如何写摘要.docx
《如何写摘要.docx》由会员分享,可在线阅读,更多相关《如何写摘要.docx(11页珍藏版)》请在冰豆网上搜索。
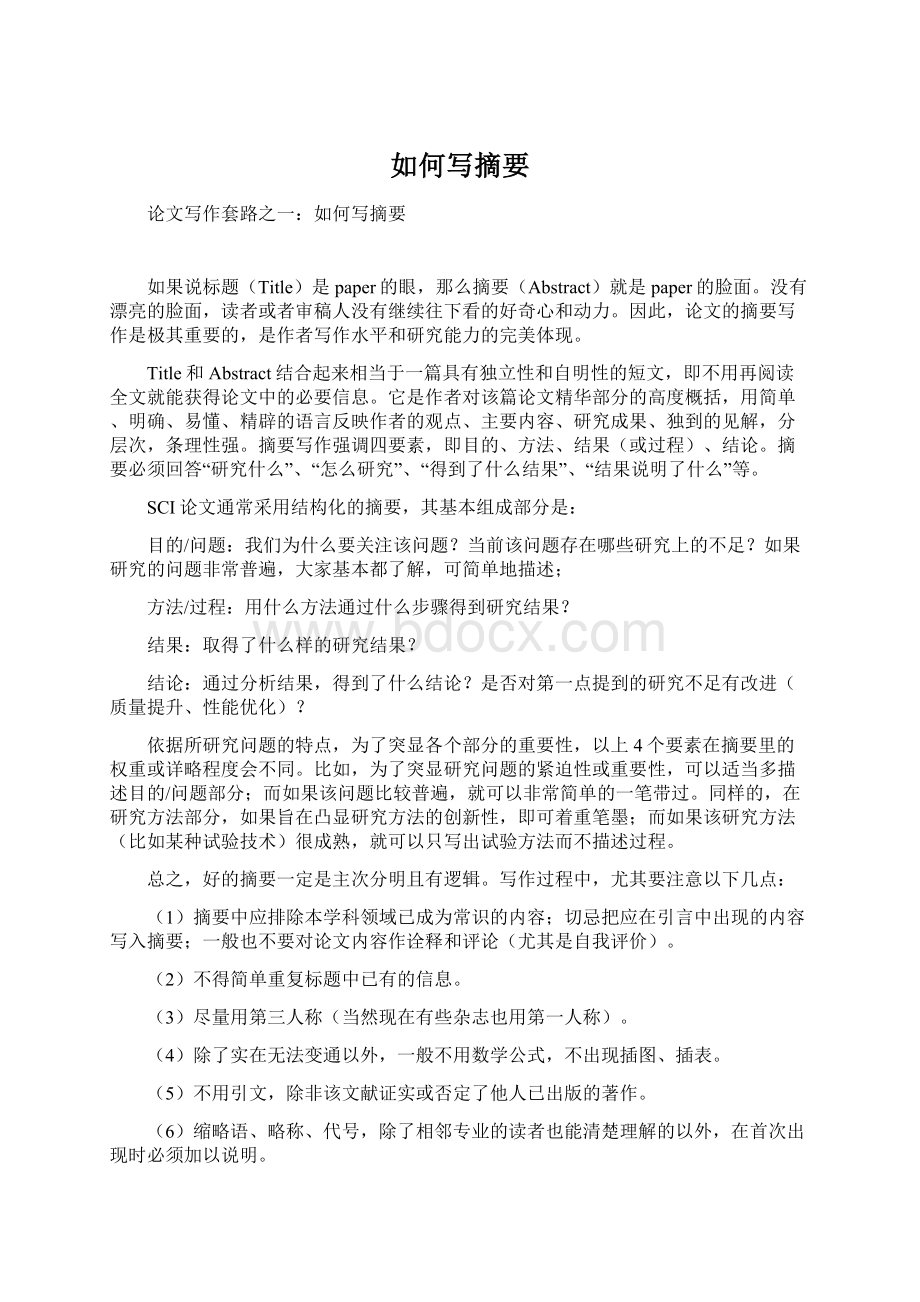
如何写摘要
论文写作套路之一:
如何写摘要
如果说标题(Title)是paper的眼,那么摘要(Abstract)就是paper的脸面。
没有漂亮的脸面,读者或者审稿人没有继续往下看的好奇心和动力。
因此,论文的摘要写作是极其重要的,是作者写作水平和研究能力的完美体现。
Title和Abstract结合起来相当于一篇具有独立性和自明性的短文,即不用再阅读全文就能获得论文中的必要信息。
它是作者对该篇论文精华部分的高度概括,用简单、明确、易懂、精辟的语言反映作者的观点、主要内容、研究成果、独到的见解,分层次,条理性强。
摘要写作强调四要素,即目的、方法、结果(或过程)、结论。
摘要必须回答“研究什么”、“怎么研究”、“得到了什么结果”、“结果说明了什么”等。
SCI论文通常采用结构化的摘要,其基本组成部分是:
目的/问题:
我们为什么要关注该问题?
当前该问题存在哪些研究上的不足?
如果研究的问题非常普遍,大家基本都了解,可简单地描述;
方法/过程:
用什么方法通过什么步骤得到研究结果?
结果:
取得了什么样的研究结果?
结论:
通过分析结果,得到了什么结论?
是否对第一点提到的研究不足有改进(质量提升、性能优化)?
依据所研究问题的特点,为了突显各个部分的重要性,以上4个要素在摘要里的权重或详略程度会不同。
比如,为了突显研究问题的紧迫性或重要性,可以适当多描述目的/问题部分;而如果该问题比较普遍,就可以非常简单的一笔带过。
同样的,在研究方法部分,如果旨在凸显研究方法的创新性,即可着重笔墨;而如果该研究方法(比如某种试验技术)很成熟,就可以只写出试验方法而不描述过程。
总之,好的摘要一定是主次分明且有逻辑。
写作过程中,尤其要注意以下几点:
(1)摘要中应排除本学科领域已成为常识的内容;切忌把应在引言中出现的内容写入摘要;一般也不要对论文内容作诠释和评论(尤其是自我评价)。
(2)不得简单重复标题中已有的信息。
(3)尽量用第三人称(当然现在有些杂志也用第一人称)。
(4)除了实在无法变通以外,一般不用数学公式,不出现插图、插表。
(5)不用引文,除非该文献证实或否定了他人已出版的著作。
(6)缩略语、略称、代号,除了相邻专业的读者也能清楚理解的以外,在首次出现时必须加以说明。
(7)切忌不可将摘要、序、结论写的一样。
(8)摘要、引言、结论三者之间的关系:
①“摘要”应有结论性的语言;②“引言”不应出现结论性的语言;③“结论”不应出现背景介绍、论文主要分析部分。
这里我选择8篇关于机器学习的论文摘要进行对比,分析他们是怎么写的。
下面来看例子:
1)ConvolutionalPoseMachines(论文标题)
PoseMachinesprovideasequentialpredictionframeworkforlearningrichimplicitspatialmodels.
本摘要没有过多的铺垫,直奔主题,第一句首先提到了姿态机。
Inthisworkweshowasystematicdesignforhowconvolutionalnetworkscanbeincorporatedintotheposemachineframeworkforlearningimagefeaturesandimage-dependentspatialmodelsforthetaskofposeestimation.
然后直接说明本文的意图,即介绍如何设计卷积神经网络,将其包括到姿态机这个框架中来学习图像的特征和依赖图像的空间模型进而实现姿态估计。
Thecontributionofthispaperistoimplicitlymodellong-rangedependenciesbetweenvariablesinstructuredpredictiontaskssuchasarticulatedposeestimation.
其后直接说出本文的贡献:
即隐式地对长范围的依赖进行建模。
Weachievethisbydesigningasequentialarchitecturecomposedofconvolutionalnetworksthatdirectlyoperateonbeliefmapsfrompreviousstages,producingincreasinglyrefinedestimatesforpartlocations,withouttheneedforexplicitgraphicalmodel-styleinference.
而后说出本文具体是怎么做的:
通过设计包含CNN的网络结构,该网络结构能够在前一阶段的beliefmap的结果之上进行,这样可以逐渐地得到经过精化之后的身体部件的位置,这种方式不需要显式地进行图模型建模。
Ourapproachaddressesthecharacteristicdifficultyofvanishinggradientsduringtrainingbyprovidinganaturallearningobjectivefunctionthatenforcesintermediatesupervision,therebyreplenishingback-propagatedgradientsandconditioningthelearningprocedure.Wedemonstratestate-of-the-artperformanceandoutperformcompetingmethodsonstandardbenchmarksincludingtheMPII,LSP,andFLICdatasets.
最后给出了本文方法所强调要解决的问题,并且说明本方法NB,stateofartperformance。
点评:
这种写法输入一般套路写法
1首先简单铺垫;
2本文的大体意图;
3一句话说明贡献;
4一句话说明具体是怎么做的
⑤最后给出本文所的方法所解决的困难是什么,本文方法在xxx数据集上取得了stateofart结果
可以使用的语法结构:
你方法的大体意图
inthisworkwoshowasystematicdesignforxxxx
介绍你的贡献
thecontributionofthispaperistoxxx
用于介绍你自己的方法
weachievethisbyxxxx
说明你方法NB
ourapproachaddressxxxproblembyxxxxx.Wedemonstratestate-of-the-artperformanceandoutperformcompetingmethodsonstandardbenchmarksincludingthexxxx
2)End-to-EndLearningofDeformableMixtureofPartsandDeepConvolutionalNeuralNetworksforHumanPoseEstimation
Recently,DeepConvolutionalNeuralNetworks(DCNNs)havebeenappliedtothetaskofhumanposeestimation,andhaveshownitspotentialoflearningbetterfeaturerepresentationsandcapturingcontextualrelationships.
首先介绍CNN用于人的姿态估计,并且能够捕获好的特征。
However,itisdifficulttoincorporatedomainpriorknowledgesuchasgeometricrelationshipsamongbodypartsintoDCNNs.
引出要解决的问题:
难以包含领域先验知识,比如身体部件之间的几何关系到CNN中
Inaddition,trainingDCNN-basedbodypartdetectorswithoutconsiderationofglobalbodyjointconsistencyintroducesambiguities,whichincreasesthecomplexityoftraining.
进一步突出要解决的问题重要性:
即如果不考虑身体部件之间的关系会导致引入歧义,增加模型训练复杂度
Inthispaper,weproposeanovelend-to-endframeworkforhumanposeestimationthatcombinesDCNNswiththeexpressivedeformablemixtureofparts.
接着提出本文的方法:
端到端的框架,能够将CNN和可变部件模型结合起来
Weexplicitlyincorporatedomainpriorknowledgeintotheframework,whichgreatlyregularizesthelearningprocessandenablestheflexibilityofourframeworkforloopymodelsortree-structuredmodels.
具体介绍本文方法如何做的:
显式地将领域先验知识加入到框架,这样做能够对学习过程起到正则化的作用,保证框架的灵活性
TheeffectivenessofjointlylearningaDCNNwithadeformablemixtureofpartsmodelisevaluatedthroughintensiveexperimentsonseveralwidelyusedbenchmarks.
Theproposedapproachsignificantlyimprovestheperformancecomparedwithstate-of-the-artapproaches,especiallyonbenchmarkswithchallengingarticulations.
说明方法的结果:
在若干个benchmarks上NB,又是stateofart…….
点评:
一般套路写法
1铺垫
2引出要解决的问题,突触要解决的问题的重要性
3一句话介绍你的方法
4一句话介绍具体如何做的
⑤你的方法NB,在xxx数据集行stateofart
善用连词:
一般用在引出你所要解决的问题上
However
用于进一步强调如果不解决这个问题会怎么样
Inaddition
用于介绍你自己的方法
inthispaperweproposeanovelend-toendframeworkforxxxxthatxxxxxxxx
说明你方法NB
Theeffectivenessofxxxxxisevaluatedthrougthintensiveexperimentsonxxxxbenchmarks,theproposedapproachsignificantlyimprovestheperformancecomparedwithstate-of-the-artapproaches
3)HumanPoseEstimationwithIterativeErrorFeedback
HierarchicalfeatureextractorssuchasConvolutionalNetworks(ConvNets)haveachievedimpressiveperformanceonavarietyofclassificationtasksusingpurelyfeedforwardprocessing.
铺垫:
CNN能够提取层次特征
Feedforwardarchitecturescanlearnrichrepresentationsoftheinputspacebutdonotexplicitlymodeldependenciesintheoutputspaces,thatarequitestructuredfortaskssuchasarticulatedhumanposeestimationorobjectsegmentation.
引出要解决的问题:
前馈结构可以学习到输入空间的丰富的表达但是不能显式地建模在输出空间的依赖关系。
Hereweproposeaframeworkthatexpandstheexpressivepowerofhierarchicalfeatureextractorstoencompassbothinputandoutputspaces,byintroducingtop-downfeedback.
提出本文的方法:
提出一种扩展层次特征提取器的表达能力的方法即将通过引入top-down的反馈将输入和输出空间都包括进来
Insteadofdirectlypredictingtheoutputsinonego,weuseaself-correctingmodelthatprogressivelychangesaninitialsolutionbyfeedingbackerrorpredictions,inaprocesswecallIterativeErrorFeedback(IEF).
进一步突出本文方法的区别:
使用自矫正模型,通过将错误预测的无法反馈回去,渐进地改变初始解,本文称之为IEF
IEFshowsexcellentperformanceonthetaskofarticulatedposeestimationinthechallengingMPIIandLSPbenchmarks,matchingthestate-of-the-artwithoutrequiringgroundtruthscaleannotation.
说明本文方法NB:
IEFNB
点评:
一般套路写法
1一句话铺垫
2引出要解决的问题
3提出本文方法
4突出本文方法的区别
5突出自己方法NB
可用的语法结构:
用于引出要解决的问题
xxxxisgoodbutdonotxxxxx
提出本文方法
Hereweproposeaframeworkthatxxxx,byxxxxx
突出本文方法
Insteadofxxx,weusexxxx
突出自己方法NB
Ourmethodshowexcellentperformanceonthetaskofxxxinthechallengingxxxandxxxbenchmarks
4)PersonalizingHumanVideoPoseEstimation
WeproposeapersonalizedConvNetposeestimatorthatautomaticallyadaptsitselftotheuniquenessofaperson’sappearancetoimproveposeestimationinlongvideos.
没有铺垫,直接提出本文方法:
提出了个性化的卷积神经网络姿态估计器,该方法能够自动地将其适配到某个人的外形上去,从而改善长时间段的视频中的人的姿态估计性能
Wemakethefollowingcontributions:
(i)weshowthatgivenafewhigh-precisionposeannotations,e.g.fromagenericConvNetposeestimator,additionalannotationscanbegeneratedthroughoutthevideousingacombinationofimage-basedmatchingfortemporallydistantframes,anddenseopticalflowfortemporallylocalframes;
(ii)wedevelopanocclusionawareself-evaluationmodelthatisabletoautomaticallyselectthehigh-qualityandrejecttheerroneousadditionalannotations;
(iii)wedemonstratethatthesehigh-qualityannotationscanbeusedtofine-tuneaConvNetposeestimatorandtherebypersonalizeittolockontokeydiscriminativefeaturesoftheperson’sappearance.
直接说出本文贡献:
三个点
TheoutcomeisasubstantialimprovementintheposeestimatesforthetargetvideousingthepersonalizedConvNetcomparedtotheoriginalgenericConvNet.
本文方法NB
Ourmethodoutperformsthestateoftheart(includingtopConvNetmethods)byalargemarginontwostandardbenchmarks,aswellasonanewchallengingYouTubevideodataset.Furthermore,weshowthattrainingfromtheautomaticallygeneratedannotationscanbeusedtoimprovetheperformanceofagenericConvNetonotherbenchmarks.
本文方法还是NB
点评:
直奔主题的写法套路
1提出本文方法
2给出本文贡献,逐个列出来
3强调本文方法NB
可用的语法结构:
提出本文方法:
Weproposeaxxxthatxxxx
给出本文贡献
Wemakethefollowingcontributions:
(i)weshowthatxxx
(ii)wedevelopaxxx
(iii)wedemonstratethatxxx
本文方法NB
Ourmethodoutperformsthestateoftheartbyalargemarginontwostandardbenchmarks,aswellasonanewchallengingYouTubevideodataset
5)StructuredFeatureLearningforPoseEstimation
Inthispaper,weproposeastructuredfeaturelearningframeworktoreasonthecorrelationsamongbodyjointsatthefeaturelevelinhumanposeestimation.
提出本文方法:
结构化特征学习框架用于在特征层次上推断关节点之间的关系
Differentfromexistingapproachesofmodelingstructuresonscoremapsorpredictedlabels,featuremapspreservesubstantiallyricherdescriptionsofbodyjoints.
强调本文方法的区别:
现有方法都是对在scoremap或者预测类标上的结构进行建模,而featuremap则保留了大量更加丰富的关节描述信息
Therelationshipsbetweenfeaturemapsofjointsarecapturedwiththeintroducedgeometricaltransformkernels,whichcanbeeasilyimplementedwithaconvolutionlayer.
Featuresandtheirrelationshipsarejointlylearnedinanend-to-endlearningsystem.Abi-directionaltreestructuredmodelisproposed,sothatthefeaturechannelsatabodyjointcanwellreceiveinformationfromotherjoints.
本文方法具体怎么做
Theproposedframeworkimprovesfeaturelearningsubstantially.Withverysimplepostprocessing,itreachesthebestmeanPCPontheLSPandFLICdatasets.ComparedwiththebaselineoflearningfeaturesateachjointseparatelywithConvNet,themeanPCPhasbeenimprovedby18%onFLIC.Thecodeisreleasedtothepublic.
本文方法NB
点评:
直奔主题的写法套路
1提出本文方法
2强调本文方法的区别
3本文方法具体怎么做
4本文方法NB
可用的语法结构:
提出本文方