JMP和Minitab的比较二简单回归分析文档格式.docx
《JMP和Minitab的比较二简单回归分析文档格式.docx》由会员分享,可在线阅读,更多相关《JMP和Minitab的比较二简单回归分析文档格式.docx(12页珍藏版)》请在冰豆网上搜索。
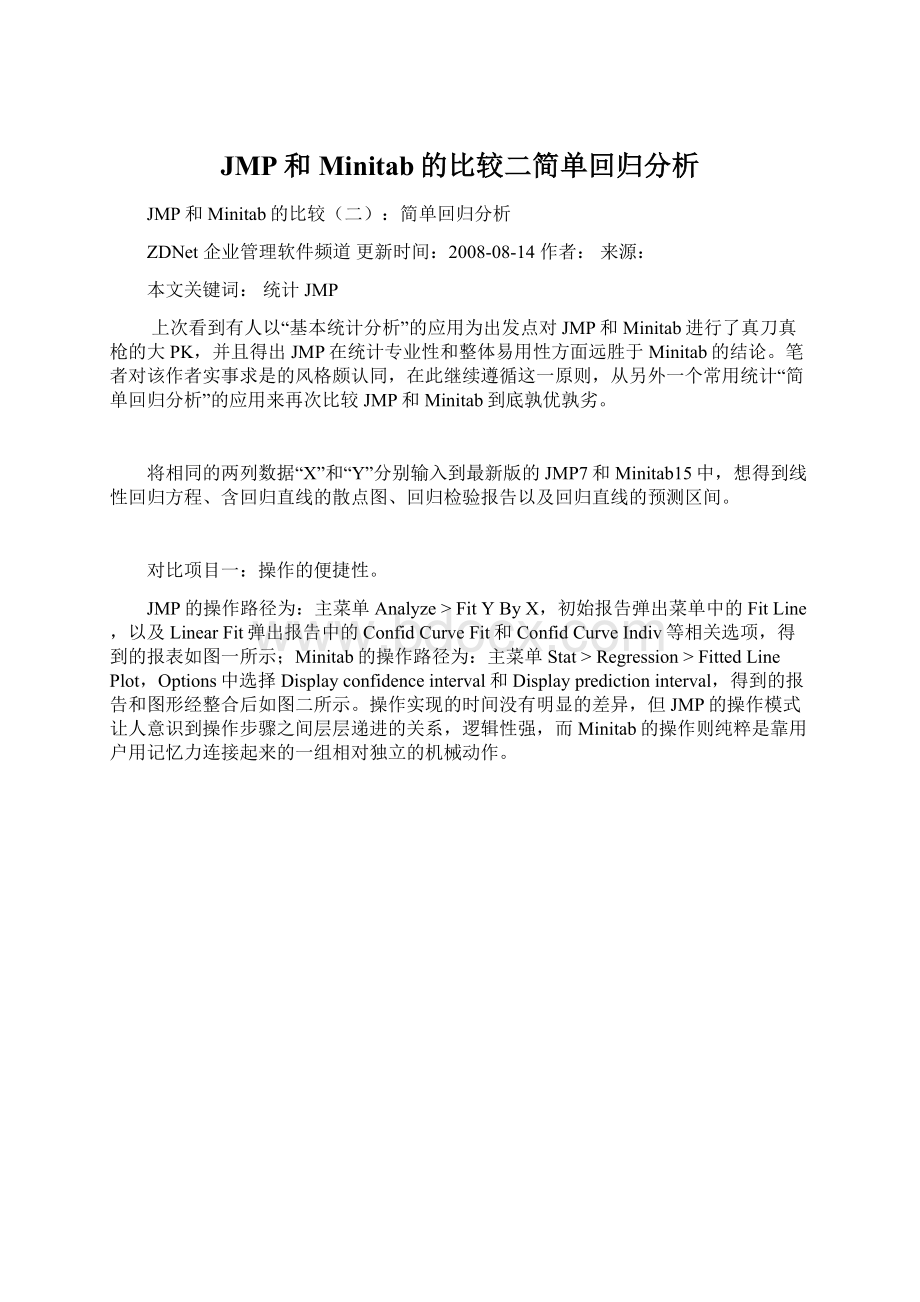
将相同的两列数据“X”和“Y”分别输入到最新版的JMP7和Minitab15中,想得到线性回归方程、含回归直线的散点图、回归检验报告以及回归直线的预测区间。
对比项目一:
操作的便捷性。
JMP的操作路径为:
主菜单Analyze>
FitYByX,初始报告弹出菜单中的FitLine,以及LinearFit弹出报告中的ConfidCurveFit和ConfidCurveIndiv等相关选项,得到的报表如图一所示;
Minitab的操作路径为:
主菜单Stat>
Regression>
FittedLinePlot,Options中选择Displayconfidenceinterval和Displaypredictioninterval,得到的报告和图形经整合后如图二所示。
操作实现的时间没有明显的差异,但JMP的操作模式让人意识到操作步骤之间层层递进的关系,逻辑性强,而Minitab的操作则纯粹是靠用户用记忆力连接起来的一组相对独立的机械动作。
对比项目二:
输出报表的整体效果。
JMP将统计分析结果和相关图形天然地整合在一起,用户查阅起来一目了然。
而Minitab的统计分析结果显示在Session窗口,而相关图形又显示在另一个独立的Graph窗口中,查阅起来平添了几分麻烦。
如果分析的数据、内容、次数一多,这种麻烦就更难忍受了。
对比项目三:
统计分析的具体内容。
无论是回归方程的系数,还是R2、显著性检验P值等等,JMP和Minitab的输出结果都是一致的,这说明两种软件背后所遵循的统计原理其实都是一样的。
如果观察得更仔细一些,你会发现JMP中的小数位保留得比Minitab更多,而且可以自定义,显得更精确、更专业一些。
图二Minitab的输出结果
对比项目四:
统计图形的效果。
在回归分析的早期,只需要观察最基本的散点图,JMP和Minitab的图形效果差不多。
但是到了回归模型的预测应用阶段,置信区间的显示至关重要,JMP可以通过“区间阴影化”的方式加深用户对预测模型的理解。
相比之下,Minitab就相形见拙了。
如果要比较边际图的效果,两者的差距就更大了。
JMP只需在原有的报表基础上再选择HistogramBorders就能完成,结果如图三所示。
它既保留了原先预测区间的特征,又能实现其中散点图与直方图之间的动态链接,Minitab则要重新从主菜单中选择Graph>
MarginalPlot,重新在一个新的Graph窗口才能完成,结果如图四所示。
而且可惜的是,原先预测区间的特征消失了,图形之间动态链接的效果更是从来都无法体现的。
图三JMP的边际图
图四Minitab的边际图
对比项目五:
统计分析的拓展性。
JMP和Minitab都考虑到了这一点,但无论是广度,还是深度来看,两者之间的差异都很明显。
先看广度,除了两者都具备的功能外,JMP的回归报表中还整合了非参数拟合、样条拟合、分组拟合、特殊拟合和椭圆密度等丰富实用的内容,令Minitab望尘莫及。
即使是双方都涉及的内容,我们也可以挖掘其涉及的深度来观察两者的差别。
以多项式回归为例,JMP最高可支持六次项,Minitab则仅为三次项。
以保存数据为例,JMP不仅能够保存残差值和预测值,而且能够保存预测公式,Minitab则不具备保存公式的功能。
诸如此类,举不胜举。
唯一可以让Minitab挽回一些脸面的是它在进行残差分析的时候会比JMP稍快一些。
总结以上五项对比内容的结果,所有真正理解回归的人都会得到一个一致的结论:
JMP在“简单回归分析”方面远胜于Minitab。
这个结论的正确性在我们做一些简单的工作时可能会体会不深,但是随着分析问题的深入,这种感觉会越来越强烈地让人感受到。
同样,笔者愿以此文抛砖引玉,希望有更多真正理解统计、需要统计来进行质量管理、六西格玛项目的爱好者来交流切磋,共同提高。
k-折交叉验证(K-foldcross-validation)是指将样本集分为k份,其中k-1份作为训练数据集,而另外的1份作为验证数据集。
用验证集来验证所得分类器或者回归的错误码率。
一般需要循环k次,直到所有k份数据全部被选择一遍为止。
CrossValidation
Crossvalidationisamodelevaluationmethodthatisbetterthanresiduals.Theproblemwithresidualevaluationsisthattheydonotgiveanindicationofhowwellthelearnerwilldowhenitisaskedtomakenewpredictionsfordataithasnotalreadyseen.Onewaytoovercomethisproblemistonotusetheentiredatasetwhentrainingalearner.Someofthedataisremovedbeforetrainingbegins.Thenwhentrainingisdone,thedatathatwasremovedcanbeusedtotesttheperformanceofthelearnedmodelon``new'
'
data.Thisisthebasicideaforawholeclassofmodelevaluationmethodscalledcrossvalidation.
Theholdoutmethodisthesimplestkindofcrossvalidation.Thedatasetisseparatedintotwosets,calledthetrainingsetandthetestingset.Thefunctionapproximatorfitsafunctionusingthetrainingsetonly.Thenthefunctionapproximatorisaskedtopredicttheoutputvaluesforthedatainthetestingset(ithasneverseentheseoutputvaluesbefore).Theerrorsitmakesareaccumulatedasbeforetogivethemeanabsolutetestseterror,whichisusedtoevaluatethemodel.Theadvantageofthismethodisthatitisusuallypreferabletotheresidualmethodandtakesnolongertocompute.However,itsevaluationcanhaveahighvariance.Theevaluationmaydependheavilyonwhichdatapointsendupinthetrainingsetandwhichendupinthetestset,andthustheevaluationmaybesignificantlydifferentdependingonhowthedivisionismade.
K-foldcrossvalidationisonewaytoimproveovertheholdoutmethod.Thedatasetisdividedintoksubsets,andtheholdoutmethodisrepeatedktimes.Eachtime,oneoftheksubsetsisusedasthetestsetandtheotherk-1subsetsareputtogethertoformatrainingset.Thentheaverageerroracrossallktrialsiscomputed.Theadvantageofthismethodisthatitmatterslesshowthedatagetsdivided.Everydatapointgetstobeinatestsetexactlyonce,andgetstobeinatrainingsetk-1times.Thevarianceoftheresultingestimateisreducedaskisincreased.Thedisadvantageofthismethodisthatthetrainingalgorithmhastobererunfromscratchktimes,whichmeansittakesktimesasmuchcomputationtomakeanevaluation.Avariantofthismethodistorandomlydividethedataintoatestandtrainingsetkdifferenttimes.Theadvantageofdoingthisisthatyoucanindependentlychoosehowlargeeachtestsetisandhowmanytrialsyouaverageover.
Leave-one-outcrossvalidationisK-foldcrossvalidationtakentoitslogicalextreme,withKequaltoN,thenumberofdatapointsintheset.ThatmeansthatNseparatetimes,thefunctionapproximatoristrainedonallthedataexceptforonepointandapredictionismadeforthatpoint.Asbeforetheaverageerroriscomputedandusedtoevaluatethemodel.Theevaluationgivenbyleave-one-outcrossvalidationerror(LOO-XVE)isgood,butatfirstpassitseemsveryexpensivetocompute.Fortunately,locallyweightedlearnerscanmakeLOOpredictionsjustaseasilyastheymakeregularpredictions.ThatmeanscomputingtheLOO-XVEtakesnomoretimethancomputingtheresidualerroranditisamuchbetterwaytoevaluatemodels.WewillseeshortlythatVizierreliesheavilyonLOO-XVEtochooseitsmetacodes.
Figure26:
Crossvalidationcheckshowwellamodelgeneralizestonewdata
Fig.26showsanexampleofcrossvalidationperformingbetterthanresidualerror.Thedatasetinthetoptwographsisasimpleunderlyingfunctionwithsignificantnoise.Crossvalidationtellsusthatbroadsmoothingisbest.Thedatasetinthebottomtwographsisacomplexunderlyingfunctionwithnonoise.Crossvalidationtellsusthatverylittlesmoothingisbestforthisdataset.
Nowwereturntothequestionofchoosingagoodmetacodefordataseta1.mbl:
File->
Open->
a1.mbl
Edit->
Metacode->
A90:
9
Model->
LOOPredict
L90:
L10:
LOOPredictgoesthroughtheentiredatasetandmakesLOOpredictionsforeachpoint.AtthebottomofthepageitshowsthesummarystatisticsincludingMeanLOOerror,RMSLOOerror,andinformationaboutthedatapointwiththelargesterror.ThemeanabsoluteLOO-XVEsforthethreemetacodesgivenabove(thesamethreeusedtogeneratethegraphsinfig.25),are2.98,1.23,and1.80.Thosevaluesshowthatgloballinearregressionisthebestmetacodeofthosethree,whichagreeswithourintuitivefeelingfromlookingattheplotsinfig.25.Ifyourepeattheaboveoperationondatasetb1.mblyou'
llgetthevalues4.83,4.45,and0.39,whichalsoagreeswithourobservations.
Whatarecross-validationandbootstrapping?
--------------------------------------------------------------------------------
Cross-validationandbootstrappingarebothmethodsforestimatinggeneralizationerrorbasedon"
resampling"
(WeissandKulikowski1991;
EfronandTibshirani1993;
Hjorth1994;
Plutowski,Sakata,andWhite1994;
ShaoandTu1995).Theresultingestimatesofgeneralizationerrorareoftenusedforchoosingamongvariousmodels,suchasdifferentnetworkarchitectures.
Cross-validation
++++++++++++++++
Ink-foldcross-validation,youdividethedataintoksubsetsof(approximately)equalsize.Youtrainthenetktimes,eachtimeleavingoutoneofthesubsetsfromtraining,butusingonlytheomittedsubsettocomputewhatevererrorcriterioninterestsyou.Ifkequalsthesamplesize,thisiscalled"
leave-one-out"
cross-validation."
Leave-v-out"
isamoreelaborateandexpensiveversionofcross-validationthatinvolvesleavingoutallpossiblesubsetsofvcases.
Notethatcross-validationisquitedifferentfromthe"
split-sample"
or"
hold-out"
methodthatiscommonlyusedforearlystoppinginNNs.Inthesplit-samplemethod,onlyasinglesubset(thevalidationset)isusedtoestimatethegeneralizationerror,insteadofkdifferentsubsets;
i.e.,thereisno"
crossing"
.Whilevariouspeoplehavesuggestedthatcross-validationbeappliedtoearlystopping,theproperwayofdoingsoisnotobvious.
Thedistinctionbetweencross-validationandsplit-samplevalidationisextremelyimportantbecausecross-validationismarkedlysuperiorforsmalldatasets;
thisfactisdemonstrateddramaticallybyGoutte(1997)inareplytoZhuandRohwer(1996).Foraninsightfuldiscussionofthelimitationsofcross-validatorychoiceamongseverallearningmethods,seeStone(1977).
Jackknifing
+++++++++++
Leave-one-outcross-validationisalsoeasilyconfusedwithjackknifing.Bothinvolveomittingeachtrainingcaseinturnandretrainingthenetworkontheremainingsubset.Butcross-validationisusedtoestimategeneralizationerror,whilethejackknifeisusedtoestimatethebiasofastatistic.Inthejackknife,youcomputesomestatisticofinterestineachsubsetofthedata.Theaverageofthesesubsetstatisticsiscomparedwiththecorrespondingstatisticcomputedfromtheentiresampleinordertoestimatethebiasofthelatter.Youcanalsogetajackknifeestimateofthestandarderrorofastatistic.Jackknifingcanbeusedtoestimatethebiasofthetrainingerrorandhencetoestimatethegeneralizationerror,butthisprocessismorecomplicatedthanleave-one-outcross-validation(Efron,1982;
Ripley,1996,p.73).
Choiceofcross-validationmethod
+++++++++++++++++++++++++++++++++
Cross-validationcanbeusedsimplytoestimatethegeneralizationerrorofagivenmodel,oritcanbeusedformodelselectionbychoosingoneofseveralmodelsthathasthesmallestestimatedgeneralizationerror.Forexample,youmightusecross-validationtochoosethenumberofhiddenunits,oryoucouldusecross-validationtochooseasubsetoftheinputs(subsetselection).Asubsetthatconta