卷积神经网络机器学习外文文献翻译中英文Word下载.docx
《卷积神经网络机器学习外文文献翻译中英文Word下载.docx》由会员分享,可在线阅读,更多相关《卷积神经网络机器学习外文文献翻译中英文Word下载.docx(8页珍藏版)》请在冰豆网上搜索。
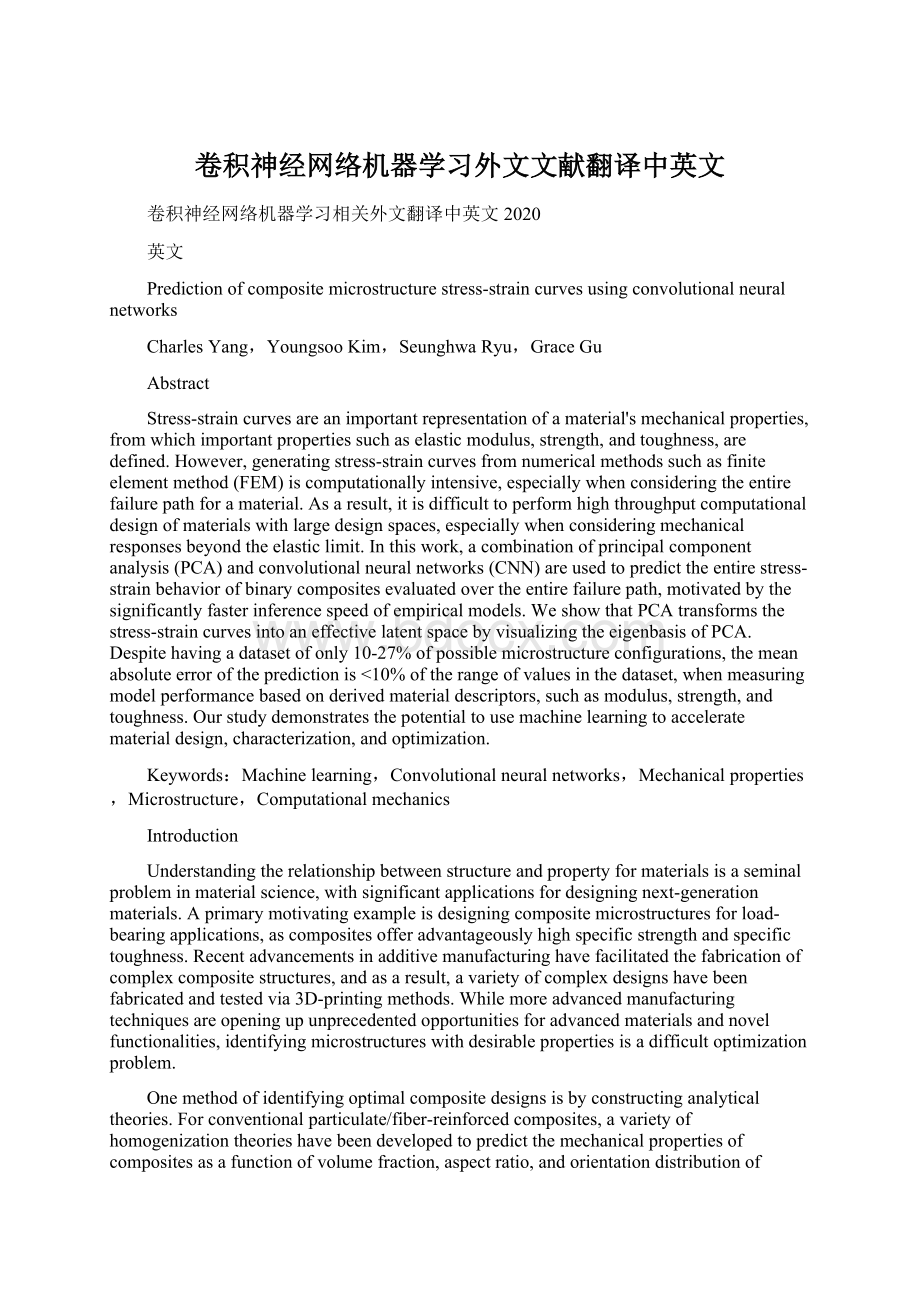
卷积神经网络机器学习外文文献翻译中英文@#@卷积神经网络机器学习相关外文翻译中英文2020@#@英文@#@Predictionofcompositemicrostructurestress-straincurvesusingconvolutionalneuralnetworks@#@CharlesYang,YoungsooKim,SeunghwaRyu,GraceGu@#@Abstract@#@Stress-straincurvesareanimportantrepresentationofamaterial'@#@smechanicalproperties,fromwhichimportantpropertiessuchaselasticmodulus,strength,andtoughness,aredefined.However,generatingstress-straincurvesfromnumericalmethodssuchasfiniteelementmethod(FEM)iscomputationallyintensive,especiallywhenconsideringtheentirefailurepathforamaterial.Asaresult,itisdifficulttoperformhighthroughputcomputationaldesignofmaterialswithlargedesignspaces,especiallywhenconsideringmechanicalresponsesbeyondtheelasticlimit.Inthiswork,acombinationofprincipalcomponentanalysis(PCA)andconvolutionalneuralnetworks(CNN)areusedtopredicttheentirestress-strainbehaviorofbinarycompositesevaluatedovertheentirefailurepath,motivatedbythesignificantlyfasterinferencespeedofempiricalmodels.WeshowthatPCAtransformsthestress-straincurvesintoaneffectivelatentspacebyvisualizingtheeigenbasisofPCA.Despitehavingadatasetofonly10-27%ofpossiblemicrostructureconfigurations,themeanabsoluteerrorofthepredictionis<@#@10%oftherangeofvaluesinthedataset,whenmeasuringmodelperformancebasedonderivedmaterialdescriptors,suchasmodulus,strength,andtoughness.Ourstudydemonstratesthepotentialtousemachinelearningtoacceleratematerialdesign,characterization,andoptimization.@#@Keywords:
@#@Machinelearning,Convolutionalneuralnetworks,Mechanicalproperties,Microstructure,Computationalmechanics@#@Introduction@#@Understandingtherelationshipbetweenstructureandpropertyformaterialsisaseminalprobleminmaterialscience,withsignificantapplicationsfordesigningnext-generationmaterials.Aprimarymotivatingexampleisdesigningcompositemicrostructuresforload-bearingapplications,ascompositesofferadvantageouslyhighspecificstrengthandspecifictoughness.Recentadvancementsinadditivemanufacturinghavefacilitatedthefabricationofcomplexcompositestructures,andasaresult,avarietyofcomplexdesignshavebeenfabricatedandtestedvia3D-printingmethods.Whilemoreadvancedmanufacturingtechniquesareopeningupunprecedentedopportunitiesforadvancedmaterialsandnovelfunctionalities,identifyingmicrostructureswithdesirablepropertiesisadifficultoptimizationproblem.@#@Onemethodofidentifyingoptimalcompositedesignsisbyconstructinganalyticaltheories.Forconventionalparticulate/fiber-reinforcedcomposites,avarietyofhomogenizationtheorieshavebeendevelopedtopredictthemechanicalpropertiesofcompositesasafunctionofvolumefraction,aspectratio,andorientationdistributionofreinforcements.Becausemanynaturalcomposites,synthesizedviaself-assemblyprocesses,haverelativelyperiodicandregularstructures,theirmechanicalpropertiescanbepredictediftheloadtransfermechanismofarepresentativeunitcellandtheroleoftheself-similarhierarchicalstructureareunderstood.However,theapplicabilityofanalyticaltheoriesislimitedinquantitativelypredictingcompositepropertiesbeyondtheelasticlimitinthepresenceofdefects,becausesuchtheoriesrelyontheconceptofrepresentativevolumeelement(RVE),astatisticalrepresentationofmaterialproperties,whereasthestrengthandfailureisdeterminedbytheweakestdefectintheentiresampledomain.Numericalmodelingbasedonfiniteelementmethods(FEM)cancomplementanalyticalmethodsforpredictinginelasticpropertiessuchasstrengthandtoughnessmodulus(referredtoastoughness,hereafter)whichcanonlybeobtainedfromfullstress-straincurves.@#@However,numericalschemescapableofmodelingtheinitiationandpropagationofthecurvilinearcracks,suchasthecrackphasefieldmodel,arecomputationallyexpensiveandtime-consumingbecauseaveryfinemeshisrequiredtoaccommodatehighlyconcentratedstressfieldnearcracktipandtherapidvariationofdamageparameterneardiffusivecracksurface.Meanwhile,analyticalmodelsrequiresignificanthumaneffortanddomainexpertiseandfailtogeneralizetosimilardomainproblems.Inordertoidentifyhigh-performingcompositesinthemidstoflargedesignspaceswithinrealistictime-frames,weneedmodelsthatcanrapidlydescribethemechanicalpropertiesofcomplexsystemsandbegeneralizedeasilytoanalogoussystems.Machinelearningoffersthebenefitofextremelyfastinferencetimesandrequiresonlytrainingdatatolearnrelationshipsbetweeninputsandoutputse.g.,compositemicrostructuresandtheirmechanicalproperties.Machinelearninghasalreadybeenappliedtospeeduptheoptimizationofseveraldifferentphysicalsystems,includinggraphenekirigamicuts,fine-tuningsp