4xccccc.docx
《4xccccc.docx》由会员分享,可在线阅读,更多相关《4xccccc.docx(18页珍藏版)》请在冰豆网上搜索。
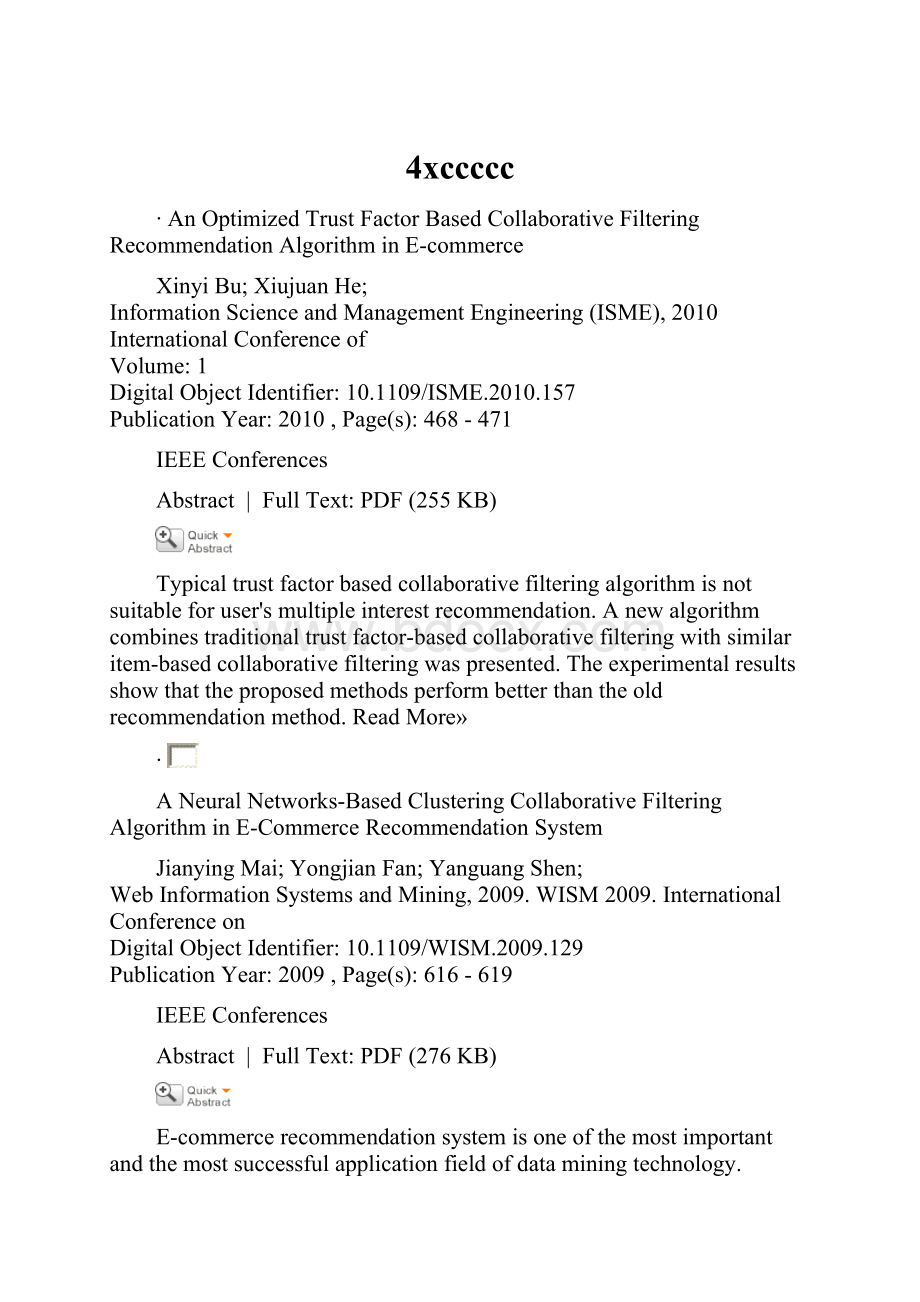
4xccccc
∙AnOptimizedTrustFactorBasedCollaborativeFilteringRecommendationAlgorithminE-commerce
XinyiBu;XiujuanHe;
InformationScienceandManagementEngineering(ISME),2010InternationalConferenceof
Volume:
1
DigitalObjectIdentifier:
10.1109/ISME.2010.157
PublicationYear:
2010,Page(s):
468-471
IEEEConferences
Abstract | FullText:
PDF (255KB)
Typicaltrustfactorbasedcollaborativefilteringalgorithmisnotsuitableforuser'smultipleinterestrecommendation.Anewalgorithmcombinestraditionaltrustfactor-basedcollaborativefilteringwithsimilaritem-basedcollaborativefilteringwaspresented.Theexperimentalresultsshowthattheproposedmethodsperformbetterthantheoldrecommendationmethod.ReadMore»
∙
ANeuralNetworks-BasedClusteringCollaborativeFilteringAlgorithminE-CommerceRecommendationSystem
JianyingMai;YongjianFan;YanguangShen;
WebInformationSystemsandMining,2009.WISM2009.InternationalConferenceon
DigitalObjectIdentifier:
10.1109/WISM.2009.129
PublicationYear:
2009,Page(s):
616-619
IEEEConferences
Abstract | FullText:
PDF (276KB)
E-commercerecommendationsystemisoneofthemostimportantandthemostsuccessfulapplicationfieldofdataminingtechnology.Recommendationalgorithmisthecoreoftherecommendationsystem.Inthispaper,aneuralnetworks-basedclusteringcollaborativefilteringalgorithmine-commercerecommendationsystemisdesigned,tryingtoestablishanclassifiermodelbasedonBPneuralnetworkforthepre-classificationtoitemsandgivingrealizationofclusteringcollaborativefilteringalgorithmandBPneuralnetworkalgorithm,andcarryingontheanalysisanddiscussiontothisalgorithmfrommultipleaspects.Thisalgorithmishelpfultoimprovesparsityproblemofcollaborativefilteringalgorithmandtoformthemoreeffectiveandthemoreaccuraterecommendationresult.ReadMore»
∙
Usingcategory-basedcollaborativefilteringintheActiveWebMuseum
Kohrs,A.;Merialdo,B.;
MultimediaandExpo,2000.ICME2000.2000IEEEInternationalConferenceon
Volume:
1
DigitalObjectIdentifier:
10.1109/ICME.2000.869613
PublicationYear:
2000,Page(s):
351-354vol.1
IEEEConferences
Abstract | FullText:
PDF (488KB)
Collaborativefilteringisanimportanttechnologyforcreatinguser-adaptingWebsites.Ingeneraltheeffortsofimprovingfilteringalgorithmsandusingthepredictionsforthepresentationoffilteredobjectsaredecoupled.Therefore,commonmeasures(ormetrics)forevaluatingcollaborativefiltering(recommender)systemsfocusmainlyonthepredictionalgorithm.Itishardtorelatetheclassicmeasurementstoactualusersatisfactionbecauseofthewaytheuserinteractswiththerecommendations,determinedbytheirrepresentation,influencesthebenefitsfortheuser.Weproposeanabstractaccessparadigm,whichcanbeappliedtothedesignoffilteringsystems,andatthesametimeformalizestheaccesstofilteringresultsviamulti-corridors(basedoncontent-basedcategories).Thisleadstonewmeasureswhichbetterrelatetotheusersatisfaction.Weusethesemeasurestoevaluatetheuseofvariouskindsofmulti-corridorsforourprototypeuser-adaptingWebsite,theActiveWebMuseumReadMore»
∙
AScalableCollaborativeFilteringBasedRecommenderSystemUsingIncrementalClustering
Chakraborty,P.S.;
AdvanceComputingConference,2009.IACC2009.IEEEInternational
DigitalObjectIdentifier:
10.1109/IADCC.2009.4809245
PublicationYear:
2009,Page(s):
1526-1529
IEEEConferences
Abstract | FullText:
PDF (2491KB)
RecommendersystemshelptoovercometheproblemofinformationoverloadontheInternetbyprovidingpersonalizedrecommendationstotheusers.Content-basedfilteringandcollaborativefilteringareusuallyappliedtopredicttheserecommendations.Amongthesetwo,Collaborativefilteringisthemostcommonapproachfordesigninge-commercerecommendersystems.TwomajorchallengesforCFbasedrecommendersystemsarescalabilityandsparsity.Inthispaperwepresentanincrementalclusteringapproachtoimprovethescalabilityofcollaborativefiltering.ReadMore»
∙
MemeticCollaborativeFilteringBasedRecommenderSystem
Banati,H.;Mehta,S.;
InformationTechnologyforRealWorldProblems(VCON),2010SecondVaagdeviInternationalConferenceon
DigitalObjectIdentifier:
10.1109/VCON.2010.28
PublicationYear:
2010,Page(s):
102-107
IEEEConferences
Abstract | FullText:
PDF (296KB)
WebbasedDecisionSupportsystemslikerecommendationsystemshavebecomeeffectivetoolsfordecisionmakingintherecentpast.Howevertherecommendersystemsemployingconventionalclusteringtechniques(KRS)likeK-Meansforcollaborativefiltering,sufferfromthelimitationofgettinglocaloptimumresults.ThispaperpresentsMemeticRecommenderSystem(MRS)basedonthecollaborativebehaviorofmemes.MemeticAlgorithms(MAs)areconsideredasoneofthemostsuccessfulapproachesforcombinatorialoptimization.MAsarethegeneticalgorithmswhichincorporatelocalsearchintheevolutionaryscheme.Weproposeadistinctivestrategytoperformlocalsearchinmemeticalgorithms.MRSworksin2phases-InthefirstphaseamodelisdevelopedbasedonMemeticClusteringalgorithmandinthesecondphasetrainedmodelisusedtopredictrecommendationsfortheactiveuser.RigorousexperimentswereconductedtoprovethedecisionsupportandstatisticalefficacyofMRSvisavisKRS.Resultsconfirmedthattheproposedapproachyieldsmuchbetterperformanceascomparedtotheconventionalcollaborativefilteringrecommendersystem.ReadMore»
∙
SyncretizingContextInformationintotheCollaborativeFilteringRecommendation
RuliangXiao;FaliangHong;JinboXiong;XiaojianZheng;ZhengqiuZhang;
DatabaseTechnologyandApplications,2009FirstInternationalWorkshopon
DigitalObjectIdentifier:
10.1109/DBTA.2009.57
PublicationYear:
2009,Page(s):
33-36
IEEEConferences
Abstract | FullText:
PDF (357KB)
Socialnetworkallowsuserstoorganizecollectionsofresourcesonthewebinacollaborativefashion.Collaborativefilteringasaclassicalmethodhasbeenalsousedinhelpingpeopletodealwithinformationoverloadinfolksonomysystem.Theproblemofdevisingmethodstosolvethecontextualproblemsemergingintheprocessofrecommendationapplicationoverthesocialnetworkisincreasingopen.Hereweproposeanovelmeanstosyncretizecontextinformationintotherecommendersystem.Thispaperfirstrecalltraditionalmethodsofcollaborativefiltering,thenpresentssomedefinitionsandalgorithmframework,proposesacontextualratingestimation.Finally,experimentcomparisondemonstratesthatthecontextualapproachcanproducesbetterratingestimations.ReadMore»
∙
AddressingInterestDiversityinP2PBasedCollaborativeSpamFiltering
FangWeidong;DongShoubin;
GridandCooperativeComputingWorkshops,2006.GCCW'06.FifthInternationalConferenceon
DigitalObjectIdentifier:
10.1109/GCCW.2006.16
PublicationYear:
2006,Page(s):
163-169
IEEEConferences
Abstract | FullText:
PDF (271KB)
CollaborativeinformationfilteringtendstobeapromisingtechnologyinthefightagainInternetspam,andthepeer-to-peerframeworkisbelievedtobemoresuitabletoimplementthiscollaborationcomparedtocentralizedones.However,theassumptionofuniforminformationinterestsacrosspeersincollaborativefilteringlimitstheimprovementinfilteringaccuracyandincreasesnetworktrafficunnecessarily.Toaddressthisproblem,weproposetoconstructmultipleinterestgroupsforapeer,eachcorrespondingtoonemessagecategory;andtouseapercolationsearchalgorithmtoretrievemessagefeedbacksinscale-freeemailnetworks.ExperimentsshowtheproposedmodelcangetremarkableimprovementinfalsepositivereductionandgoodperformanceinspamfeedbackretrievalReadMore»
∙
LearningtheSpectrumviaCollaborativeFilteringinCognitiveRadioNetworks
HushengLi;
NewFrontiersinDynamicSpectrum,2010IEEESymposiumon
DigitalObjectIdentifier:
10.1109/DYSPAN.2010.5457847
PublicationYear:
2010,Page(s):
1-12
IEEEConferences
Abstract | FullText:
PDF (336KB)
Secondaryusersincognitiveradionetworksneedtolearnthestatisticsofspectruminordertoachieveefficientcommunications.Duetothespatialcorrelation,theefficiencyoflearningisimprovedbylettingsecondaryuserscollaborateandexchangeinformation.DuetothesimilaritybetweenthecollaborativelearningincognitiveradionetworksandtherecommendationsystemsofelectroniccommercelikeAmazon,thetechniqueofcollaborativefilteringisapplied.Predictionorientedandrewardorientedcriteriaareproposedtoderivetheprocedureofcollaborativefiltering.Fortheformercriterion,linearpredictionisusedfortheparameterestimation,heuristicmetricisderivedforchannelselection,andsimilaritybasedBoltzmandistributionisusedforcollaboratorselection.Forthelattercriterion,thetechniqueofmulti-armedbanditisappliedtomaximizethetotalrewardofspectrumaccess.Numericalsimulationshowsthattheproposedcollaborativefilteringschemecansignificantlyimprovetheperformanceofspectrumlearning.ReadMore»
∙
AStudyontheImprovedCollaborativeFilteringAlgorithmforRecommenderSystem
HeeChoonLee;SeokJunLee;YoungJunChung;
SoftwareEngineeringResearch,Management&Applications,2007.SERA2007.5thACISInternationalConferenceon
DigitalObjectIdentifier:
10.1109/SERA.2007.33
PublicationYear:
2007,Page(s):
297-304
IEEEConferences
Abstract | FullText:
PDF (314KB)
Thepurposeofthisstudyistosuggestanal