三Investment Tools Quantitative Methods.docx
《三Investment Tools Quantitative Methods.docx》由会员分享,可在线阅读,更多相关《三Investment Tools Quantitative Methods.docx(30页珍藏版)》请在冰豆网上搜索。
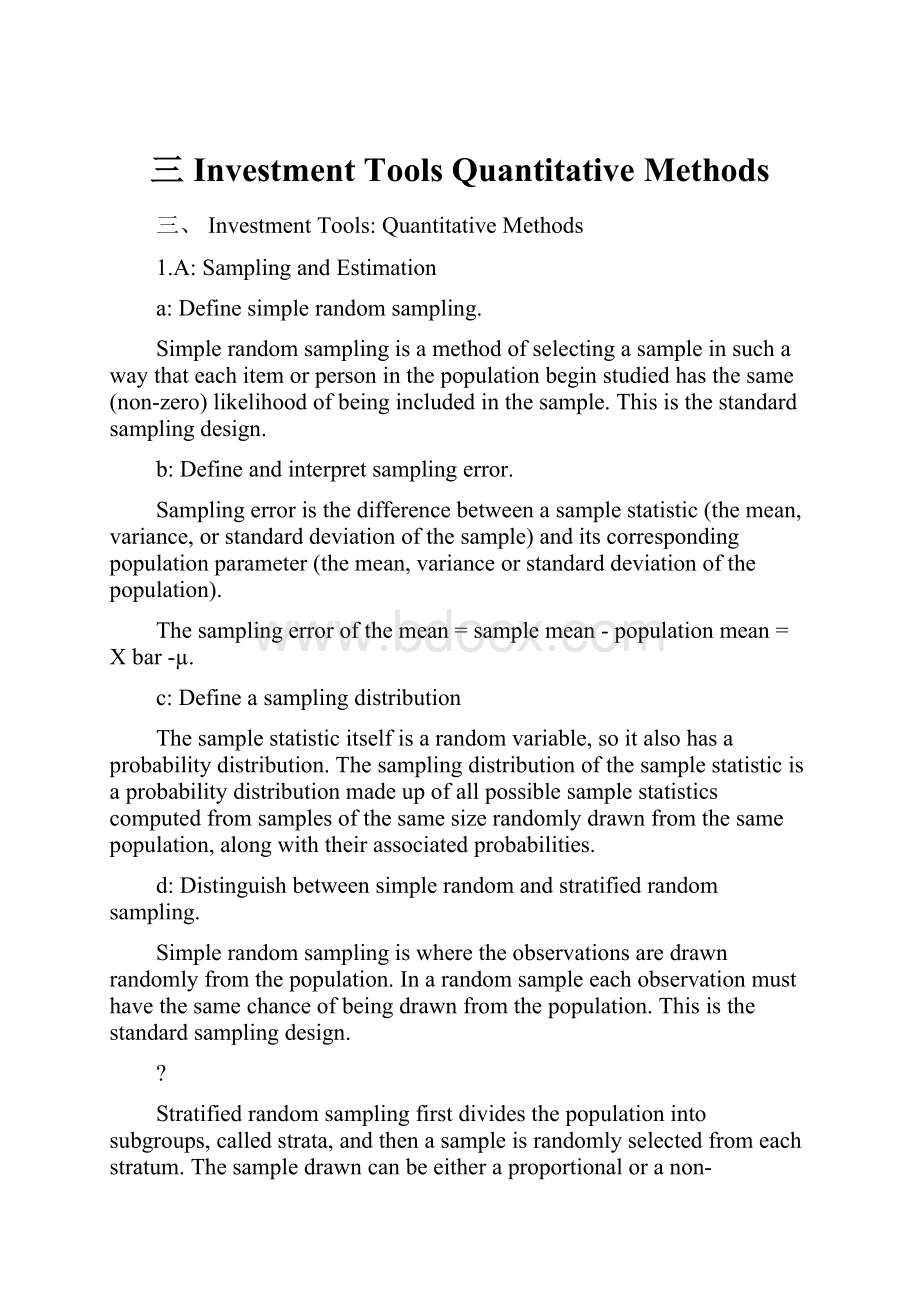
三InvestmentToolsQuantitativeMethods
三、InvestmentTools:
QuantitativeMethods
1.A:
SamplingandEstimation
a:
Definesimplerandomsampling.
Simplerandomsamplingisamethodofselectingasampleinsuchawaythateachitemorpersoninthepopulationbeginstudiedhasthesame(non-zero)likelihoodofbeingincludedinthesample.Thisisthestandardsamplingdesign.
b:
Defineandinterpretsamplingerror.
Samplingerroristhedifferencebetweenasamplestatistic(themean,variance,orstandarddeviationofthesample)anditscorrespondingpopulationparameter(themean,varianceorstandarddeviationofthepopulation).
Thesamplingerrorofthemean=samplemean-populationmean=Xbar-µ.
c:
Defineasamplingdistribution
Thesamplestatisticitselfisarandomvariable,soitalsohasaprobabilitydistribution.Thesamplingdistributionofthesamplestatisticisaprobabilitydistributionmadeupofallpossiblesamplestatisticscomputedfromsamplesofthesamesizerandomlydrawnfromthesamepopulation,alongwiththeirassociatedprobabilities.
d:
Distinguishbetweensimplerandomandstratifiedrandomsampling.
Simplerandomsamplingiswheretheobservationsaredrawnrandomlyfromthepopulation.Inarandomsampleeachobservationmusthavethesamechanceofbeingdrawnfromthepopulation.Thisisthestandardsamplingdesign.
?
Stratifiedrandomsamplingfirstdividesthepopulationintosubgroups,calledstrata,andthenasampleisrandomlyselectedfromeachstratum.Thesampledrawncanbeeitheraproportionaloranon-proportionalsample.Aproportionalsamplerequiresthatthenumberofitemsdrawnfromeachstratumbeinthesameproportionasthatfoundinthepopulation.
e:
Distinguishbetweentime-seriesandcross-sectionaldata.
Atime-seriesisasampleofobservationstakenataspecificandequallyspacedpointsintime.ThemonthlyreturnsonMicrosoftstockfromJanuary1990toJanuary2000areanexampleoftime-seriesdata.
Cross-sectionaldataisasampleofobservationstakenatasinglepointintime.ThesampleofreportedearningspershareofallNasdaqcompaniesasofDecember31,2000isanexampleofcross-sectionaldata.
f:
Statethecentrallimittheoremanddescribeitsimportance.
Thecentrallimittheoremtellsusthatforapopulationwithameanµandafinitevariance σ2,thesamplingdistributionofthesamplemeansofallpossiblesamplesofsizenwillbeapproximatelynormallydistributedwithameanequaltoµandavarianceequaltoσ2/n.
Thecentrallimittheoremisextremelyusefulbecausethenormaldistributionisrelativelyeasytoworkwithwhendoinghypothesistestingandformingconfidenceintervals.Wecanmakeveryspecificinferencesaboutthepopulationmean,usingthesamplemean,nomatterthedistributionofthepopulation,aslongasthesamplesizeis"large."
Whatyouneedtoknowfortheexam:
1.Ifthesamplesizenissufficientlylarge(greaterthan30),thesamplingdistributionofthesamplemeanswillbeapproximatelynormal.
2.Themeanofthepopulation,µ,andthemeanofallpossiblesamplemeans,µx,areequal.
3.Thevarianceofthedistributionofsamplemeansisσ2/n.
g:
Calculateandinterpretthestandarderrorofthesamplemean.
Standarderrorofthesamplemeansisthestandarddeviationofthesamplingdistributionofthesamplemeans.Thestandarderrorofthesamplemeanswhenthestandarddeviationofthepopulationisknowniscalculatedby:
σx=σ/√n,where:
σx=thestandarderrorofthesamplemeans,σ=thestandarddeviationofthepopulation,andn=thesizeofthesample.
Example:
ThemeanhourlywageforIowafarmworkersis$13.50withastandarddeviationof$2.90.LetxbethemeanwageperhourforarandomsampleofIowafarmworkers.Findthemeanandstandarderrorofthesamplemeans,x,forasamplesizeof30.
Themeanμxofthesamplingdistributionofxisμx=μ=$13.50.Sinceσisknown,thestandarderrorofthesamplemeansis:
σx=σ/√n=2.90/√30=$.53.Inconclusion,ifyouweretotakeallpossiblesamplesofsize30fromtheIowafarmworkerpopulationandprepareasamplingdistributionofthesamplemeansyouwillgetameanof$13.50andstandarderrorof$.53.
h:
Distinguishbetweenapointestimateandaconfidenceintervalestimateofapopulationparameter.
Pointestimatesaresingle(sample)valuesusedtoestimatepopulationparameters.Theformulaweusetocomputethepointestimateiscalledtheestimator.Forexample,thesamplemeanXbarisanestimatorofthepopulationmeanµ,andiscomputedusingthefollowingformula:
Xbar=(Σx/n)
Thevalueweobtainfromthiscalculationforaspecificsampleiscalledthepointestimateofthemean.
Aconfidenceintervalisarangeofestimatedvalueswithinwhichtheactualvalueoftheparameterwillliewithagivenprobabilityof1- α.Thetermαisalsocalledthesignificancelevelofthetest.Itisalsoknownastheconfidencelevel.
i:
Identifyanddescribethedesirablepropertiesofanestimate.
Whenwehaveachoiceamongseveralestimators,wewanttoselecttheonewiththemostdesirablestatisticalproperties:
unbiasedness,efficiency,andconsistency.
Anunbiasedestimatorisoneforwhichtheexpectedvalueoftheestimatorisequaltotheparameteryouaretryingtoestimate.
Anunbiasedestimatorisalsoefficientifthevarianceofitssamplingdistributionissmallerthanalltheotherunbiasedestimatorsoftheparameteryouaretryingtoestimate.Thesamplemean,forexample,isanefficientestimatorofthepopulationmean.
Aconsistentestimatorprovidedamoreaccurateestimateoftheparameterasthesamplesizeincreases.Asthesamplesizeincreases,thestandarddeviation(standarderror)ofthesamplemeanfallsandthesamplingdistributionbunchesmorecloselyaroundthepopulationmean.
j:
Calculateandinterpretaconfidenceintervalforapopulationmean,givenanormaldistributionwithaknownpopulationvariance.
Ifthedistributionofthepopulationisnormalandweknowthepopulationvariance,wecanconstructtheconfidenceintervalforthepopulationmeanasfollows:
?
XbarZα/2(σ/?
√n)
?
Example:
Supposeweadministerapracticeexamto100CFALevelIcandidates,andwediscoverthemeanscoreonthispracticeexamforall36ofthecandidatesinthesamplewhostudiedatleast10hoursaweekinpreparationfortheexamis80.Assumethepopulationstandarddeviationis15.Constructa99%confidenceintervalforthemeanscoreonthepracticeexamofcandidateswhostudyatleast10hoursaweek.
?
802.575(15/√36)=806.4
?
The99%confidencehasalowerlimitof73.6andanupperlimitof86.4.
k:
DescribethepropertiesofStudent'st-distribution.
Thestudent'st-distributionissimilar,butnotidenticaltothenormaldistributioninshape.Itisdefinedbyasingleparameter(thedegreesoffreedom),whereasthenormaldistributionisdefinedbytwoparameters(themeanandvariance).
Thestudent'st-distributionhasthefollowingproperties:
∙Itissymmetrical.
∙Itisdefinedbyasingleparameter,thedegreesoffreedom(df),wherethedegreesoffreedomareequaltothenumberofsampleobservationsminusone.(n-1).
∙Itislesspeakedthananormaldistribution,withmoreprobabilityinthetails.
∙Asthedegreesoffreedom(thesamplesize)getslarger,theshapeofthet-distributionapproachesastandardnormaldistribution.
l:
Calculateandinterpretaconfidenceintervalforapopulationmean,givenanormaldistributionwithanunknownpopulationvariance.
Example:
Supposeyoutakeasampleofthepast30monthlyreturnsforMcCrearyInc.Themeanreturnis2%,andthesamplestandarddeviationis20%.Thestandarderrorofthesamplewasfoundtobe3.6%.Constructa95%confidenceintervalforthemeanmonthlyreturn.
Becausethereare30observations,thedegreesoffreedomare30-1=29.Remember,becausethisisatwo-tailedtest,wewantthetotalprobabilityinthetailstobeα=5%;becausethet-distributionissymmetrical,theprobabilityineachtailwillbe2.5%whendf=29.Fromthet-table,wecandeterminethatthereliabilityfactorfortα/2,ort25,is2.045.Thentheconfidenceintervalis:
22.045(20/ √30)=2%7.4%
The95%confidenceintervalhasalowerlimitof-5.4%andanupperlimitof9.4%.
m:
Discusstheissuessurroundingselectionoftheappropriatesamplesize.
Whenthedistributionisnon-normal,thesizeofthesampleinfluenceswhetherornotwecanconstructtheappropriateconfidenceintervalforthesamplemean.Ifthedistributionisnon-normal,butthevarianceisknown,wecanstillusetheZ-statisticaslongasthesamplesizeislarge(n>30).Wecandothisbecausethecentrallimittheoremassuresusthatthedistributionofthesamplemeanisapproximatelynormalwhenthesampleislarge.
Ifthedistributionisnon-normalandthevarianceisunknown,wecanusethet-statisticaslongasthesamplesizeislarge(n>30).
Thismeansthatifwearesamplingfromanon-normaldistribution(whichissometimesthecaseinfinance),wecannotcreateaconfidenceintervalifthesamplesizeislessthan30.So,allelseequal,makesureyouhaveasamplelargerthan30,andthelarger,thebetter.
n:
Defineanddiscussdata-snooping/data-miningbias.
Data-snoopingbiasoccurswhentheresearcherbaseshisresearchonthepreviouslyreportedempiricalevidenceofothers,ratherthanonthetestablepredictionsofwell-developedeconomictheories.
Datasnoopingoftenleadstodatamining,whentheanalystcontinuallyusesthesamedatabasetosearchforpatternsortradingrulesuntilhefindsonethat"works."Forexample,someresearchersarguethatthevalueano