Multiple Linear Regression Model1Word格式.docx
《Multiple Linear Regression Model1Word格式.docx》由会员分享,可在线阅读,更多相关《Multiple Linear Regression Model1Word格式.docx(12页珍藏版)》请在冰豆网上搜索。
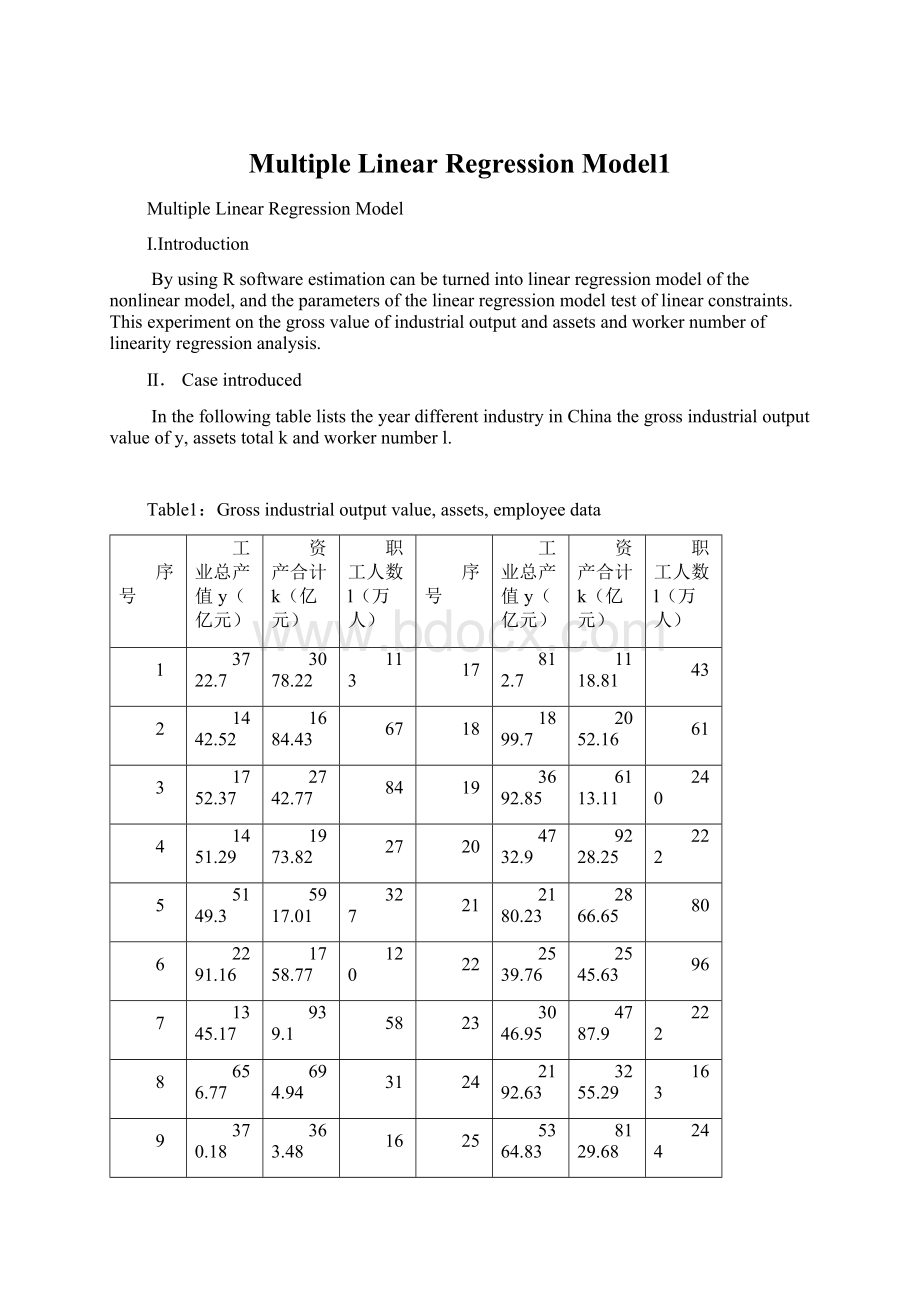
240
4
1451.29
1973.82
27
20
4732.9
9228.25
222
5
5149.3
5917.01
327
21
2180.23
2866.65
80
6
2291.16
1758.77
120
22
2539.76
2545.63
96
7
1345.17
939.1
58
23
3046.95
4787.9
8
656.77
694.94
31
24
2192.63
3255.29
163
9
370.18
363.48
16
25
5364.83
8129.68
244
10
1590.36
2511.99
66
26
4834.68
5260.2
145
11
616.71
973.73
7549.58
7518.79
138
12
617.94
516.01
28
867.91
984.52
46
13
4429.19
3785.91
29
4611.39
18626.94
218
14
5749.02
8688.03
254
30
170.3
610.91
15
1781.37
2798.9
83
325.53
1523.19
45
1243.07
1808.44
33
Ⅲ.ModelBuilding
3.1.Model_1Setamodelfor
Step1:
Readthedata
>
da=read.table("
C:
/23/f.csv"
sep="
"
)
y=da[,2]
k=da[,3]
l=da[,4]
win.graph(width=3.5,height=3.5,pointsize=8)
plot(y,k)#plotthescatterofyandk
plot(y,l)#plotthescatterofyandl
Step2:
PARAMETERESTIMATION
m1=lm(y~k+l)
summary(m1)
Call:
lm(formula=y~k+l)
Residuals:
Min1QMedian3QMax
-2113.0-472.9-232.7196.53928.2
Coefficients:
EstimateStd.ErrortvaluePr(>
|t|)
(Intercept)588.61734339.389361.7340.09386.
k0.199260.081942.4320.02167*
l11.120213.622673.0700.00473**
---
Signif.codes:
0‘***’0.001‘**’0.01‘*’0.05‘.’0.1‘’1
Residualstandarderror:
1143on28degreesoffreedom
MultipleR-squared:
0.6715,AdjustedR-squared:
0.648
F-statistic:
28.62on2and28DF,p-value:
1.706e-07
Itisconcludedthatthefollowingregressionequation:
Analysisofvariance
anova(m1)
AnalysisofVarianceTable
Response:
y
DfSumSqMeanSqFvaluePr(>
F)
k1624666966246669647.80771.624e-07***
l112311730123117309.42250.004725**
Residuals28365854951306625
Residualanalysis
re1=residuals(m1)
plot(re1)
Step3:
Resultsanalysis
1.coefficientofdetermination
BecauseofR^2=0.6715,sothefittingeffectisnotgood
2.TESTSOFHYPOTHESES
Agivenlevelofsignificance:
qf(0.975,2,27)
[1]4.242094
qt(0.975,27)
[1]2.051831
F=28.62>
F(2,27)=4.24
P=1.706e-07<
0.05,Showthatk,lcombinedtohaveasignificantlinearimpactony.
T(k)=2.432〉t(27)=2.05;
T(l)=3.070〉t(27)=2.05,Showthatk,lofyhassignificantlinearinfluence.
3.Accordingtotheresidualfigure,wecanfindthatasthedatasequence,residualobviousdeviationfromthemean,theincreasescope,showedthatpoorregressionresults.
4.Theregressionequationresidualanalysis
plot(m1)
Fromtheaboveknowable,deviatingfromthefittinglinesituationisrelativelyserious,canalsobeconcludedthatthefittingeffectisnotgood.
3.2.Model2
Setamodelfor
Thelogarithmictransformation,
Canbeconvertedinto
Step1:
DATAPROCESSING
y1=log(y)#takelogarithms
k1=log(k)
l1=log(l)
Table2:
data(ln)
Serialnumber
Grossvalueofindustrialoutput
lnY
Assetstotal
lnK
Theworkernumber
lnL
(亿元)
(万人)
8.222204
8.032107
4.727388
7.274147
7.429183
4.204693
7.468724
7.916724
4.430817
7.280208
7.587726
3.295837
8.546616
8.685587
5.78996
7.736814
7.47237
4.787492
7.204276
6.844922
4.060443
6.487334
6.543826
3.433987
5.913989
5.895724
2.772589
7.371716
7.828831
4.189655
6.424399
6.881134
6.426391
6.246126
3.332205
8.395972
8.239042
4.110874
8.656785
9.069701
5.537334
7.485138
7.936982
4.418841
7.125339
7.50022
3.496508
6.700362
7.020021
3.7612
7.549451
7.626648
8.214154
8.718191
5.480639
8.462293
9.130025
5.402677
7.687186
7.960899
4.382027
7.839825
7.842133
4.564348
8.021896
8.473847
7.692857
8.088037
5.09375
8.58762
9.003277
5.497168
8.48357
8.567924
4.976734
8.929247
8.92516
4.927254
6.766088
6.892154
3.828641
8.436285
9.832364
5.384495
5.137562
6.41495
2.944439
5.785455
7.328562
3.806662
Parameterestimation
m2=lm(log(y)~log(k)+log(l))
summary(m2)
lm(formula=log(y)~log(k)+log(l))
-1.20679-0.155170.031790.265320.73927
(Intercept)1.15400.72761.5860.12397
log(k)0.60920.17643.4540.00178**
log(l)0.36080.20161.7900.08432.
0.4255on28degreesoffreedom
0.8099,AdjustedR-squared:
0.7963
59.66on2and28DF,p-value:
8.035e-11
(exp(1.1540)=3.170851)
anova(m2)
log(y)
log(k)121.024921.0249116.10691.805e-11***
log(l)10.58000.58003.20320.08432.
Residuals285.07030.1811
Residualanalysis
re2=residuals(m2)
plot(re2)
2.coefficientofdetermination
:
BecauseofR^2=0.8099,Sothefittingeffectisbetterthanmodel1.
3.TESTSOFHYPOTHESES
Agivenlevelofsignificanceis5%,
F=59.66>
P=8.035e-11<
0.05,ShowthatLNK,LNLcombinedtohaveasignificantlinearimpactonlny
。
T(lnk)=3.454>
2.05,T(lnl)=1.790<
2.05,LNKparametersthroughthetestofsignificanceofvariables;
ButtheparametersoftheLNLfailedtheinspection.
Ifagiven10%significancelevel
qt(0.95,27)
[1]1.703288
1.70;
T(lnl)=1.790>
1.70,SotheLNK,LNLparametersthroughthetestofsignificanceofvariables.
3.Accordingtotheresidualfigure,wecanfindthatinadditiontothethreeabnormalpointsafter,evenlydistributedaroundthezeroresidual,andvolatility,within0.5regressionresultisgood.
4.Theregressionequationresidualfigure
plot(m2)