introductory econometrics for finance Chapter8solutions.docx
《introductory econometrics for finance Chapter8solutions.docx》由会员分享,可在线阅读,更多相关《introductory econometrics for finance Chapter8solutions.docx(13页珍藏版)》请在冰豆网上搜索。
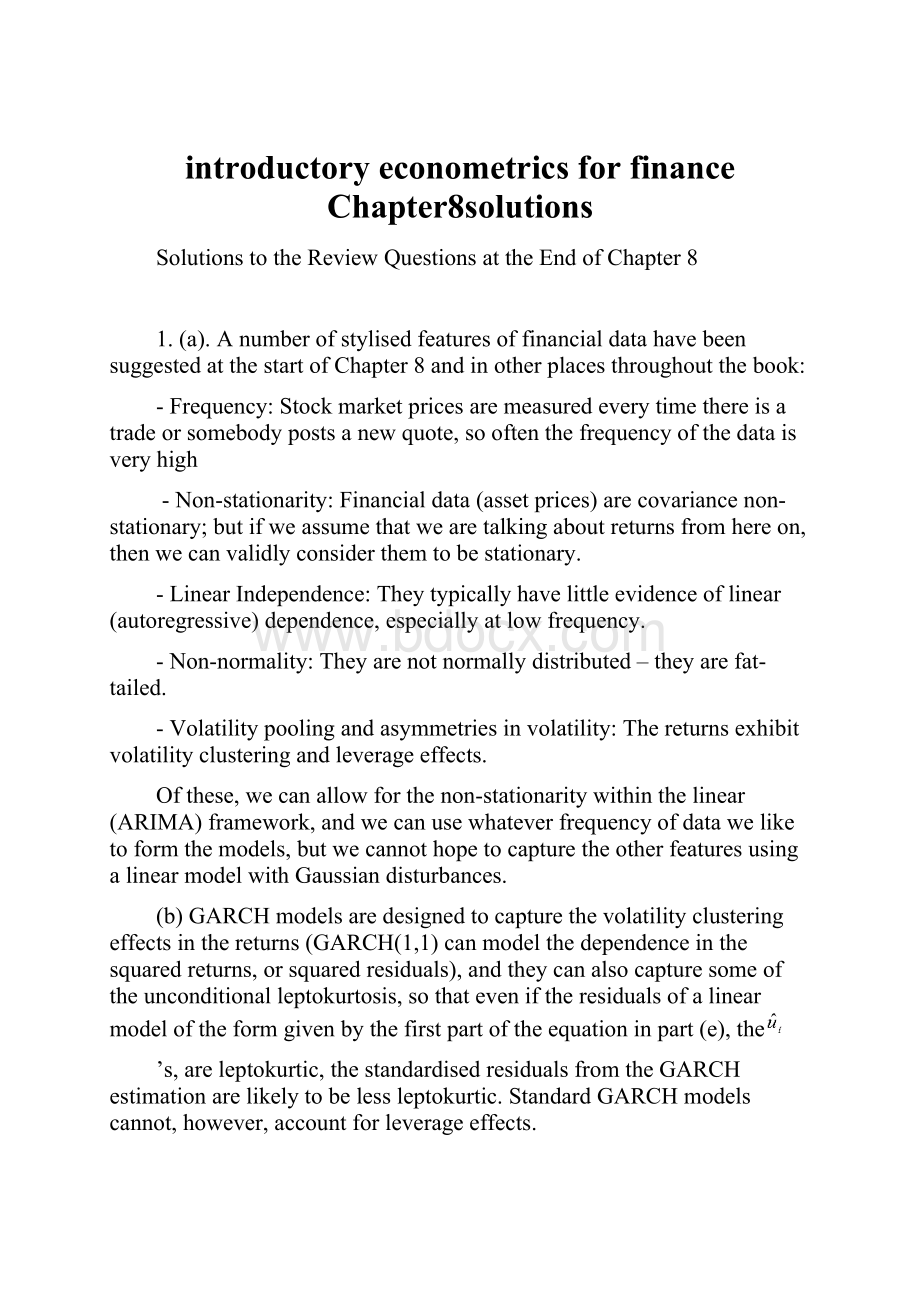
introductoryeconometricsforfinanceChapter8solutions
SolutionstotheReviewQuestionsattheEndofChapter8
1.(a).AnumberofstylisedfeaturesoffinancialdatahavebeensuggestedatthestartofChapter8andinotherplacesthroughoutthebook:
-Frequency:
Stockmarketpricesaremeasuredeverytimethereisatradeorsomebodypostsanewquote,sooftenthefrequencyofthedataisveryhigh
-Non-stationarity:
Financialdata(assetprices)arecovariancenon-stationary;butifweassumethatwearetalkingaboutreturnsfromhereon,thenwecanvalidlyconsiderthemtobestationary.
-LinearIndependence:
Theytypicallyhavelittleevidenceoflinear(autoregressive)dependence,especiallyatlowfrequency.
-Non-normality:
Theyarenotnormallydistributed–theyarefat-tailed.
-Volatilitypoolingandasymmetriesinvolatility:
Thereturnsexhibitvolatilityclusteringandleverageeffects.
Ofthese,wecanallowforthenon-stationaritywithinthelinear(ARIMA)framework,andwecanusewhateverfrequencyofdataweliketoformthemodels,butwecannothopetocapturetheotherfeaturesusingalinearmodelwithGaussiandisturbances.
(b)GARCHmodelsaredesignedtocapturethevolatilityclusteringeffectsinthereturns(GARCH(1,1)canmodelthedependenceinthesquaredreturns,orsquaredresiduals),andtheycanalsocapturesomeoftheunconditionalleptokurtosis,sothateveniftheresidualsofalinearmodeloftheformgivenbythefirstpartoftheequationinpart(e),the
’s,areleptokurtic,thestandardisedresidualsfromtheGARCHestimationarelikelytobelessleptokurtic.StandardGARCHmodelscannot,however,accountforleverageeffects.
(c)Thisisessentiallya“whichdisadvantagesofARCHareovercomebyGARCH”question.ThedisadvantagesofARCH(q)are:
-Howdowedecideonq?
-Therequiredvalueofqmightbeverylarge
-Non-negativityconstraintsmightbeviolated.
WhenweestimateanARCHmodel,werequirei>0i=1,2,...,q(sincevariancecannotbenegative)
GARCH(1,1)goessomewaytogetaroundthese.TheGARCH(1,1)modelhasonlythreeparametersintheconditionalvarianceequation,comparedtoq+1fortheARCH(q)model,soitismoreparsimonious.SincetherearelessparametersthanatypicalqthorderARCHmodel,itislesslikelythattheestimatedvaluesofoneormoreofthese3parameterswouldbenegativethanallq+1parameters.Also,theGARCH(1,1)modelcanusuallystillcaptureallofthesignificantdependenceinthesquaredreturnssinceitispossibletowritetheGARCH(1,1)modelasanARCH(),solagsofthesquaredresidualsbackintotheinfinitepasthelptoexplainthecurrentvalueoftheconditionalvariance,ht.
(d)Thereareanumberthatyoucouldchoosefrom,andtherelevantonesthatwerediscussedinChapter8,inlcudingEGARCH,GJRorGARCH-M.
Thefirsttwoofthesearedesignedtocaptureleverageeffects.Theseareasymmetriesintheresponseofvolatilitytopositiveornegativereturns.ThestandardGARCHmodelcannotcapturethese,sincewearesquaringthelaggederrorterm,andwearethereforelosingitssign.
TheconditionalvarianceequationsfortheEGARCHandGJRmodelsarerespectively
And
t2=0+1
+t-12+ut-12It-1
whereIt-1=1ifut-10
=0otherwise
Foraleverageeffect,wewouldsee>0inbothmodels.
TheEGARCHmodelalsohastheaddedbenefitthatthemodelisexpressedintermsofthelogofht,sothateveniftheparametersarenegative,theconditionalvariancewillalwaysbepositive.Wedonotthereforehavetoartificiallyimposenon-negativityconstraints.
OneformoftheGARCH-Mmodelcanbewritten
yt=+otherterms+t-1+ut,utN(0,ht)
t2=0+1
+t-12
sothatthemodelallowsthelaggedvalueoftheconditionalvariancetoaffectthereturn.Inotherwords,ourbestcurrentestimateofthetotalriskoftheassetinfluencesthereturn,sothatweexpectapositivecoefficientfor.Notethatsomeauthorsuset(i.e.acontemporaneousterm).
(e).Sinceytarereturns,wewouldexpecttheirmeanvalue(whichwillbegivenby)tobepositiveandsmall.Wearenottoldthefrequencyofthedata,butsupposethatwehadayearofdailyreturnsdata,thenwouldbetheaveragedailypercentagereturnovertheyear,whichmightbe,say0.05(percent).Wewouldexpectthevalueof0againtobesmall,say0.0001,orsomethingofthatorder.Theunconditionalvarianceofthedisturbanceswouldbegivenby0/(1-(1+2)).Typicalvaluesfor1and2are0.8and0.15respectively.Theimportantthingisthatallthreealphasmustbepositive,andthesumof1and2wouldbeexpectedtobelessthan,butcloseto,unity,with2>1.
(f)Sincethemodelwasestimatedusingmaximumlikelihood,itdoesnotseemnaturaltotestthisrestrictionusingtheF-testviacomparisonsofresidualsumsofsquares(andat-testcannotbeusedsinceitisatestinvolvingmorethanonecoefficient).Thusweshoulduseoneoftheapproachestohypothesistestingbasedontheprinciplesofmaximumlikelihood(Wald,LagrangeMultiplier,LikelihoodRatio).Theeasiestonetousewouldbethelikelihoodratiotest,whichwouldbecomputedasfollows:
1.Estimatetheunrestrictedmodelandobtainthemaximisedvalueofthelog-likelihoodfunction.
2.Imposetherestrictionbyrearrangingthemodel,andestimatetherestrictedmodel,againobtainingthevalueofthelikelihoodatthenewoptimum.NotethatthisvalueoftheLLFwillbelikelytobelowerthantheunconstrainedmaximum.
3.Thenformthelikelihoodratioteststatisticgivenby
LR=-2(Lr-Lu)2(m)
whereLrandLuarethevaluesoftheLLFfortherestrictedandunrestrictedmodelsrespectively,andmdenotesthenumberofrestrictions,whichinthiscaseisone.
4.Ifthevalueoftheteststatisticisgreaterthanthecriticalvalue,rejectthenullhypothesisthattherestrictionsarevalid.
(g)Infact,itispossibletoproducevolatility(conditionalvariance)forecastsinexactlythesamewayasforecastsaregeneratedfromanARMAmodelbyiteratingthroughtheequationswiththeconditionalexpectationsoperator.
WeknowallinformationincludingthatavailableuptotimeT.TheanswertothisquestionwillusetheconventionfromtheGARCHmodellingliteraturetodenotetheconditionalvariancebyhtratherthant2.WhatwewanttogenerateareforecastsofhT+1T,hT+2T,...,hT+sTwhereTdenotesallinformationavailableuptoandincludingobservationT.Adding1then2then3toeachofthetimesubscripts,wehavetheconditionalvarianceequationsfortimesT+1,T+2,andT+3:
hT+1=0+1
+hT
(1)
hT+2=0+1
+hT+1
(2)
hT+3=0+1
+hT+2(3)
Let
betheonestepaheadforecastforhmadeattimeT.Thisiseasytocalculatesince,attimeT,weknowthevaluesofallthetermsontheRHS.Given
howdowecalculate
thatisthe2-stepaheadforecastforhmadeattimeT?
From
(2),wecanwrite
=0+1ET(
)+
(4)
whereET(
)istheexpectation,madeattimeT,of
whichisthesquareddisturbanceterm.Themodelassumesthattheseriesthaszeromean,sowecannowwrite
Var(ut)=E[(ut-E(ut))2]=E[(ut)2].
Theconditionalvarianceofutisht,so
htt=E[(ut)2]
Turningthisargumentaround,andapplyingittotheproblemthatwehave,
ET[(uT+1)2]=hT+1
butwedonotknowhT+1,sowereplaceitwith
sothat(4)becomes
=0+1
+
=0+(1+)
Whataboutthe3-stepaheadforecast?
Bysimilararguments,
=ET(0+1
+hT+2)
=0+(1+)
=0+(1+)[0+(1+)
]
Andsoon.Thisisthemethodwecouldusetoforecasttheconditionalvarianceofyt.Ifytwere,say,dailyreturnsontheFTSE,wecouldusethesevolatilityforecastsasaninputintheBlackScholesequationtohelpdeterminetheappropriatepriceofFTSEindexoptions.
(h)Ans-stepaheadforecastfortheconditionalvariancecouldbewritten
(x)
Forthenewvalueof,thepersistenceofshockstotheconditionalvariance,givenby(1+)is0.1251+0.98=1.1051,whichisbiggerthan1.Itisobviousfromequation(x),thatanyvaluefor(1+)biggerthanonewillleadtheforecaststoexplode.Theforecastswillkeeponincreasingandwilltendtoinfinityastheforecasthorizonincreases(i.e.assincreases).Thisisobviouslyanundesirablepropertyofaforecastingmodel!
Thisiscalled“non-stationarityinvariance”.
For(1+)<1,theforecastswillconvergeontheunconditionalvarianceastheforecasthorizonincreases.For(1+)=1,knownas“integratedGARCH”orIGARCH,thereisaunitrootintheconditionalvariance,andtheforecastswillstayconstantastheforecasthorizonincreases.
2.(a)Maximumlikelihoodworksbyfindingthemostlikelyvaluesoftheparametersgiventheactualdata.Morespecifically,alog-likelihoodfunctionisformed,usuallybaseduponanormalityassumptionforthedisturbanceterms,andthevaluesoftheparametersthatmaximiseitaresought.Maximumlikelihoodestimationcanbeemployedtofindparametervaluesforbothlinearandnon-linearmodels.
(b)Thethreehypothesistestingproceduresavailablewithinthemaximumlikelihoodapproacharelagrangemultiplier(LM),likelihoodratio(LR)andWaldtests.ThedifferencesbetweenthemaredescribedinFigure8.4,andarenotdefinedagainhere.TheLagrangemultipliertestinvolvesestimationonlyunderthenullhypothesis,thelikelihoodratiotestinvolvesestimationunderboththenullandthealternativehypothesis,whiletheWaldtestinvolvesestimationonlyunderthealternative.Giventhis,itshouldbeevidentthattheLMtestwillinmanycasesbethesimplesttocomputesincetherestrictionsimpliedbythenullhypothesiswillusuallyleadtosometermscancellingouttogiveasimplifiedmode