大数据外文翻译参考文献综述文档格式.docx
《大数据外文翻译参考文献综述文档格式.docx》由会员分享,可在线阅读,更多相关《大数据外文翻译参考文献综述文档格式.docx(15页珍藏版)》请在冰豆网上搜索。
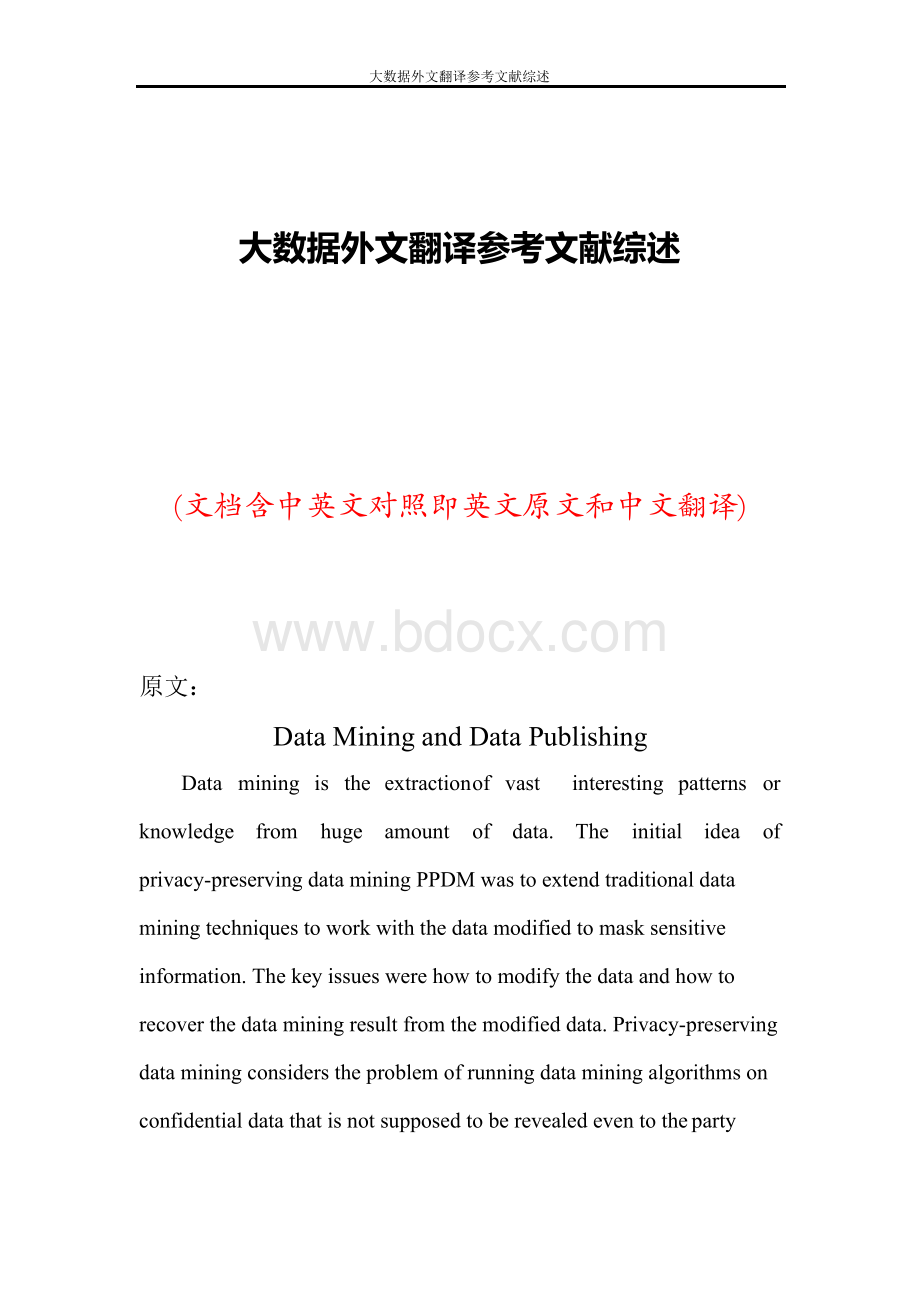
大数据外文翻译参考文献综述@#@大数据外文翻译参考文献综述@#@(文档含中英文对照即英文原文和中文翻译)@#@原文:
@#@@#@@#@DataMiningandDataPublishing@#@Data miningisthe extraction ofvast interestingpatterns orknowledge from huge amount of data. The initial idea ofprivacy-preservingdataminingPPDMwastoextendtraditionaldataminingtechniquestoworkwiththedatamodifiedtomasksensitiveinformation.Thekeyissueswerehowtomodifythedataandhowtorecoverthedataminingresultfromthemodifieddata.Privacy-preservingdataminingconsiderstheproblemofrunningdataminingalgorithmsonconfidentialdatathatisnotsupposedtoberevealedeventotheparty@#@runningthealgorithm.Incontrast,privacy-preservingdatapublishing(PPDP)maynotnecessarilybetiedtoaspecificdataminingtask,andthedataminingtaskmaybeunknownatthetimeofdatapublishing.PPDPstudieshowtotransformrawdataintoaversionthatisimmunizedagainstprivacyattacksbutthatstillsupportseffectivedataminingtasks.Privacy-preservingforbothdatamining(PPDM)anddatapublishing(PPDP)hasbecomeincreasinglypopularbecauseitallowssharingofprivacysensitivedataforanalysispurposes.Onewellstudiedapproachisthek-anonymitymodel[1]whichinturnledtoothermodelssuchasconfidencebounding,l-diversity,t-closeness,(α,k)-anonymity,etc.Inparticular,allknownmechanismstrytominimizeinformationlossandsuchanattemptprovidesaloopholeforattacks.Theaimofthispaperistopresentasurveyformostofthecommonattackstechniquesforanonymization-basedPPDM&@#@PPDPandexplaintheireffectsonDataPrivacy.@#@Althoughdataminingispotentiallyuseful,manydataholdersarereluctanttoprovidetheirdatafordataminingforthefearofviolatingindividualprivacy.Inrecentyears,studyhasbeenmadetoensurethatthesensitiveinformationofindividualscannotbeidentifiedeasily.@#@AnonymityModels,k-anonymizationtechniqueshavebeenthe@#@focusofintenseresearchinthelastfewyears.Inordertoensureanonymizationofdatawhileatthesametimeminimizingtheinformation@#@lossresultingfromdatamodifications,everalextendingmodelsareproposed,whicharediscussedasfollows.@#@1.k-Anonymity@#@k-anonymityisoneofthemostclassicmodels,whichtechniquethatpreventsjoiningattacksbygeneralizingand/orsuppressingportionsofthereleasedmicrodatasothatnoindividualcanbeuniquelydistinguishedfromagroupofsizek.Inthek-anonymoustables,adatasetisk-anonymous(k≥1)ifeachrecordinthedatasetisin -distinguishablefromatleast(k.1)otherrecordswithinthesamedataset.Thelargerthevalueofk,thebettertheprivacyisprotected.k-anonymitycanensurethatindividualscannotbeuniquelyidentifiedbylinkingattacks.@#@2.ExtendingModels@#@Sincek-anonymitydoesnotprovidesufficientprotectionagainstattributedisclosure.Thenotionofl-diversityattemptstosolvethisproblem byrequiring that each equivalence class has at least lwell-representedvalueforeachsensitiveattribute.Thetechnologyofl-diversityhassomeadvantagesthank-anonymity.Becausek-anonymitydatasetpermitsstrongattacksduetolackofdiversityinthesensitiveattributes.Inthismodel,anequivalenceclassissaidtohavel-diversityifthereareatleastlwell-representedvalueforthesensitiveattribute.Becausetherearesemanticrelationshipsamongtheattributevalues,anddifferent values have very different levels of sensitivity. After@#@anonymization,inanyequivalenceclass,thefrequency(infraction)ofasensitivevalueisnomorethanα.@#@3.RelatedResearchAreas@#@Severalpollsshowthatthepublichasanin-creasedsenseofprivacyloss.Sincedataminingisoftenakeycomponentofinformationsystems,homelandsecuritysystems,andmonitoringandsurveillancesystems,itgivesawrongimpressionthatdataminingisatechniqueforprivacyintrusion.Thislackoftrusthasbecomeanobstacletothebenefitofthetechnology.Forexample,thepotentiallybeneficialdataminingre-searchproject,TerrorismInformationAwareness(TIA),wasterminatedbytheUSCongressduetoitscontroversialproceduresofcollecting,sharing,andanalyzingthetrailsleftbyindividuals.Motivatedbytheprivacyconcernsondataminingtools,aresearchareacalledprivacy-reservingdatamining(PPDM)emergedin2000.TheinitialideaofPPDMwastoextendtraditionaldataminingtechniquestoworkwiththedatamodifiedtomasksensitiveinformation.Thekeyissueswerehowtomodifythedataandhowtorecoverthe dataminingresultfromthemodifieddata.@#@Thesolutionswereoftentightlycoupledwiththedataminingalgorithmsunderconsideration.Incontrast,privacy-preservingdatapublishing(PPDP)maynotnecessarilytietoaspecificdataminingtask,andthedataminingtaskissometimesunknownatthetim