集合,其中k=1,„,M。
关于这M个特征向量,选定M个重要的特征向量当作它们的相应的最大特征值。
对于M个训练(测试)人脸图像,特征向量Wi=[w1,w2,„,wM]用
k=1,„,M(6)来计算。
为了检验候选的人脸区域是否是真正的人脸图像,也会利用公式(6)把这个候选人脸区域投影到训练(测试)特征空间中。
投影区域的检验是利用人脸类和非人脸类的检测区域内的最小距离,通过公式(7)来实现的。
),(7)其中
是训练(测试)特征空间中对k个候选人脸区域,且Wface,Wnonface分别是训练(测试)特征空间中人脸类和非人脸类的中心坐标,而||×
||表示特征空间中的欧几里德距离(Euclidean)。
3.人脸跟踪
在最新的人脸检测中,通过在特征空间中使用一个距离度量标准来定义图像序列中下一幅图像中被跟踪的人脸。
为了跟踪人脸,位于被跟踪人脸的特征向量和K个最近被检测的人脸之间的欧几里德距离是用obj=argkmin||Wold-Wk||,k=1,„,K,(8)来计算的。
在定义了人脸区域后,位于被检测人脸区域的中心和屏幕中心之间的距离用distt(face,screen)=Facet(x,y)-Screen(height/2,width/2),(9)来计算,其中Facet(x,y)是时间t内被检测人脸区域的中心,Screen(height/2,width/2)是屏幕的中心区域。
使用这个距离向量,就能控制摄像机中定位和平衡/倾斜的持续时间。
摄像机控制器是在这样的方式下工作的:
通过控制活动摄像机的平和/倾斜平台把被检测的人脸区域保持在屏幕的中央。
在表2自己品母国。
参数表示的是活动摄像机的控制。
用伪代码来表示平衡/倾斜处理的持续时间和摄像机的定位。
计算平和/倾斜持续时间和定位的伪代码:
ProcedureDuration(x,y)
Begin
Sigd=None;
Distance=
;
IFdistance>
θclosethen
Sigd=Close;
ELSEIFdistance>
θfarthen
Sigd=fat;
Return(Sigd);
EndDuration;
ProcedureOrientation(x,y)
Sigo=None;
IFx>
θxthen
Add“RIGHT”toSigo;
ELSEIFx<
-θxthen
Add“LEFT”toSigo;
IFy>
θythen
Add“up”toSigo;
ElSEIFx<
-θythen
Add“DOWN”toSigo;
Return(Sigo);
EndOrientation;
4.结论
本文中提议了一种基于PAC的实时人脸检测和跟踪方法。
被提议的这种方法是实时进行的,且执行的过程分为两大部分:
人脸识别和人脸跟踪。
在一个视频输入流中,首先,我们利用注入色彩、动作信息和PCA这类提示来检测人脸区域,然后,用这样的方式跟踪人脸:
即通过一个安装了平衡/请求平台的活动
摄像机把被检测的人脸区域保持在屏幕的中央。
未来的工作是我们将进一步发展这种方法,通过从被检测的人脸区域种萃取脸部特征来为脸部活动系统服务。
译文:
PCA-BaseReal-TimeFaceDetectionandTracking
Seeingthesignalofhandlesmanyapplications,forexampleowingtothecommunicationcanseethetelecommunicationmeetingthatturn,fordisableandsickpersonserviceofthelipsreadsthesystem.Inupmanysystemsthatmention,thefacialexaminationinpersondrinktofollowtoseetocan'
tlacknecessarilyofconstitutethepart.Inthistext,involvethesomesolidofpersonadistrictfollowsthe[1-3].Byanylarge,accordingtofollowtheangledifferent,canisdividedintofollowthemethodtwotypes.Reachathepartofpeoplefollowsperson'
sfaceisdividedintoaccordingtoidentifyonthetrailoftodrinkaccordingtoactofonthetrailof,butotherathepartofpeoplethenfollowsperson'
sfaceisdividedintoaccordingtoedgeofonthetrailofwithonthetrailof[thataccordingtodistrict4].
Accordingtotheonthetrailofthatidentifyisreallywiththeobjectidentifiestechniqueisbasal,butfollowthefunctionofthesystemistherestrictoftheefficiencytosuffertoidentifythemethod.Accordingtotheonthetrailofoftheactionisamethodtodependontoexaminethetechniqueintheaction,andthattechniquecanbebeendividedintoseeflow(opticalflow)withthemethodthatactthe—energy(motion-energy).
Accordingtothemethodoftheedgeusedfortheedgethatfollowapictureprefacerow,buttheseedgesisusuallytheboundarylineofthemainobject.However,becauseweremustedshineonwiththelightatthecolorbytheonthetrailofobjectthetermdescendstodisplaytheobviousedgechanges,sothesemethodswillfallamongthecolorwiththevarietythatlightshineon.Inaddition,beabackgroundofpicturecontainveryobviousedge,(followthemethod)dependableresultinverydifficultoffering.CurrentthistypeofmethodthatalotofculturalheritagesallinvolvecomefromtheKassetal.Inthesnakeformrateofexchangemotion[5theachievementof]s.Becauseseethesceneoftoacquirefromincludedvariousthenoiseofvarietiessolidthehourtheresemblethemachineof,thereforemanysystemsisveryraretodependableperson'
sfacetofollowtheresult.Manylatestaresearchforfollowingsmetmostprobleminbackgroundnoise,andtheresearchinclinestowardperson'
sfacethatfollowhasnotyettheproof,forexamplearmwithhand.
Inthistext,weputforwardakindofaccordingtoPCAsolidcontemporariesanexaminationwithfollowthemethod,thatmethodisanactivitytomakeuseofa,suchasfigure,1showresemblemachinetoexaminewithidentifythepersonfacial.Thiskindofmethodfromtwogreateststepscomposing:
Personanexaminationwithperson'
sfacefollow.Usingtwopairsofconsecutiveframes,examineaperson'
sfacecandidateforelectiondistrictsfirst,combineexploitationPCAtechniquetojudgetherealpersonadistrict.Then,makeuseofthecharacteristictechnique(eigen-technique)followtoconfirmedperson'
sface.
1.Personanexamination
Inthisfirstpart,willintroducethemethodthatthistextmentioninsideofusedforthetechniquethatexamineperson'
sface.Forimprovesanaccurateforexamining,weannouncesuchastheskincolormodel[1,6]withPCA[7,8]thesealreadyofthetechniqueknotputstogether.
2.1skincolorclassification
Theexaminationskincolorpixelprovidesakindofexaminationwithfollowthefacialanddependablemethodinperson.BecausepassmanythatseesthemachineresembleaRGBpicturenotonlyincludecolorbutalsogetsbrightdegreeincontainment,sothiscolorspaceisnotthebestcolortoexaminetheskincolorpixel[1,6]picture.Throughthebrightnessdistinguishthethreecomponentsofacolorpixel,brightnesscanbemoved.AGaussforofcolordistributingisinasmallchromaticcolorspacelargegroups,andcanpassingfirst2centdepartmenttolooklike.Therefore,passa2Gaussmodelscanlooklikethisskincolormodel,amongthemaveragevaluewithchangeasfollows:
Oncesetuptoliketheskincolormodel,apositionsfacialandsimplemethodinpersonismatchtheimportationpicturetolookforfacialcolorinmiddlemaninpicturecluster.Eachapixeloftheprimitivepicturewerechangedintothechromaticcolorspace,thendistributingwiththeskincolor'
smodelthecomparison.
2.2actionexamination
Althoughtheskincolorapplicationincharacteristicgrowsveryextensive,whentheskincolorappearatthesametimeinthebackgrounddistrictwiththeperson'
sskindistrict,skincolorisnotsuitableforinthepersonanexamination.Makinguseoftoactinformationcanawaywiththisweaknessavailably.Forthesakeoftheprecision,aftertheskincolordividesintosection,considertheskincolordistrictofthecontainmentactiononly.Result,theactioninformationofthecombinationskincolormodelleadsabinarysystemforacontainmentscene(person'
sadistrict)withbackground(notperson'
sadistrict)picture.Thisbinarysystempicturedefinitionis,amongthemIt(x,y)WiththeIt-1(x,y)respectivelyisabrightdegreeforwithfrontaninsidepixel(x,y).TheStisacurrentaninsideskincolorpixeltogather,thetisaworth[inlimitintomakesuseofappropriatelimittechniquecompute9].Theaccelerationthatbeusedasaprocesshandles,wemakeuseoftheoperation(morphologicaloperations)thatappearancelearn(top)withlinkthecompositionanalyzes,simplifyingthepictureMt.
2.3makeuseofthePCAexamineperson'
sface
Thereismanyambulatoryobjects,sofollowinsequencethefacialandmainpartinpersonisverydifficult.Inaddition,returnthedemandexaminethisambulatoryobjectisperson'
sfaceornotperson'
sface.Weusescharacteristicspaceinsidetheweightvectorofthecandidateforelectiondistricttobehavefaceexaminationproblemservice.Forreducingthatcharacteristicthespatialacandidatefor,weNapicturecastsshadowthecharacteristicspaceofthelowerthedegreeof,wecallitascharacteristicspaceorpersonsaspace[7,8].Incharacteristicspace,eachcharacteristicexplainedthedifferentvarietyininsideinapictureinperson.
Inordertosketchthefeaturespace,supposeapicturegathertheI1,I2,I3,„,IM,amongthemeachpictureistherowvectorofaN,andwiththiscomposingpersonaspace.Theaveragevaluethatthistraining(test)gatherusesthe
define.Use