Formal Verification based on Boolean Expression DiagramsWord文档下载推荐.docx
《Formal Verification based on Boolean Expression DiagramsWord文档下载推荐.docx》由会员分享,可在线阅读,更多相关《Formal Verification based on Boolean Expression DiagramsWord文档下载推荐.docx(27页珍藏版)》请在冰豆网上搜索。
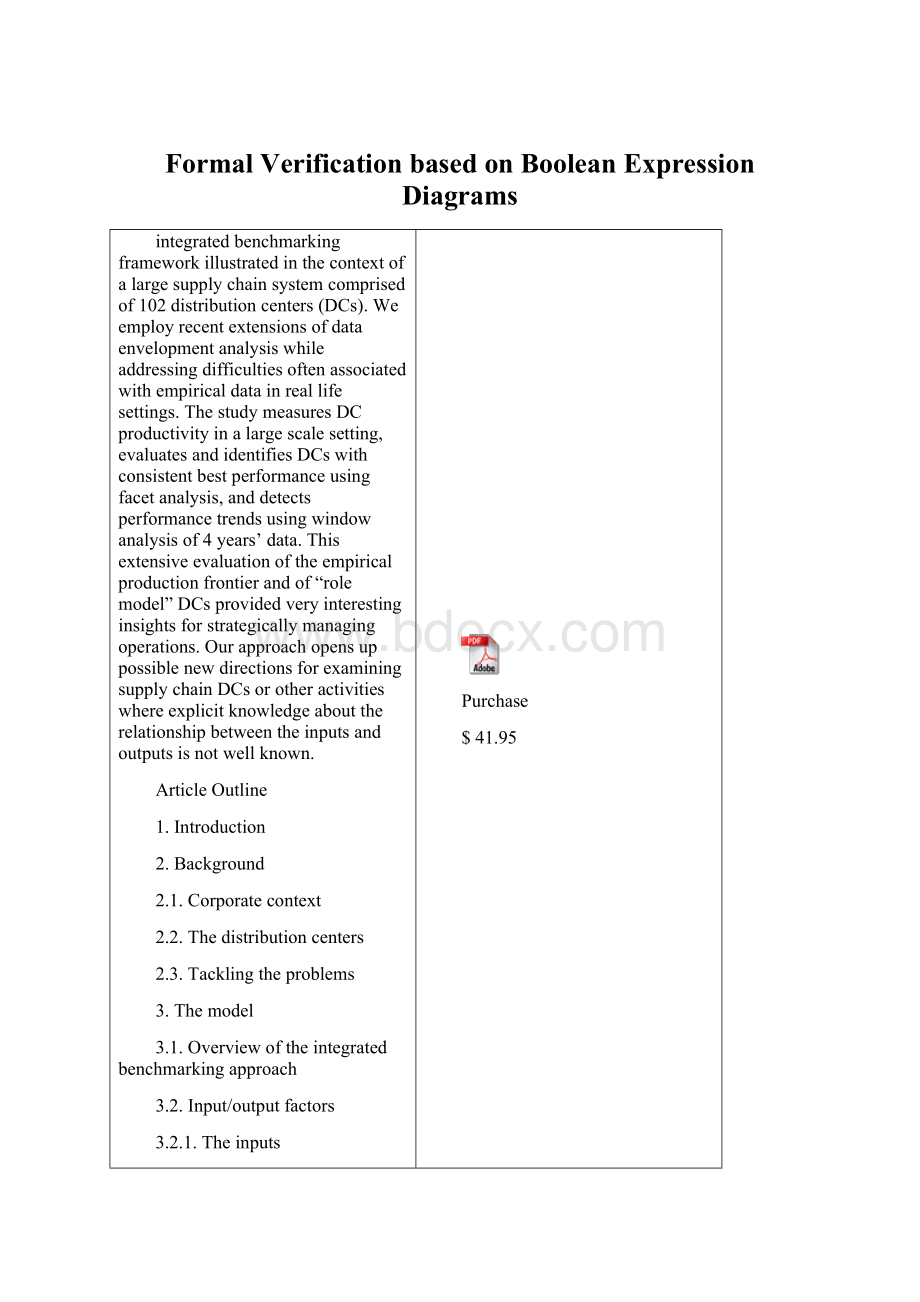
3.3.Modelformulation
3.4.Performancetrendsovertime
4.Results
4.1.Supplynetworkperformancetrends
4.2.Frontiermembership
4.3.Facetanalysis
5.Conclusions
5.1.Theintegratedbenchmarkingapproach
5.2.Managerialinsights
AppendixA
References
Purchase
$41.95
243
Whendodifferencesmatter?
On-linefeatureextractionthroughcognitiveeconomy
OriginalResearchArticle
CognitiveSystemsResearch,Volume6,Issue4,December2005,Pages263-281
DavidJ.Finton
Closepreview
|
Relatedarticles
Relatedreferenceworkarticles
AbstractAbstract|Figures/TablesFigures/Tables|ReferencesReferences
Abstract
Foranintelligentagenttobetrulyautonomous,itmustbeabletoadaptitsrepresentationtotherequirementsofitstaskasitinteractswiththeworld.Mostcurrentapproachestoon-linefeatureextractionareadhoc;
incontrast,thispaperderivesprincipledcriteriaforrepresentationaladequacybyapplyingthepsychologicalprincipleofcognitiveeconomytoreinforcementlearning.Thecriteriaareprincipledbecausetheyarebasedonananalysisoftheamountofrewardtheagentforfeitswhenitgeneralizesoverstates.Thisanalysisshowsthataction-valueerrorsaresometimesirrelevant,andthattheagentmayoptimizeitsperformancewithlimitedcognitiveresourcesbygroupingtogetherstateswhosedifferencesdonotmatterinitstask.Thepaperpresentsanalgorithmbasedonthisanalysis,incorporatinganactiveformofQ-learningandpartitioningcontinuousstate-spacesbymergingandsplittingVoronoiregions.Theexperimentsillustrateanewmethodologyfortestingandcomparingrepresentationsbymeansoflearningcurves.Resultsfromthepuck-on-a-hilltaskdemonstratethealgorithm’sabilitytolearneffectiverepresentations,superiortothoseproducedbysomeother,well-known,methods.
1.Introduction
1.1.Effectiverepresentations
1.2.Functionapproximationandvalueprediction
1.3.Featureextractionandstateabstraction
1.4.Adhocapproachestostateabstraction
1.5.Cognitiveeconomy
2.Representationalcriteria
2.1.Preferenceandvaluefunctions
2.2.Representationaladequacy
2.3.Statecompatibility
3.Analgorithmforon-linefeatureextraction
3.1.Thetoplevelofthealgorithm
3.2.Activestateinvestigations
3.3.Stateabstractionmodule
3.4.Methodology
4.Casestudy:
puck-on-a-hilltask
4.1.Analysis
4.2.Results
4.2.1.Generatedrepresentations
4.2.2.Controlrepresentations
4.2.3.Learningcurves
5.Discussionandrelatedwork
5.1.Assumptions
5.2.Nearest-neighborrepresentation
5.3.Activelearning
5.4.Non-activeapproaches
5.5.Statecompatibility
6.Conclusions
$37.95
244
Minimizingmakespanonanm-machinere-entrantflowshop
Computers&
OperationsResearch,Volume35,Issue5,May2008,Pages1684-1696
Seong-WooChoi,Yeong-DaeKim
Thispaperfocusesonanm-machinere-entrantflowshopschedulingproblemwiththeobjectiveofminimizingmakespan.Inthere-entrantflowshopconsideredhere,routesofalljobsareidenticalasinordinaryflowshops,butthejobsmustbeprocessedmultipletimesonthemachines.Wepresentheuristicalgorithms,whicharemodifiedfromwell-knownexistingalgorithmsforthegeneralm-machineflowshopproblemornewlydevelopedinthisresearch.Forevaluationoftheperformanceofthealgorithms,computationalexperimentsareperformedonrandomlygeneratedtestproblemsandresultsarereported.
2.Notationandassumptions
3.Heuristicalgorithms
3.1.Simpleheuristicalgorithms
3.1.1.Lowerbound-basedalgorithm(LBB)
3.1.2.Idletime-basedalgorithm(ITB)
3.1.3.HybridLBB–ITBalgorithm1(HLI1)
3.1.4.HybridLBB–ITBalgorithm(HLI2)
3.2.Constructivealgorithms
3.2.1.ModifiedNEHalgorithm1(MN1)
3.2.2.ModifiedNEHalgorithm2(MN2)
3.2.3.ModifiedNEHalgorithm3(MN3)
3.3.SOalgorithm
3.4.SAalgorithm
4.Computationalexperiments
5.Conclusions
Acknowledgements
$31.50
245
Habitatsuitabilitymodellingasamappingtoolformacrobenthiccommunities:
AnexamplefromtheBelgianpartoftheNorthSea
ContinentalShelfResearch,Volume28,Issue3,15February2008,Pages369-379
S.Degraer,E.Verfaillie,W.Willems,E.Adriaens,M.Vincx,V.VanLancker
Beingecologicallyimportantandwell-known,thespatialdistributionpatternofthemacrobenthosisoftenusedtosupportanecologicallysustainablemarinemanagement.Thoughinmanycasesthemacrobenthicspatialdistributionisrelativelywell-known,thisinformationismerelyrestrictedtopointobservationsatthesamplingstations:
althoughbeingincreasinglydemanded,fullcoveragespatialdistributionmapsaregenerallylacking.Thisstudythereforeaimedatdemonstratingtheusefulnessofhabitatsuitabilitymodellingasafullcoveragemappingtoolwithhighrelevanceformarinemanagementthrough
(1)theconstructionofahabitatsuitabilitymodelforthesoftsedimentmacrobenthiccommunitiesintheBelgianpartoftheNorthSea(BPNS)and
(2)predictingthefullcoveragespatialdistributionofmacrobenthiccommunitieswithintheBPNS.TheBPNSwasselectedasacasestudyareabecauseofthehighdataavailabilityonbothmacrobenthosandenvironmentalcharacteristics.Discriminantfunctionanalysis(DFA)objectivelyselectedmediangrainsizeandsedimentmudcontentandomittedbathymetry,slopeanddistancetothecoasttorepresentthemostimportantenvironmentalvariablesdeterminingthemacrobenthiccommunitydistribution.Theconsequentcrossvalidated,empiricalhabitatsuitabilitymodel,usingbothmediangrainsizeandmudcontent,showedanaposterioriaveragecorrectlyclassifiedinstances(CCI)of79%(community-dependentCCIrangingfrom72%to86%)andaCohen'
skappaof0.71,pointingtowardsaverygoodagreementbetweenmodelpredictionsandobservations.Theapplicationofthehabitatsuitabilitymodelonthefullcoveragemapsofmediangrainsizeandsedimentmudcontent,takenfromliterature,allowedtoreliablyassessthedistributionofthemacrobenthiccommunitieswithin96.3%ofthe53,297BPNSgridcellswitharesolutionof250
m.NexttoitsapplicabilitytotheBPNS,themodelisfurtheranticipatedtopotentiallyperformwellinthefullSouthernBightoftheNorthSea:
testingisadvisedhere.Sincethehabitatsuitabilityisconsideredfarmorestablethroughtimecomparedtothepermanentlyfluctuatingmacrobenthiccommunities,informationonthehabitatsuitabilityofanareaisconsideredhighlyimportantforascientificallysoundmarinemanagement.
2.Materialsandmethods
2.1.TheBelgianpartoftheNorthSea:
currentknowledge
2.2.Researchstrategy
2.3.Dataavailability
2.3.1.Biologicaldata
2.3.2.Environmentaldata
2.3.2.1.Habitatsuitabilitymodelinputdata
2.3.2.2.Fullcoveragemaps
2.4.Habitatsuitabilitymodelling
2.4.1.Modellingstrategy
2.4.2.Biologicaldataexploration:
communityanalysis
2.4.3.Discriminantfunctionanalysis
2.5.Habitatsuitabilitymapping
3.Results
3.1.Communityanalysis
3.2.Communityhabitatpreferences
3.3.Communityhabitatsuitabilitymodelling
3.3.1.Crossvalidation
3.3.2.Finalmodel
3.4.Habitatsuitabilitymaps
4.Discussion
4.1.Habitatsuitabilitymodel
4.2.Habitatsuitabilitymapping
4.3.Relevanceformarinemanagement
246
Anapplicationofsupportvectormachinesinbankruptcypredictionmodel
ExpertSystemswithApplications,Volume28,Issue1,January2005,Pages127-135
Kyung-ShikShin,TaikSooLee,Hyun-jungKim
Thisstudyinvestigatestheefficacyofapplyingsupportvectormachines(SVM)tobankruptcypredictionproblem.Althoughitisawell-knownfactthattheback-propagationneuralnetwork(BPN)performswellinpatternrecognitiontasks,themethodhassomelimitationsinthatitisanarttof