华南理工大学《模式识别》大作业报告.docx
《华南理工大学《模式识别》大作业报告.docx》由会员分享,可在线阅读,更多相关《华南理工大学《模式识别》大作业报告.docx(9页珍藏版)》请在冰豆网上搜索。
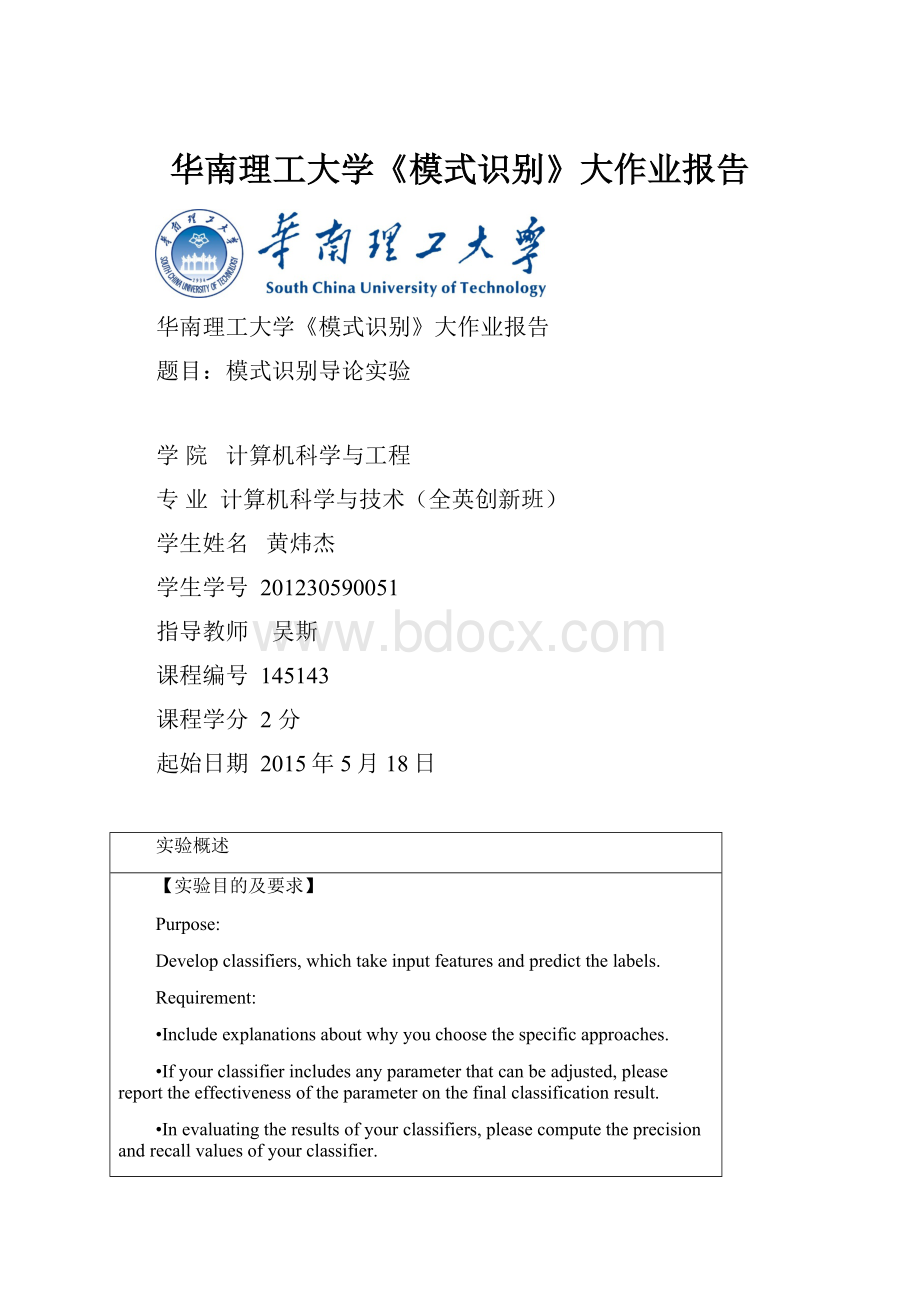
华南理工大学《模式识别》大作业报告
华南理工大学《模式识别》大作业报告
题目:
模式识别导论实验
学院计算机科学与工程
专业计算机科学与技术(全英创新班)
学生姓名黄炜杰
学生学号201230590051
指导教师吴斯
课程编号145143
课程学分2分
起始日期2015年5月18日
实验概述
【实验目的及要求】
Purpose:
Developclassifiers,whichtakeinputfeaturesandpredictthelabels.
Requirement:
•Includeexplanationsaboutwhyyouchoosethespecificapproaches.
•Ifyourclassifierincludesanyparameterthatcanbeadjusted,pleasereporttheeffectivenessoftheparameteronthefinalclassificationresult.
•Inevaluatingtheresultsofyourclassifiers,pleasecomputetheprecisionandrecallvaluesofyourclassifier.
•Partitionthedatasetinto2foldsandconductacross-validationprocedureinmeasuringtheperformance.
•Makesuretousefiguresandtablestosummarizeyourresultsandclarifyyourpresentation.
【实验环境】
Operatingsystem:
window8(64bit)
IDE:
MatlabR2012b
Programminglanguage:
Matlab
实验内容
【实验方案设计】
Mainstepsfortheprojectis:
1.Tomakeitmorechallenging,Iselectthelargerdataset,Pedestrian,ratherthanthesmallerone.Butitmaybenotwisetolearningonsuchalargedataset,soInormalizethedatasetfrom0to1firstandperformak-meanssamplingtoselectthemostrepresentativesamples.Afterthatfeatureselectionisdonesoastodecreasetheamountoffeatures.Atlast,aPCAdimensionreductionisusedtodecreasethesizeofthedataset.
2.SixlearningalgorithmsincludingK-NearestNeighbor,perception,decisiontree,supportvectormachine,multi-layerperceptionandNaïveBayesianareusedtolearnthepatternofthedataset.
3.Sixlearningalgorithmarecombingintosixmulti-classifierssystemindividually,usingbaggingalgorithm.
实验过程:
Theinputdatasetisnormalizedtotherangeof[0,1]sothatmakeitsuitableforperformingk-meansclusteringonit,andalsoincreasethespeedoflearningalgorithms.
Therearetoomuchsampleinthedataset,onlyasmallpartofthemareenoughtolearnagoodclassifier.Toselectthemostrepresentativesamples,k-meansclusteringisusedtoclusterthesampleintocgroupandselectr%ofthem.Thereare14596samplesinitially,but1460maybeenough,sor=10.Theselectionofcshouldfollowthreecriterions:
a)Lessdropofaccuracy
b)Littlechangeaboutratiooftwoclasses
c)Smallerc,lowertimecomplexity
SoIdesigntwoexperimentstofindthebestparameterc:
Experiment1:
Findoutthetrainingaccuracyofdifferentamountofcluster.Theresultisshowninthefigureontheleft.X-axisisamountofclusterandY-axisisaccuracy.Redlinedenotesaccuracybeforesamplingandbluelinedenotesaccuracyaftersampling.Asit’sshowninthefigure,c=2,5,7,9,13maybegoodchoicesincetheyhaverelativehigheraccuracy.
Experiment2:
Findouttheratioofsampleamountoftwoclass.Theresultisshowninthefigureontheright.X-axisisamountofclusterandY-axisistheratio.Redlinedenotesratiobeforesamplingandbluelinedenotesratioaftersampling.Asit’sshowninthefigure,c=2,5,9maybegoodchoicesincetheratiodonotchangesomuch.
Asaresult,c=5isselectedtosatisfythethreecriterions.
3780featuresismuchmorethanneededtotrainaclassifier,soIselectasmallpartofthembeforelearning.Thetargetistoselectmostdiscriminativefeatures,thatistosay,selectfeaturesthathavelargestaccuracyineachstep.Buttherearesixlearningalgorithminourproject,it’shardtodecidewhichlearningalgorithmthisfeatureselectionprocessshoulddependonanditmayalsohashightimecomplexity.Sorelevance,whichisthecorrelationbetweenfeatureandclassisusedasadiscriminationmeasurementtoselectthebestfeaturesets.Butonlyselectthemostrelevantfeaturesmayintroducerichredundancy.Soatradeoffbetweenrelevanceandredundancyshouldbemade.Anexperimentabouthowtomakethebesttradeoffisdone:
Experiment3:
Thisexperimentisafilterforwardfeatureselectionprocess.Thetargetistoselectthefeaturehasthemaximumvalueof(relevance+λ*redundancy)ineachstep,whererelevancedenotesthecorrelationbetweenfeatureandclass,andredundancydenotesmeanofpairwisefeaturecorrelation.λissetfrom-1to1.Theresultisshowntotheright:
X-axisdenotesnumberofselectedfeatures,Y-axisdenotesaccuracy.Eachlinesrepresentoneλ.It’sobviouslythatwithahigherλ,theaccuracyislower,thatistosay,withhigherredundancy,theperformanceoftheclassifierisworse.SoIselectλ=-1,andtheheuristicfunctionbecomes:
max(relevance-redundancy)
Theheuristicfunctionisknownnowbutthebestamountoffeaturesisstillunknownandisfoundinexperiment4:
Experiment4:
Findoutthetrainingaccuracyofdifferentamountoffeatures.Theresultisshownbelow.X-axisisamountoffeaturesandY-axisisaccuracy.Redlinedenotesaccuracybeforefeatureselectionandbluelinedenotesaccuracyafterfeatureselection.Asit’sshowninthefigure,whenfeatureamountreach50,theaccuracytrendtobestable.Soonly50featuresisselected.
Tomakethedatasetsmaller,featureswithcontributionrateofPCA≥85%isselected.Sowefinallyobtainadatasetwith1460samplesand32features.Thesizeofthedatasetdropsfor92.16%butaccuracyonlyhas0.61%decease.Sothesepreprocessingstepsaresuccessfultodecreasethesizeofthedataset.
6modelsareusedinthelearningsteps:
K-NearestNeighbor,perception,decisiontree,supportvectormachine,multi-layerperceptionandNaïveBayesian.IdesignedaRBFclassifierandMLPclassifieratfirstbuttheyaretooslowforthereasonthatmatrixmanipulationhasn’tbeendesignedcarefully,soIusethefunctioninthelibraryinstead.Parameterdeterminationfortheseclassifiersare:
1K-NN
Whenk≥5,theaccuracytrendstobestable,sok=5
2Decisiontree
Maxcritisusedasbinarysplittingcriterion.
3MLP
5unitsforhiddenisenough
Thesixlearningalgorithmcanbecombinginto6multi-classifierssystemindividuallytoincreasetheiraccuracy.Mostpopularmodelareboostingandbagging:
1Boosting
Eachclassifierisdependentonpreviousone,andhastheirownweight.Misclassifiedsampleshavehigherweight.Boostingalwaysoutperformbagging,butmaycausetheproblemofoverfitting.
2Bagging
Eachclassifierisindependentandallsamplearetreatedequally.Finalresultarevotebyeachclassifier.MoresuitableforunstableclassifiersuchasANN(littlechangeininputmaycauselargedifferenceinlearningresult).
IaminterestingaboutwillbaggingtrulyhelpincreasingaccuracyofunstableclassifiersuchasMLPanddecisiontree,andwhataboutstableclassifierlikeK-NN,NaïveBayesian,PerceptionandSVM.Thereisalsoaquestionthathowmanyclassifierisneed.Experiment5willshowtheanswer:
Experiment5:
Sixclassifiersisinvestigatedindividually,accuracyunderdifferentamountofclassifiersisshowninthefigurebelow.Eachfiguresstandsforacertainkindofclassifier.X-axisdenotesamountofclassifiersandY-axisdenotestheaccuracy.Blacklineisforthehighestaccuracyforthoseaccuracy,greenfortheworst,blueforthemeanofthemandredlineisforbaggingclassifiers.Wecanlearnfromthefigurethatbaggingdosehelpincreasingtheaccuracyofeachclassifiers,andforthedecisiontreeandMLP,baggingimprovetheaccuracyforagreatdegree.Whichisconsistentwiththeassumption.
实验结果
Conclusion:
Dimensionreductiontechniquesuchassampling,featureselectionandPCAarereallyhelpfultodecreasethesizeofdatasetandonlysacrificealittlebitofperformance.Butthepreconditionisthateveryparametershouldbedeterminecarefullytoguaranteegoodperformance.
Ensemblelearningisusefulforcombingweakclassifierstoobtainastrongclassifier.Evenforstrongclassifiers,ensemblelearningtechniquescanalsoimprovetheirperformance.
指导教师评语及成绩:
评语:
成绩:
指导教师签名:
批阅日期: