基于微博的社交网络影响力模型化方法研究论文.docx
《基于微博的社交网络影响力模型化方法研究论文.docx》由会员分享,可在线阅读,更多相关《基于微博的社交网络影响力模型化方法研究论文.docx(78页珍藏版)》请在冰豆网上搜索。
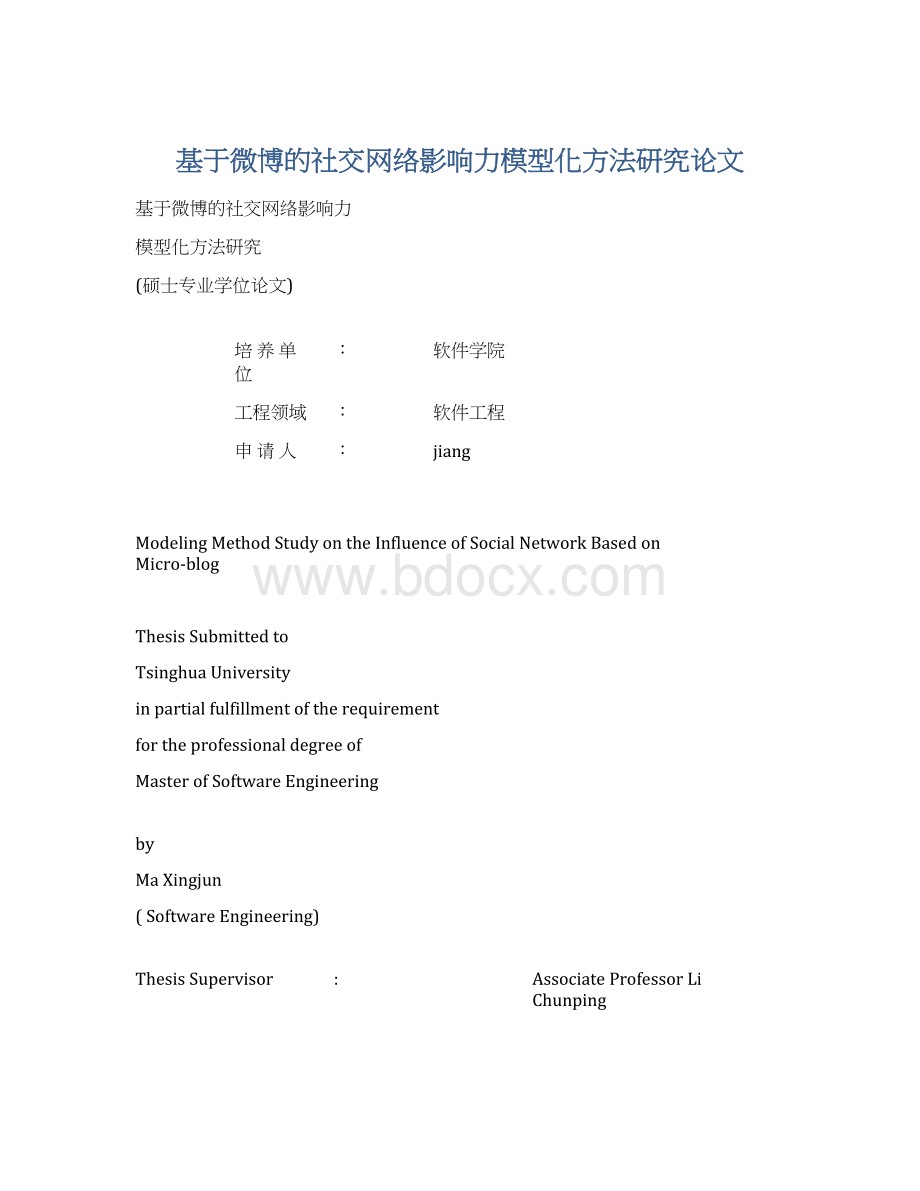
基于微博的社交网络影响力模型化方法研究论文
基于微博的社交网络影响力
模型化方法研究
(硕士专业学位论文)
培养单位
:
软件学院
工程领域
:
软件工程
申请人
:
jiang
ModelingMethodStudyontheInfluenceofSocialNetworkBasedonMicro-blog
ThesisSubmittedto
TsinghuaUniversity
inpartialfulfillmentoftherequirement
fortheprofessionaldegreeof
MasterofSoftwareEngineering
by
MaXingjun
(SoftwareEngineering)
ThesisSupervisor
:
AssociateProfessorLiChunping
November,2014
摘要
随着Twitter的流行和海量数据的堆积,如何从这些数据中进行合理的筛选并从中挖掘出有意义的内容已经成为当今的研究热点。
虽然数据挖掘的目的各不相同但其中特征的选择和模型的构建是影响研究的关键因素。
本文的研究基于微博平台Twitter展开。
本文对用户的时间特征,也就是活跃度随时间的变化规律进行了详细的研究并结合此类特征对现有影响力模型进行了改进。
首先,我们对Twitter用户的时间特征进行了统计分析并基于K-SC聚类算法对用户的时间模式进行了聚类。
统计分析和聚类的结果显示Twitter用户的时间模式可以划分为6种不同的类型。
同时我们发现用户总体活跃的高峰期是在周一、二的12到23点以及周六、日的20到23点。
另外,我们在对用户的延迟指标的研究中发现:
72%的转发是在1小时之内完成的,而相应回复所占的比例是83%。
这些结论不仅详细的展现了用户的时间特征也说明了Twitter消息的时效性。
在随后的实验中本文挖掘了影响信息传播的关键特征。
结合社交特征、时间特征和话题特征,本文构建了新的特征空间并基于响应(统指转发和回复)预测模型研究了不同特征对消息传播的影响。
基于新特征空间的GBDT预测模型的预测准确率达到了80.55%,同时我们发现接收者是否对Tweet作出响应取决于三个关键因素:
接收者和发布者之间的话题相似度、发布者被分组的次数和接收者在Tweet发布时的活跃度。
最后,基于在响应预测分析中得到的结论和PageRank算法的思想,本文提出了一种新的影响力排行模型:
TTR(Temporal-TopicRank)。
在实验评估部分我们验证了TTR在三种不同的影响力排行任务中的表现比TR(TwitterRank)和PR(PageRank)更加优秀、更加灵活。
在全局影响力排行任务中TTR不但能够挖掘影响力大的用户而且能够通过调节惩罚因子C的大小从影响力大的用户中重点突出那些影响信息传播的关键用户。
在好友推荐任务中TTR算法在7种场景下的6种场景中的表现优于TR算法,同时在6种场景下优于PR算法,其中在TR表现最差的两种场景下TTR算法可以通过调节惩罚因子的大小大幅提高推荐效果。
另外,在挖掘最大影响力好友的任务中TTR的结果比TR和PR更接近实际情况。
关键词:
时间特征响应预测影响力排行社交网络
Abstract
AlongwiththepopularityofTwitter,moreandmorepeoplebecomeactive,andtheaccumulation ofmassivedata,howtoextractproperfeaturesselectivelyfromthosedataandtomakeuseofthemtominevaluableinformationhavebecamehottopicsalready.Althoughfocusingondifferenttargets,featureselectionandmodelingmethodarethetwocriticalfactorsthataffecttoday’sresearches.
TwitterFeaturescouldbedividedintofourdimensions:
social,topic,spacialandtemporaldimension,amongwhichthetemporalfeatureconsistsofusertemporalfeatureandtweettemporalfeature.Usertemporalfeaturereferstovariationpatternsofuserbehaviorsuchaspost,retweetandreply,whiletweettemporalfeaturemeanssimilarvariationpatterns,howeveroftweet.Thisarticlestudiesuser-temporal-featurecomprehensivelyandfurtherinvestigatesitsinfluenceonexistingmodels.
Inthefirstplace,usinganalyticalmethodsof Statistics,wecalculatedthevariationpatternsofTwitterusers’activedegree,andthenclusteredthosepatternsintosixcommonclassesbasedonK-SCclusteringalgorithm.Experimentresultindicatesthattherearesixuniquepatterns,andeachofthemrepresentsonetypeofuser’sactivityvariation.ItalsoshowsthatthepeakperiodsofTwitteruser’spatterncurvearetwelvetotwentythreeo'clockonMondayandTuesday,andtwentytotwentythreeo'clockattheWeekend.Meanwhile,bycomputingdelayandlook-backdistributionofalltheresponses,wefindsthat72percentoftheretweetaretakenplacewithinonehourandthepercentofreplyis83.Actually,80%oftheretweetareretweetedbylookingbacknomorethan100historicaltweetsandthatofreplyis85%.Theseinterestingfindingsnotonlydisplayusers’readinghabitbutalsoindicatethattweetsdohavetimevalidity.
Furthermore,weinvestigatedtheinfluencethatuser-temporal-featurehasonresponsepredictionmodel.Basedonnewfeaturespace,ournewmodelcouldpredictwhetherauserwouldretweetorreplyoneofhis/herfriends’tweetwithanaccuracyof80.55%.ComparedwithPetrovic’smodel,thenewmodelachievesa
score(BalancedF-score)of86.6,whichbettersPetrovic’sof46.6.Besidesoftheaccuracyandperformance,werankedallthefeaturesaccordingtotheircontributionstothemodel.Rankingresultshowsthatthetop3featuresareasfollows:
thetopicsimilaritybetweenthefriendandthefollower,thelistednumberofthefriendandthetimefeatureofthefollower.
Inthethirdpartofourresearchweincorporateusertimefeatureintooneofcurrentinfluencerankingmodels:
TR(TwitterRank).ThenewmodelwethengetisdenotedasTTR(Temporal-TopicRank).Newrankingresultsubstantiatestheassumptionthatusertimefeaturewouldhavegreateffectsonexistingmodels:
thecoefficientdegreeofTTRandTRisjust0.7627.Albeitdifferentrankingmodelsmayservedifferentrankingtaskswell,thediscrepancybetweenTRandTTRcouldatleastdemonstratesthatusertimefeaturedoeshaveacertainamountofinfluenceonTR.
Keywords:
TemporalPatternResponsePredictionInfluenceRankingSocialNetwork
第1章引言
1.1课题背景和意义
近年来,随着互联网行业的蓬勃发展出现了很多大型的社交网络,如Twitter、Facebook等,这些社交平台对人们的生活产生越来越大的影响。
在这些大型社交网络中Twitter是一个典型的代表,它允许用户不经过其他用户的同意而关注他们。
这种特征吸引了越来越多的用户,他们在Twitter中阅读、发布、转发和回复各种信息,这使得Twitter更像是一个新闻媒体[1]。
据Twitter官方发布的统计数据,截止到2013年10月Twitter活跃用户超过2亿,每天发布Tweet超过4亿条。
大量的活跃用户和快速的信息传播使得Twitter在很多事件中都产生了巨大的影响力,如2008年美国