毕业论文外文翻译并条机自调匀整利用人工神经网络确定在自调匀整作用点外文原文+中文翻译.docx
《毕业论文外文翻译并条机自调匀整利用人工神经网络确定在自调匀整作用点外文原文+中文翻译.docx》由会员分享,可在线阅读,更多相关《毕业论文外文翻译并条机自调匀整利用人工神经网络确定在自调匀整作用点外文原文+中文翻译.docx(24页珍藏版)》请在冰豆网上搜索。
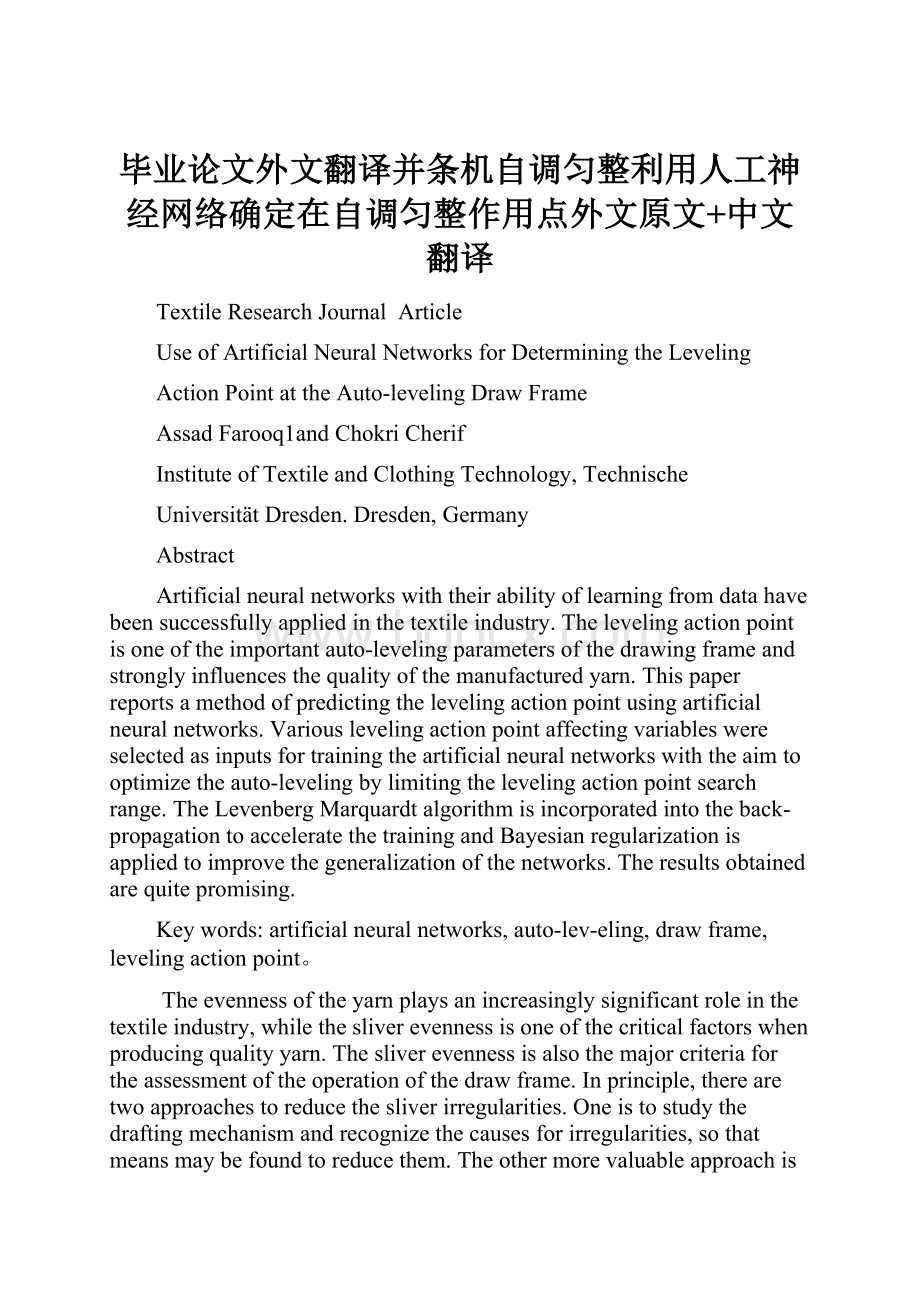
毕业论文外文翻译并条机自调匀整利用人工神经网络确定在自调匀整作用点外文原文+中文翻译
TextileResearchJournalArticle
UseofArtificialNeuralNetworksforDeterminingtheLeveling
ActionPointattheAuto-levelingDrawFrame
AssadFarooq1andChokriCherif
InstituteofTextileandClothingTechnology,Technische
UniversitätDresden.Dresden,Germany
Abstract
Artificialneuralnetworkswiththeirabilityoflearningfromdatahavebeensuccessfullyappliedinthetextileindustry.Thelevelingactionpointisoneoftheimportantauto-levelingparametersofthedrawingframeandstronglyinfluencesthequalityofthemanufacturedyarn.Thispaperreportsamethodofpredictingthelevelingactionpointusingartificialneuralnetworks.Variouslevelingactionpointaffectingvariableswereselectedasinputsfortrainingtheartificialneuralnetworkswiththeaimtooptimizetheauto-levelingbylimitingthelevelingactionpointsearchrange.TheLevenbergMarquardtalgorithmisincorporatedintotheback-propagationtoacceleratethetrainingandBayesianregularizationisappliedtoimprovethegeneralizationofthenetworks.Theresultsobtainedarequitepromising.
Keywords:
artificialneuralnetworks,auto-lev-eling,drawframe,levelingactionpoint。
Theevennessoftheyarnplaysanincreasinglysignificantroleinthetextileindustry,whilethesliverevennessisoneofthecriticalfactorswhenproducingqualityyarn.Thesliverevennessisalsothemajorcriteriafortheassessmentoftheoperationofthedrawframe.Inprinciple,therearetwoapproachestoreducethesliverirregularities.Oneistostudythedraftingmechanismandrecognizethecausesforirregularities,sothatmeansmaybefoundtoreducethem.Theothermorevaluableapproachistouseauto-levelers[1],sinceinmostcasesthedoublingisinadequatetocorrectthevariationsinsliver.Thecontrolofsliverirregularitiescanlowerthedependenceoncardsliveruniformity,ambientconditions,andframeparameters.
Attheauto-levelerdrawframe(RSB-D40)thethicknessvariationsinthefedsliverarecontinuallymonitoredbyamechanicaldevice(atongue-grooveroll)andsubsequentlyconvertedintoelectricalsignals.Themeasuredvaluesaretransmittedtoanelectronicmemorywithavariable,thetimedelayedresponse.Thetimedelayallowsthedraftbetweenthemid-rollandthedeliveryrollofthedrawframetoadjustexactlyatthatmomentwhenthedefectivesliverpiece,whichhadbeenmeasuredbyapairofscanningrollers,findsitselfatapointofdraft.Atthispoint,aservomotoroperatesdependingupontheamountofvariationdetectedinthesliverpiece.Thedistancethatseparatesthescanningrollerspairandthepointofdraftiscalledthezeropointofregulationorthelevelingactionpoint(LAP)asshowninFigure1.Thisleadstothecalculatedcorrectiononthecorrespondingdefectivematerial[2,3].Inauto-levelingdrawframes,especiallyinthecaseofachangeoffibermaterial,orbatchesthemachinesettingsandprocesscontrollingparametersmustbeoptimized.TheLAPisthemostimportantauto-levelingparameterwhichisinfluencedbyvariousparameterssuchasfeedingspeed,material,breakdraftgauge,maindraftgauge,feedingtension,breakdraft,andsettingofthesliverguidingrollersetc.
UseofArtificialNeuralNetworksforDeterminingtheLevelingActionPointA.FarooqandC.Cherif
Figure1Schematicdiagramofanauto-levelerdrawingframe.
Previously,thesliversampleshadtobeproducedwithdifferentsettings,takentothelaboratory,andexaminedontheevennesstesteruntiltheoptimumLAPwasfound(manualsearch).Auto-levelerdrawframeRSB-D40implementsanautomaticsearchfunctionfortheoptimumdeterminationoftheLAP.Duringthisfunction,thesliverisautomaticallyscannedbyadjustingthedifferentLAPstemporarilyandtheresultedvaluesarerecorded.Duringthisprocess,thequalityparametersareconstantlymonitoredandanalgorithmautomaticallycalculatestheoptimumLAPbyselectingthepointwiththeminimumsliverCV%.Atpresentasearchrangeof120mmisscanned,i.e.21pointsareexaminedusing100mofsliverineachcase;therefore2100mofsliverisnecessarytocarryoutthesearchfunction.Thisisaverytime-consumingmethodaccompaniedbythematerialandproductionlosses,andhencedirectlyaffectingthecostparameters.Inthiswork,wehavetriedtofindoutthepossibilityofpredictingtheLAP,usingartificialneuralnet-works,tolimittheautomaticsearchspanandtoreducetheabove-mentioneddisadvantages.
ArtificialNeuralNetworks
Themotivationofusingartificialneuralnetworksliesintheirflexibilityandpowerofinformationprocessingthatconventionalcomputingmethodsdonothave.Theneuralnetworksystemcansolveaproblem“byexperienceandlearning”theinput–outputpatternsprovidedbytheuser.Inthefieldoftextiles,artificialneuralnetworks(mostlyusingback-propagation)havebeenextensivelystudiedduringthelasttwodecades[4–6].Inthefieldofspinningpreviousresearchhasconcentratedonpredictingtheyarnpropertiesandthespinningprocessperformanceusingthefiberpropertiesoracombinationoffiberpropertiesandmachinesettingsastheinputofneuralnetworks[7–12].Back-propagationisasupervisedlearningtechniquemostfrequentlyusedforartificialneuralnetworktraining.Theback-propagationalgorithmisbasedontheWidrow-Hoffdeltalearningruleinwhichtheweightadjustmentiscarriedoutthroughthemeansquareerroroftheoutputresponsetothesampleinput[13].Thesetofthesesamplepatternsisrepeatedlypresentedtothenetworkuntiltheerrorvalueisminimized.Theback-propagationalgorithmusesthesteepestdescentmethod,whichisessentiallyafirst-ordermethodtodetermineasuitabledirectionofgradientmovement.
Overfitting
Thegoalofneuralnetworktrainingistoproduceanetworkwhichproducessmallerrorsonthetrainingset,andwhichalsorespondsproperlytonovelinputs.Whenanetworkperformsaswellonnovelinputsasontrainingsetinputs,thenetworkissaidtobewellgeneralized.Thegeneralizationcapacityofthenetworkislargelygovernedbythenetworkarchitecture(numberofhiddenneurons)andthisplaysavitalroleduringthetraining.Anetworkwhichisnotcomplexenoughtolearnalltheinformationinthedataissaidtobeunderfitted,whileanetworkthatistoocomplextofitthe“noise”inthedataleadstooverfitting.“Noise”meansvariationinthetargetvaluesthatareunpredictablefromtheinputsofaspecificnetwork.Allstandardneuralnetworkarchitecturessuchasthefullyconnectedmulti-layerperceptronarepronetooverfitting.Moreover,itisverydifficulttoacquirethenoisefreedatafromthespinningindustryduetothedependenceofendproductsontheinherentmaterialvariationsandenvironmentalconditions,etc.Earlystoppingisthemostcommonlyusedtechniquetotacklethisproblem.Thisinvolvesthedivisionoftrainingdataintothreesets,i.e.atrainingset,avalidationsetandatestset,withthedrawbackthatalargepartofthedata(validationset)canneverbethepartofthetraining.
Regularization
Theothersolutionofoverfittingisregularization,whichisthemethodofimprovingthegeneralizationbyconstrainingthesizeofthenetworkweights.Mackay[14]discussedapracticalBayesianframeworkforback-propagationnetworks,whichconsistentlyproducednetworkswithgoodgeneralization.
Theinitialobjectiveofthetrainingprocessistomini-mizethesumofsquareerrors:
(1)
Where
arethetargetsand
aretheneuralnetworkresponsestotherespectivetargets.Typically,trainingaimstoreducethesumofsquarederrorsF=Ed.However,regularizationaddsanadditionalterm,theobjectivefunction,
(2)
Inequation
(2),
isthesumofsquaresofthenetworkweights,andαandβareobjectivefunctionparameters.Therelativesizeoftheobjectivefunctionparametersdictatestheemphasisfortraining.Ifα<<β,thenthetrainingalgorithmwilldrivetheerrorssmaller.Ifα>>β,trainingwillemphasizeweightsizereductionattheexpenseofnetworkerrors,thusproducingasmoothernetworkresponse[15].
TheBayesianSchoolofstatisticsisbasedonadifferentviewofwhatitmeanstolearnfromdata,inwhichprobabilityisusedtorepresenttheuncertaintyabouttherelationshipbeinglearned.Beforeseeinganydata,theprioropinionsaboutwhatthetruerelationshipmightbecanbeexpressedinaprobabilitydistributionoverthenetworkweightsthatdefinethisrelationship.Aftertheprogramconceivesthedata,therevisedopinionsarecapturedbyaposteriordistributionovernetworkweights.Networkweightsthatseemedplausiblebefore,butwhichdonotmatchthedataverywell,willnowbeseenasbeingmuchlesslikely,while
theprobabilityforvaluesoftheweightsthatdofitthedatawellwillhaveincreased[16].
IntheBayesianframeworktheweightsofthenetworkareconsideredrandomvariables.Afterthedataistaken,theposteriorprobabilityfunctionfortheweightscanbeupdatedaccordingtoBayes’rule:
(3)
Inequation(3),Drepresentsthedataset,Mistheparticularneuralnetworkmodelused,andwisthevectorofnetworkweights.
isthepriorprobability,whichrepresentsourknowledgeoftheweightsbeforeanydataiscollected.
isthelikelihoodfunction,whichistheprobabilityofdataoccurring,giventheweightsw.
isanormalizationfactor,whichguaranteesthatthetotalprobabilityis1[15].
Inthisstudy,weemployedtheMATLABNeuralNet-worksToolboxfunction“trainbr”whichisanincorporationoftheLevenberg–MarqaurdtalgorithmandtheBayesianregularizationtheorem(orBayesianlearning)intoback-