华南理工大学《模式识别》研究生复习资料docx.docx
《华南理工大学《模式识别》研究生复习资料docx.docx》由会员分享,可在线阅读,更多相关《华南理工大学《模式识别》研究生复习资料docx.docx(29页珍藏版)》请在冰豆网上搜索。
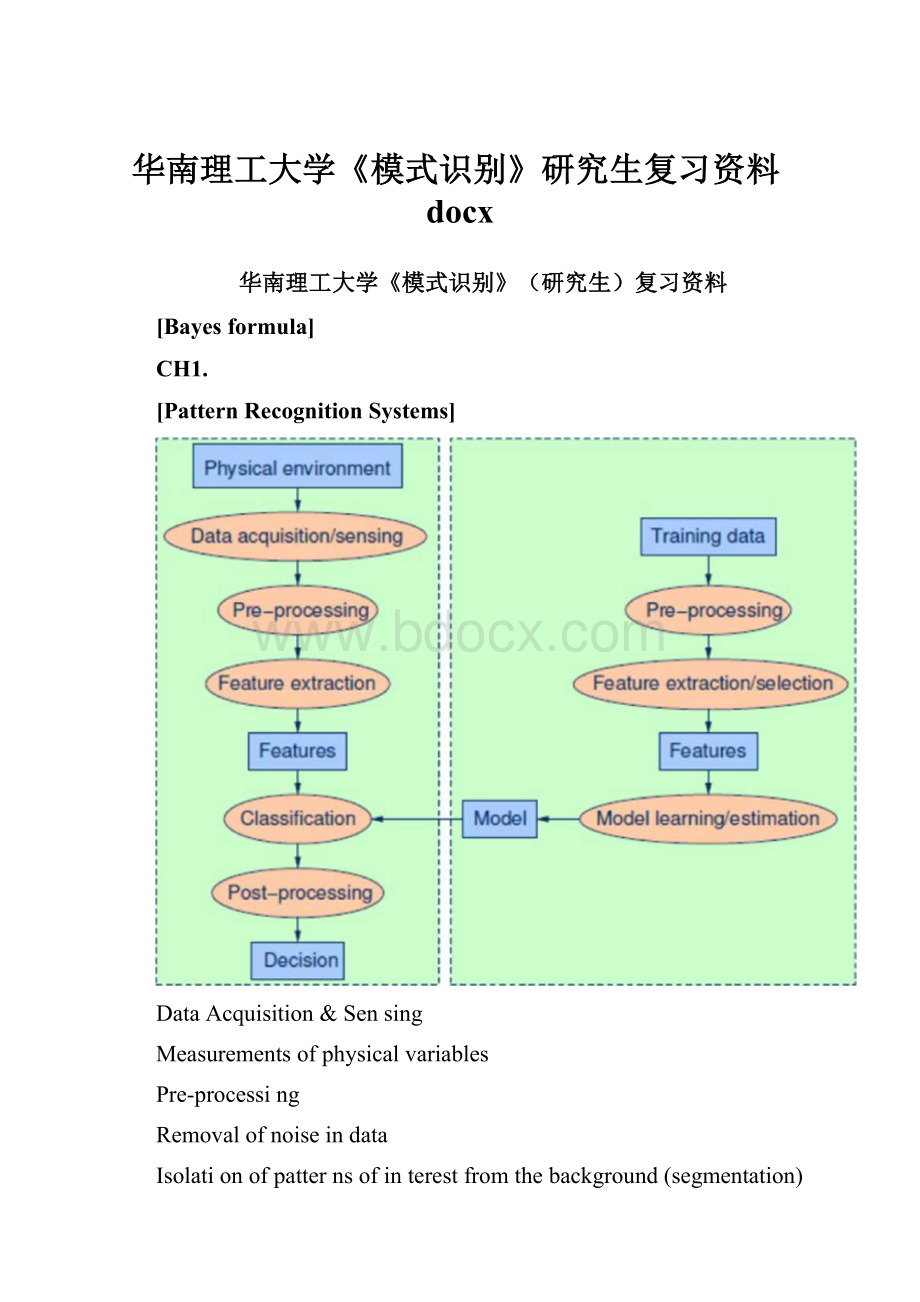
华南理工大学《模式识别》研究生复习资料docx
华南理工大学《模式识别》(研究生)复习资料
[Bayesformula]
CH1.
[PatternRecognitionSystems]
DataAcquisition&Sensing
Measurementsofphysicalvariables
Pre-processing
Removalofnoiseindata
Isolationofpatternsofinterestfromthebackground(segmentation)
Featureextraction
Findinganewrepresentationintermsoffeatures
Modellearning/estimation
Learningamappingbetweenfeaturesandpatterngroupsandcategories
Classification
Usingfeaturesandlearnedmodelstoassignapatterntoacategory
Post-processing
Evaluationofconfideneeindecisions
Exploitationofcontexttoimproveperformance
Combinationofexperts
Start
End
ELearningstrategies]
Supervisedlearning
Ateacherprovidesacategorylabelorcostforeachpatterninthetrainingset
Unsupervisedlearning
Thesystemsformsclustersornaturalgroupingoftheinputpatterns
Reinforcementlearning
Nodesiredcategoryisgivenbuttheteacherprovidesfeedbacktothesystemsuchasthedecisionisrightorwrong
[Evaluationmethods]
IndependentRun
Astatisticalmethod,alsocalledBootstrap.Repeattheexperimenttimesindependently,andtakethemeanastheresult・
Cross-validation
DatasetDisrandomlydividedintondisjointsetsDiofequalsizen/m,wherenisthenumberofsamplesinDi.Classifieristrainedmtimesandeachtimewithdifferentsetheldoutasatestingset
[BayesDecisionRule]
/Decide3iifp(3i|x)>p(o)2|x)
丁Decideo)2讦p(co2|x)>p(^i|x)
Orequivalentto
/Decidea)1ifp(x|a)1)p(d)1)>p(x|a)2)p(6o2)
VDecidea)2ifP(x|a)2)P(6O2)>p(x|co1)p(to1)
[MaximumLikelihood(ML)Rule]
Whenp(wi)=p(w2),thedecisionisbasedentirelyonthelikelihoodp(x|wj)->p(x|w)ocp(x|w)
/Decidep(尢|伽)>p(x|o)2)
/Decide6)2ifp(x|o>2)>p(x|6)i)
MultivariateNormalDensitvjnddimensions:
1_
exp--(x-Az)rS_1(x-/z)
p(x)=^4^
Where
X=(xlfx2t...,xd)T
M=(“1,“2,・・•川d)r
y=J(x-g)(x-M)7,p(x)dx
|Z|andE"1aredeterminantandinverserespectively
[MLParameterEstimation]
1十
k=l
k=l
[Erroranalysis]
Probabilityoferrorformultipassproblems:
[Discriminantfunction]
p(error|x>1-...,p(o)c|x)]
Error二BavesError+AddedError:
AddedError
BayesError
P(x|3i)p(5)dx
jp(xla)2)p((o2)dx
Ri
Ifdecisionpointisatxb,thenmin
Thediscriminantfunction
g(M=xTWiX+wtx+wi0
WhereW[=—扌为严,
-扣罗“-詢硏I
1d
+加P(5)
R2
9iM=一亍(%-丁百*(x饥(2兀)一;Zn(|2?
J)+加(P(3f))・
[Decisionboundary]
9iM=gjM
iv7(x—x0)=0wherew=阻一and
P(3f)/
In
[Lostfunction]
%弓%+幻)-(心庐*如
(闪一均)
Conditionalrisk(exoectedlossoftakingactionai):
1
PM=-=-exp
y/2na
R(q|x)=》久(如马)卩(吗伙)
7=1
Overallrisk(expectedloss):
R=J/?
(a(x)|x)p(x)dx
zero-onelossfunctionisusedtominimizetheerrorrate
[MinimumRiskDecisionRule]
Thefundamentalruleistodecidea)rif
R(aik)vR(a2lx)
[NormalDistribution]
CH3.
[Normalizeddistancefromorigintosurface]
So
[Distanceofarbitrarypointtosurface]
llwll
[PerceptronCriterion】
Usingyn6{+1,—1},allpatternsneedtosatisfy
wT0(xn)yn>0
Foreachmisclassifiedsample,PerceptronCriteriontriestominimize:
N
E(w)=-ywr0(xn)yn
n=l
[PseudoinverseMethod]
Sum-of-squared-errorfunction
JsM=l|Xw—b||2
Gradient
"s(w)=2XT(Xw—b)
NecessaryCondition
XTXw=XTb
wcanbesojveduniauRlv
怜=«収)-取“I
Problem:
乂丁X\snotalwaysnonsingular
Thesolutiondependsonb
[[ExerciseforPseudoinverseMethod]]
xandbaredefinedas
01
!
2=(1^1无2”
32b=(1111)T
Normalizeclass2samples
x‘=(jcTxyxxT
3/47/12\
-1/2-1/6
0-1/3/
Givenadatasetwith4samples,wewanttotrainalineardiscriminantfunction9(x)=wTx.Letx=/Handb=1讦class1,otherwiseb=—1.Thesum・of-squared-errorfunctionisselectedasthecriterionfunction.Findthevalueofwbypseudo-inversemethod.
index
class
1
0
1
2
0
1
1
3
1
0
2
4042
Sum-o仁squared・errorfunctionIsW=IIXw一b\\2Gradient
弘3)=2XT(Xw-b)
Sum-of-squared-errorfunction
JsM=\\Xw-b\\2Gradient
弘(w)=2XT(Xw-b)NecessaryConditionXTXw=XTbwcanbesolveduniquelyw=(XTXy1XTb
[Least-Mean-Squared(GradientDescent)]
•RecallSum-of-squared・errorfunotion
n
i=l
•Gradientfunction:
n
弘(w)=-bi)Xi
1=1
•UpdateRule:
w{k+1)=w(k)+rj(k)(bi—wTx()Xi
[LinearclassifierformultipleClasses]
One-versus-the-rest
One-versus-one
[linearlyseparableproblem]
Aproblemwhosedataofdifferentclassescanbeseparatedexactlybylineardecisionsurface.
CH4.
[Perceptionupdaterule]
w(t4-1)=w(t)+g(t)y(切ifvu(t)Tx(0y(0<0
+1)=w(t)zotherwise
(rewardandpunishmentschemes)
[[Exerciseforperception]]
Therearefourpointsinthe2・dimemsionalspace・Points(—1,0),(0,1)belongtoclassClfandpoints(0,—1),(1,0)belongtoclassC?
.Thegoalofthisexampleistodesignalinearclassifierusingtheperceptronalgorithminitsrewardandpunishmentform・Thelearningrateissetequaltoon巳andinitialweightvectorischosenasw=(0,0,0)=
w(t+1)=w(t)+“X⑴y(“ifW(t)rx(£)y(e)<0
w(t+1)=w(t)/otherwise
2.
3.
4.
7.
“(OF(0)⑴=0,w(l)=w(0)+(0)=(0)w(l)r(;)
(1)=1>0,w
(2)=w(l)
w
(2)
w(3)r0
(1)=1>0,w(5)=w(4)
w⑷
=1>0,w(4)=w(3)
w(5)r(l\
(1)=1>0,w(6)=w(5)
w⑹
(一1)=1>0,w(7)=w(6)
(-l)=-l<0,iv(3)=w
(2)
Allpatternarepresentedtothenetworkbeforelearningtakesplace
•StochasticandBatchTraining
Eachtrainingalgorithmhasstrengthanddrawback:
丁Batchlearningistypicallyslowerthanstochasticlearning.
/Stochastictrainingispreferredlargeredundanttrainingsets
[ErrorofBack-PropagationAlgorithm]
Regularizationterm
[Regularization]
Awellknownregularizationexample
J=(target—output)2/2
Updateruleforweight:
dl(w)
w(t+1)=w(t)—T]
dw
measuresthevalueofweight
Theobjectivefunctionbecomes
Minimize:
Remp+A||w||
[WeightofBack-Propagation
dj
°Wkj
{target^—outputk)fr(netk)wkj
Algorithm]
Thelearningruleforthehidden-to-outputunits:
djd]doutput比dnetk
dwkjdoutputkdnetkdwkj
Thelearningrulefortheinput-to-hiddenunits:
djdjdyjdnetj
dwjidyjdnetjdwji
Summary:
丁Hidden-to-OutputWeight
=~yjff(netk>)(/Input-to-HiddenWeight
c
瓷…f啊)为
1Lk=l
[TrainingofBack-Propagation]
Weightscanbeupdateddifferentlybypresentingthetrainingsamplesindifferentsequences.
Twopopulartrainingmethods:
StochasticTraining
Patternsarechosenrandomlyformthetrainingset(Networkweightsareupdatedrandomly)
Batchtraining
[ProblemoftrainingaNN]
Scalinginput
Targetvalues
Numberofhiddenlayers
3-layerisrecommended.Specialproblem:
morethan3
Numberofhiddenunits
roughlyn/10
Initializingweights
“Ifsetiv=0initially,learningcanneverstart・
/Standardizedatasochoosebothpositive&
negativeweights
■Ifwisinitiallytoosmall
thenetactivationofahiddenunitwillbesmallandthelinearmode/willbeimplemented
■Ifwisinitiallytoolargethehiddenunitmaysaturate(sigmoidfunctionisalways0or1)evenbeforelearningbegins
Input-to-Hidden(dinputs)
11
Hidden・to-Output(nHhiddenunits)
Weightdecay
Stochasticandbatchtraining
Stoppedtraining
Whentheerroronaseparatevalidationsetreachesaminimum
[[ExerciseforANN]]
[[ExerciseforRBF]]
ConstructaRBFnetworksuchthat
(0,0)and(1,1)aremappedto1,class(1,0)and(0,1)aremappedto0,classC2
Solution:
djf
=-(target一output)乙竝k1=1
djf
-——=—{target一output)WjXiawjiJ
reversepass:
(learningrate=0.5)
Newweightsforoutputlayer
w[=0.3一0.5*(-(0.5一0.8385)♦0.755)=0.1722
W2=0.9一0.5*(-(0.5一0.8385)*0.68)=0.7849
Newweightsforhiddenlayer
=0.1一0.5*(-(0.5一0.8385)
Wi2=0.8-0.5*(-(0.5-0.8385)
w21=0.4一0.5*(-(0.5一0.8385)
w22=0.6一0.5*(-(0.5一0.8385)
*0.1722
*0.1722
*0.7849
*0.7849
*0.35)=0.0898
*0.9)=0.7738
*0.35)=03535
*0.9)=0.4804
(/>!
(%!
,X2)=似p(-庇;;Fj,均=[l,l]r,Vi=备
。
2(衍以2)=似色=[0,°卩小2
•V00
\—/\7\—/11oofff91oo1/(X/l\/(\
0.3678
0.1353
0.3678
0.1353/10.1353
0.3678=|0.36780.3678
1I0.13531
0.3678\0.36780.3678
(2.284\
W=2.284
V-1.692/
CH5.
[StructureofRBF]
3layers:
Inputlayer:
f(x)=x
Hiddenlayer:
Gaussianfunction
Outputlayer:
linearweightsum
bias—►1
forwardpass:
g=0.8385
CH6.
[Margin]
*Marginisdefinedasthewidththattheboundarycouldbeincreasedbybeforehittingadatapoint
*Thelineardiscriminantfunction(classifier)withthemaximummarginisthebest.
*Dataclosesttothehyperplanearesupportvectors.
[CharacteristicofRBF]
Advantage:
RBFnetworktrainsfasterthanMLP
ThehiddenlayeriseasiertointerpretthanMLP
Disadvantage:
Duringthetesting,thecalculationspeedofaneuroninRBFisslowerthanMLP
[MaximumMarginClassification]
*Maximizingthemarginisgoodaccordingtointuitionandtheory.
*Impliesthatonlysupportvectorsareimportsnt;othertrainingexamplesareignorable・
Advantaae:
(comparetoLMSandperception)
Bettergeneralizationability&lessover-fitting
[Slackvariables]
llwll
[Kernels]
*WemayuseKernelfunctionstoimplicitlymaptoanewfeaturespace
Kernel:
A:
(xpx2)eR
*Kernelmustbeequivalenttoaninnerproductinsomefeaturespace
[SolvingofSVM]
*SolvingSVMisaquadraticprogrammingproblemTaraet:
maximummargin->
M=(x+-x)n
wrx++b=l=(+-yw=2
・・2...1
maximizeminimize—
/(w、+/?
)>1
Suchthat11
[NonlinearSVM]
Theoriginalfeaturespacecanalwaysbemappedtosomehigher-dimensionalfeaturespacewherethetrainingsetisseparable
[OptimizationProblem]
ff■■rminimize丄[w『z.,...
DualProblemfor2(a/isLagrangemultiplier):