东北大学数字图像处理实验.docx
《东北大学数字图像处理实验.docx》由会员分享,可在线阅读,更多相关《东北大学数字图像处理实验.docx(24页珍藏版)》请在冰豆网上搜索。
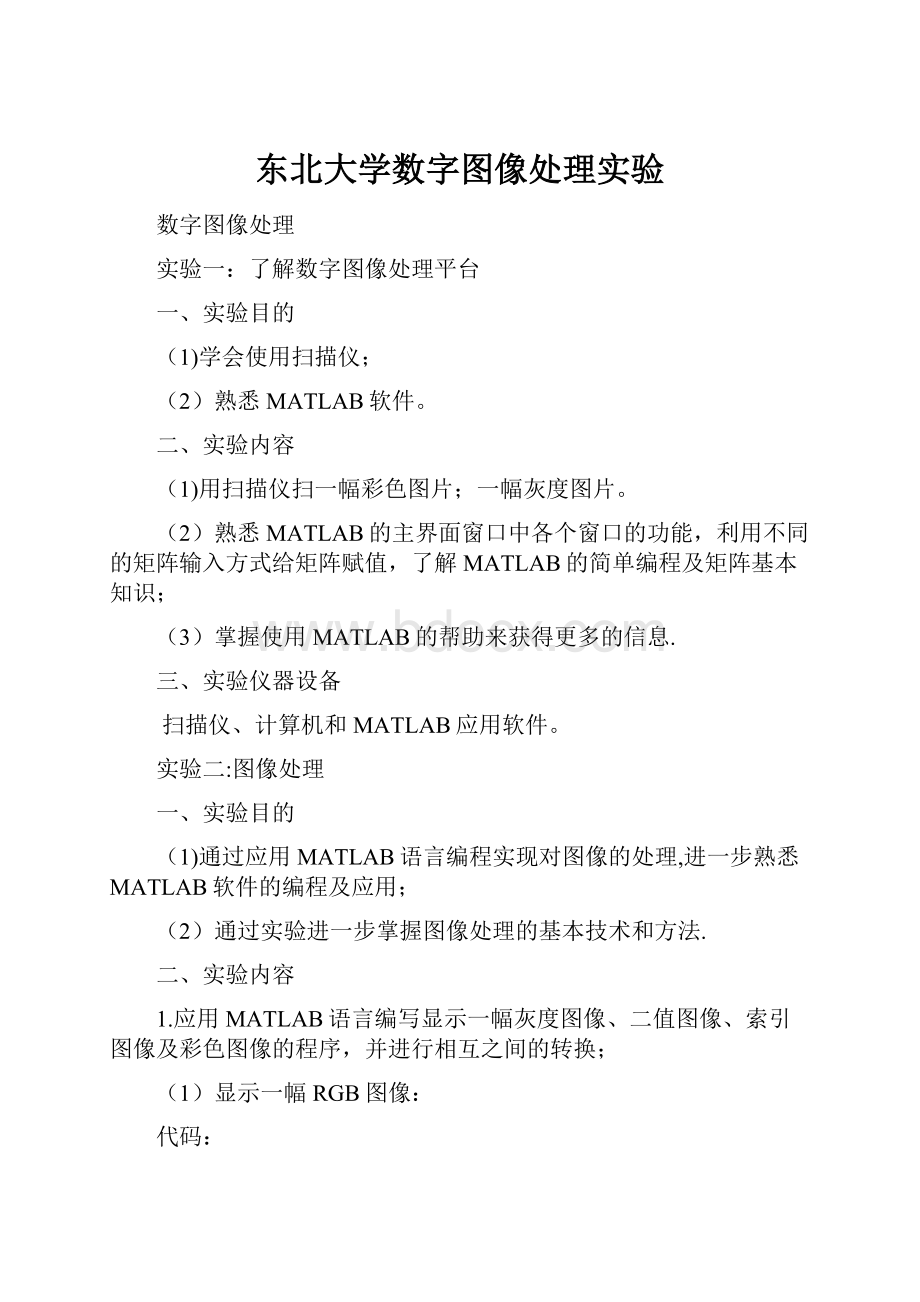
东北大学数字图像处理实验
数字图像处理
实验一:
了解数字图像处理平台
一、实验目的
(1)学会使用扫描仪;
(2)熟悉MATLAB软件。
二、实验内容
(1)用扫描仪扫一幅彩色图片;一幅灰度图片。
(2)熟悉MATLAB的主界面窗口中各个窗口的功能,利用不同的矩阵输入方式给矩阵赋值,了解MATLAB的简单编程及矩阵基本知识;
(3)掌握使用MATLAB的帮助来获得更多的信息.
三、实验仪器设备
扫描仪、计算机和MATLAB应用软件。
实验二:
图像处理
一、实验目的
(1)通过应用MATLAB语言编程实现对图像的处理,进一步熟悉MATLAB软件的编程及应用;
(2)通过实验进一步掌握图像处理的基本技术和方法.
二、实验内容
1.应用MATLAB语言编写显示一幅灰度图像、二值图像、索引图像及彩色图像的程序,并进行相互之间的转换;
(1)显示一幅RGB图像:
代码:
I=imread('cs。
jpg’);
>>imshow(I)
效果:
(2)RGB转灰度图像
代码:
>>graycs=rgb2gray(I);
>〉subplot(1,2,1);
>〉subimage(I)
〉〉subplot(1,2,2);
>〉subimage(graycs)
效果:
(3)RGB转索引图像
代码:
>〉[indcs,map]=rgb2ind(I,0。
7);
>>subplot(1,2,1)
〉〉subimage(I);
>〉subplot(1,2,2);
>>subimage(indcs,map)
效果:
(4)索引图像转RGB
代码:
>>I1=ind2rgb(indcs,map);
〉>subplot(1,2,1);
>>subimage(indcs,map);
>〉subplot(1,2,2);
>〉subimage(I1);
效果:
(5)索引图像转灰度图像:
代码:
>>i2gcs=ind2gray(indcs,map);
>>subplot(1,2,1);
>>subimage(indcs,map);
>〉subplot(1,2,2);
〉〉subimage(i2gcs,map);
效果:
(6)灰度图像转索引图像
代码:
〉>[g2ics,map]=gray2ind(graycs,64);
>〉subplot(1,2,1);
>>subimage(graycs);
>>subplot(1,2,2);
>>subimage(g2ics,map);
效果:
(7)RGB转二值图像
代码:
>>r2bwcs=im2bw(I,0。
5);
>〉subplot(1,2,1);
>>subimage(I);
>>subplot(1,2,2);
>>subimage(r2bwcs);
效果:
(8)灰度图像转二值图像
代码:
>>g2bwcs=im2bw(graycs,0.5);
〉〉subplot(1,2,1);
〉>subimage(graycs);
>>subplot(1,2,2);
>〉subimage(g2bwcs);
效果:
(9)索引图像转二值图像
代码:
〉〉i2bwcs=im2bw(indcs,map,0.7);
>>subplot(1,2,1);
〉〉subimage(indcs,map);
〉>subplot(1,2,2);
〉>subimage(i2bwcs)
效果:
2.应用MATLAB工具箱演示一幅图像的傅里叶变换、离散余弦变换,观察其频谱图.然后将它们进行逆变换,观察逆变换后的图像;
(1)傅里叶变换:
代码:
>>F=fft2(graycs);
〉>subplot(1,2,1);
〉〉subimage(graycs);
〉〉subplot(1,2,2);
>>subimage(log(abs(F)),[3,10]);
效果:
(2)傅里叶反变换:
代码:
〉>IF=ifft2(F);
〉>subplot(1,2,1);
〉〉subimage(log(abs(F)),[3,10]);
>>subplot(1,2,2);
〉〉subimage(uint8(IF));
效果:
(3)DCT变换:
代码:
>>B=dct2(graycs);
>〉subplot(1,2,1);
〉〉subimage(graycs);
〉>subplot(1,2,2);
>〉subimage(log(abs(B)),[3,5]);
效果:
(4)iDCT变换
代码:
>>iB=idct2(B);
>〉subplot(1,2,1);
>〉subimage(log(abs(B)),[3,5]);
〉>subplot(1,2,2);
>〉subimage(uint8(iB));
效果:
3.应用MATLAB语言编程来实现一幅图像的增强。
(1)取一幅灰度图像,对其进行线性点运算,即
取(α,β)分别为(1。
5,1.2)、(0。
7,1.2),对原图像进行线性处理,观察处理后的结果,并分析直方图的变化。
代码:
〉〉graycs=double(graycs);
>> graycs1=1.5*graycs+1.2;
〉〉subplot(2,2,1);
>>subimage(uint8(graycs));
〉>subplot(2,2,2);
>>imhist(uint8(graycs));
〉>subplot(2,2,3);
>>subimage(uint8(graycs1));
〉〉subplot(2,2,4);
〉>imhist(uint8(graycs1));
效果:
(2)取一幅灰度图像,对其进行直方图均衡化处理,再对其进行规定化处理,并对结果进行分析。
思考题:
如果将一幅图像进行一次直方图均衡化处理后,再进行一次直方图均衡化处理,结果会发生变化吗?
观察两次均衡化的直方图是否一样。
均衡化代码:
>〉graycs=uint8(graycs);
>〉eqcs=histeq(graycs);
〉〉subplot(2,2,1);
〉〉subimage(graycs);
>〉subplot(2,2,2);
〉>imhist(graycs);
>>subplot(2,2,3);
>>subimage(eqcs);
>>subplot(2,2,4);
>〉imhist(eqcs);
效果:
规定化代码:
〉〉hgram=50:
2:
250;
〉〉speciacs=histeq(graycs,hgram);
>〉 subplot(2,2,1);
〉>subimage(graycs);
>> subplot(2,2,2);
>〉imhist(graycs);
>〉 subplot(2,2,3);
>〉subimage(speciacs);
>> subplot(2,2,4);
〉> imhist(speciacs);
效果:
思考题:
>〉graycs=uint8(graycs);
>〉eqcs1=histeq(graycs);
>〉eqcs2=histeq(eqcs1);
〉>subplot(2,2,1);
>>subimage(eqcs1);
〉〉subplot(2,2,2);
>>imhist(eqcs1);
〉〉subplot(2,2,3);
>>subimage(eqcs2);
>>subplot(2,2,4);
>〉imhist(eqcs2);
两次均衡化结果一样.
(3)取一幅灰度图像,加入噪声后对其进行平滑滤波(均值滤波、中值滤波),并观察不同滤波方式下的效果。
代码;
〉>noisecs=imnoise(graycs,'salt & pepper’);
>〉avecs=filter2(fspecial('average',3),noisecs)/255;
〉>medcs=medfilt2(noisecs,[3,3]);
〉〉 subplot(2,2,1);
〉>subimage(graycs);
〉〉title('原图');
>>subplot(2,2,2);
>〉subimage(noisecs);
〉〉title('加噪声图’)
〉>subplot(2,2,3);
>〉subimage(avecs);
〉>title('均值平滑图’);
〉>subplot(2,2,4);
>〉subimage(medcs);
〉〉title('中值滤波图’)
效果:
均值滤波的效果与所使用的领域半径大小有关,半径越大,像素点越多,则信噪比提高越大,平滑效果越好,但是平滑图像的模糊程度越大。
中值滤波是一种非线性滤波,可以克服整形滤波器所带来的图像细节模糊,对滤波脉冲干扰及颗粒噪声最为有效.
(4)取一幅灰度图像,采用不同的算子对其进行边缘锐化,并分析结果。
思考题:
为了达到边缘锐化的反差增强效果,实际应用中将高频增强和直方图均衡化结合起来使用,这两个操作的次序能互换吗?
效果一样吗?
代码:
>>sobelcs=filter2(fspecial(’sobel'),graycs);
〉〉prewittcs=filter2(fspecial('prewitt’),graycs);
>〉laplaciancs=filter2(fspecial(’laplacian’),graycs);
>〉subplot(2,2,1);
>>subimage(graycs);
>〉title('原图');
>〉subplot(2,2,2);
>>subimage(sobelcs);
>>title('sobel狗’);
>〉subplot(2,2,3);
>>subimage(prewittcs);
〉>title(’prewitt狗’);
〉〉subplot(2,2,4);
〉〉subimage(laplaciancs);
>>title(’laplacian狗');
效果:
思考题:
不能次序交换。
因为直方图均衡化是把原始图像的灰度直方图从某个灰度区间变成在全部灰度范围内的均匀分布,而将高频增强明显的意思是对图像进行高通滤波,所以如果两个处理次序互换的话会对不同频率成分产生影响,而使效果不一样,但都可增强反差。
4.对一幅灰度图像进行模糊处理,然后进行逆滤波、维纳滤波与约束最小二乘方滤波复原实验。
代码:
>>PSF=fspecial(’motion’,30,45);
〉>blurcs=imfilter(graycs,PSF,’circular',’conv');
〉> subplot(1,2,1);
〉> subimage(graycs);
〉〉 title('原图’);
>> subplot(1,2,2);
〉> subimage(blurcs);
〉> title('运动糊化的图’);
效果:
逆滤波代码:
>〉wnr1cs=deconvwnr(blurcs,PSF);%逆滤波
>〉subplot(1,2,1);
>>subimage(blurcs);
〉〉subplot(1,2,2);
>>subimage(wnr1cs);
效果:
维纳滤波代码:
〉〉noise=0。
1*randn(size(graycs));
>〉blurnoics=imadd(blurcs,im2uint8(noise));
>> wnr2cs=deconvwnr(blurnoics,PSF);
〉〉 NSR=sum(noise(:
).^2)/sum(im2double(graycs(:
))。
^2);
>> wnr3cs=deconvwnr(blurnoics,PSF,NSR);
〉> subplot(1,3,1);
>〉subimage(blurnoics);
〉〉title(’运动加噪声狗');
〉〉 subplot(1,3,2);
〉>subimage(wnr2cs);
>〉title(’维纳滤波狗');
〉〉 subplot(1,3,3);
〉>subimage(wnr3cs);
〉〉title('真实信噪比维纳恢复狗');
5、应用MATLAB语言编写实现一幅图像的旋转、剪切和缩放;
旋转代码:
〉>rotatcs=imrotate(graycs,45,'nearest’);
〉> rotatcs1=imrotate(graycs,45,’bilinear’);
>〉 rotatcs2=imrotate(graycs,45,'bicubic');
〉〉subplot(2,2,1);
>>subimage(graycs);
〉> title(’原狗’);
>>subplot(2,2,2);
>>subimage(rotatcs);
〉〉title(’最邻域旋转狗’);
>> subplot(2,2,3);
>〉subimage(rotatcs1);
>〉title('双线性旋转狗’);
>〉 subplot(2,2,4);
〉〉subimage(rotatcs2);
>〉title(’双立方旋转狗’);
效果:
剪切代码:
>〉imshow(graycs);
>〉cropcs=imcrop(graycs,[100,100,200,200]);
>> subplot(1,2,1);
>〉imshow(graycs);
>〉title('原狗');
〉〉 subplot(1,2,2);
〉〉imshow(cropcs);
〉>title(’剪切狗');
效果:
缩放代码:
〉>minimizecs=imresize(graycs,0.2,'nearest’);
>>magnifycs=imresize(minimizecs,3,’bilinear');
〉>imshow(minimizecs);
>>imshow(magnifycs);
效果:
6. 对一幅图像进行几何失真处理后利用连接点实施图像配准复原。
代码:
>>unrealcs=imrotate(graycs,45,’bilinear’);
〉>cpselect(unrealcs,graycs);
>>input_points_corr=cpcorr(input_points2,base_points2,unrealcs,graycs);
>>Tlinear=cp2tform(input_points2,base_points2,'linearconformal’);
>>subplot(1,3,1);
>>imshow(graycs);
〉〉subplot(1,3,2);
>>imshow(unrealcs);
>〉subplot(1,3,3);
>>imshow(imtransform(unrealcs,Tlinear))
7.应用MATLAB语言编程来实现一幅图像的边缘检测。
代码:
>>csedge=edge(graycs,'canny’);
〉〉subplot(1,2,1);
〉〉subimage(graycs);
〉>subplot(1,2,2);
〉〉subimage(catedge);
>〉imshow(csedge);
效果: